The Standard Deviation Of The Distribution Of Sample Means Is
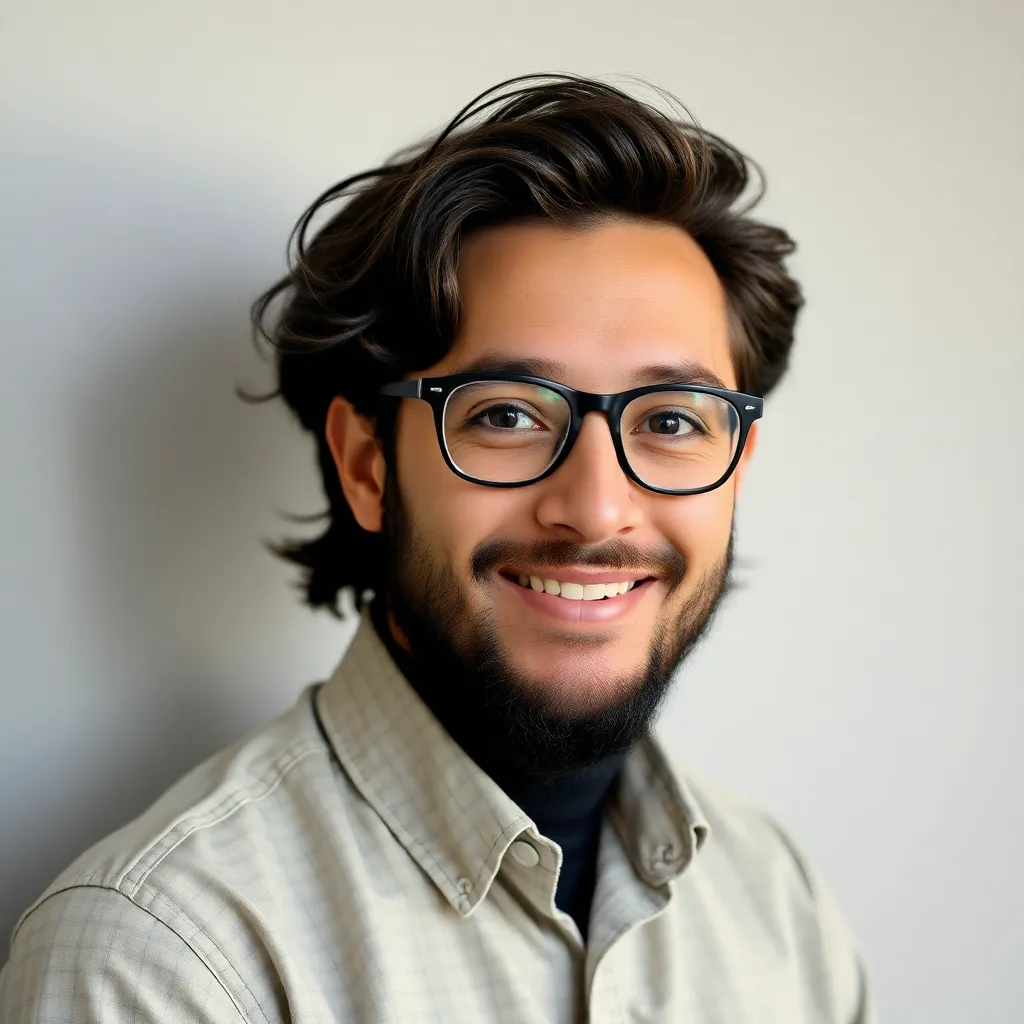
Muz Play
May 09, 2025 · 6 min read
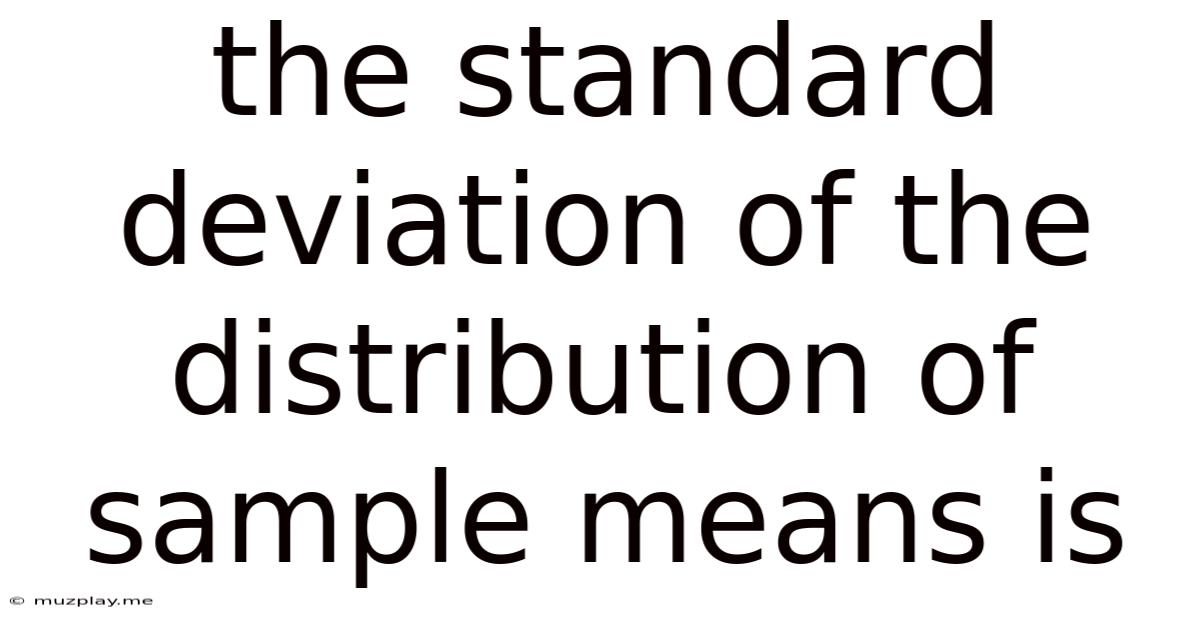
Table of Contents
The Standard Deviation of the Distribution of Sample Means: Understanding Standard Error
The standard deviation of the distribution of sample means, also known as the standard error of the mean (SEM), is a crucial concept in statistics. It quantifies the variability you'd expect to see in sample means if you were to repeatedly sample from a population. Understanding the SEM is vital for interpreting statistical results, constructing confidence intervals, and performing hypothesis tests. This article will delve deep into the SEM, explaining its calculation, interpretation, and practical applications.
What is the Standard Error of the Mean (SEM)?
The SEM isn't a measure of the variability within a single sample; instead, it describes the variability between sample means drawn from the same population. Imagine taking numerous random samples from a population, calculating the mean of each sample, and then plotting those means on a distribution. This distribution of sample means will have its own standard deviation—that's the SEM. A smaller SEM indicates that the sample means are clustered tightly around the true population mean, suggesting that the sample means are reliable estimators of the population mean. Conversely, a larger SEM suggests more variability between sample means, indicating less precision in estimating the population mean.
Why is the SEM smaller than the population standard deviation?
