Upper Limit And Lower Limit Formula Statistics