Intuitively, you might expect the SEM to be equal to the population standard deviation. However, this isn't the case. The SEM is always smaller than the population standard deviation (σ). This is due to the Central Limit Theorem (CLT). The CLT states that the distribution of sample means will approximate a normal distribution, regardless of the shape of the population distribution, as the sample size (n) increases. Furthermore, the mean of this sampling distribution will be equal to the population mean (μ), and its standard deviation (the SEM) will be equal to σ/√n. The division by the square root of the sample size is key; as the sample size increases, the SEM decreases. This reflects the fact that larger samples provide more accurate estimates of the population mean.
Calculating the Standard Error of the Mean
The formula for calculating the SEM is straightforward:
SEM = σ / √n
Where:
- SEM is the standard error of the mean.
- σ is the population standard deviation.
- n is the sample size.
When the Population Standard Deviation is Unknown
In most real-world scenarios, the population standard deviation (σ) is unknown. In such cases, we estimate the SEM using the sample standard deviation (s) as a substitute:
SEM ≈ s / √n
Where:
- s is the sample standard deviation.
This approximation becomes increasingly accurate as the sample size increases. It's important to remember that using the sample standard deviation introduces some uncertainty into the SEM estimate.
Interpreting the Standard Error of the Mean
The SEM is interpreted in the context of the sampling distribution. It provides a measure of the precision of the sample mean as an estimator of the population mean. A smaller SEM implies a more precise estimate, while a larger SEM suggests a less precise estimate. This precision is directly related to the sample size: larger samples yield smaller SEMs and more precise estimates.
Confidence Intervals and the SEM
The SEM plays a crucial role in constructing confidence intervals. A confidence interval provides a range of values within which we are confident the true population mean lies. A common confidence level is 95%, meaning that if we were to repeatedly sample from the population and construct 95% confidence intervals, 95% of those intervals would contain the true population mean. The formula for a 95% confidence interval is:
Confidence Interval = Sample Mean ± (1.96 * SEM)
The 1.96 value is derived from the standard normal distribution and corresponds to a 95% confidence level. For other confidence levels, a different multiplier would be used (e.g., 2.58 for a 99% confidence interval). The width of the confidence interval is directly proportional to the SEM: a smaller SEM results in a narrower, more precise confidence interval.
Factors Affecting the Standard Error of the Mean
Several factors influence the magnitude of the SEM:
-
Population Standard Deviation (σ): A larger population standard deviation leads to a larger SEM, indicating greater variability in the sample means. This reflects the inherent variability within the population itself.
-
Sample Size (n): As mentioned earlier, the sample size has an inverse relationship with the SEM. Larger sample sizes result in smaller SEMs and more precise estimates of the population mean. This is because larger samples provide more information about the population.
Applications of the Standard Error of the Mean
The SEM has numerous applications across various fields:
-
Hypothesis Testing: The SEM is fundamental in hypothesis testing. It's used to calculate test statistics like the t-statistic, which helps determine whether there's a statistically significant difference between two groups or between a sample mean and a hypothesized population mean.
-
Meta-Analysis: In meta-analysis, where results from multiple studies are combined, the SEM is used to weight the individual studies based on their precision. Studies with smaller SEMs (indicating greater precision) are given more weight in the overall analysis.
-
Process Control: In quality control, the SEM can be used to monitor the stability of a process. Changes in the SEM over time might indicate shifts in the process mean or increased variability.
Distinguishing SEM from Standard Deviation
It is crucial to understand the difference between the standard deviation and the standard error. The standard deviation (SD) measures the dispersion or spread of individual data points within a single sample. In contrast, the standard error of the mean (SEM) measures the dispersion or spread of the means of multiple samples drawn from the same population. The SEM reflects the precision of the sample mean as an estimate of the population mean. The SD is descriptive of a single sample, while the SEM is inferential, used to make inferences about the population.
Practical Example: Calculating and Interpreting SEM
Let's consider a simple example. Suppose we want to estimate the average height of adult women in a city. We collect a random sample of 100 women and find that the sample mean height is 165 cm, and the sample standard deviation is 5 cm.
To calculate the SEM:
SEM ≈ s / √n = 5 cm / √100 = 0.5 cm
This means that the standard error of the mean is 0.5 cm. This indicates that if we were to repeatedly sample 100 women from this city, the means of those samples would vary, on average, by 0.5 cm from the true population mean.
A 95% confidence interval for the average height of women in the city would be:
Confidence Interval = 165 cm ± (1.96 * 0.5 cm) = 165 cm ± 0.98 cm = (164.02 cm, 165.98 cm)
We are 95% confident that the true average height of women in the city lies between 164.02 cm and 165.98 cm.
Conclusion: The Importance of Standard Error
The standard error of the mean is an essential statistical concept for understanding the precision and reliability of sample means as estimates of population means. It directly informs confidence intervals and hypothesis tests, allowing researchers to make valid inferences about populations based on sample data. Understanding the SEM is crucial for anyone working with statistical data, from researchers and scientists to data analysts and decision-makers. The factors affecting the SEM – specifically the population standard deviation and sample size – highlight the importance of careful experimental design and the collection of sufficiently large and representative samples to ensure precise estimates. By understanding and correctly applying the SEM, we can greatly enhance the accuracy and reliability of our statistical conclusions.
Latest Posts
Latest Posts
-
Does Transcription And Translation Occur Simultaneously In Prokaryotes
May 09, 2025
-
Light Dependent Reaction Reactants And Products
May 09, 2025
-
Is Lithium Metal Nonmetal Or Metalloid
May 09, 2025
-
Terminal Electron Acceptor In Aerobic Respiration
May 09, 2025
-
Similarities Between Nuclear Fission And Nuclear Fusion
May 09, 2025
Related Post
Thank you for visiting our website which covers about The Standard Deviation Of The Distribution Of Sample Means Is . We hope the information provided has been useful to you. Feel free to contact us if you have any questions or need further assistance. See you next time and don't miss to bookmark.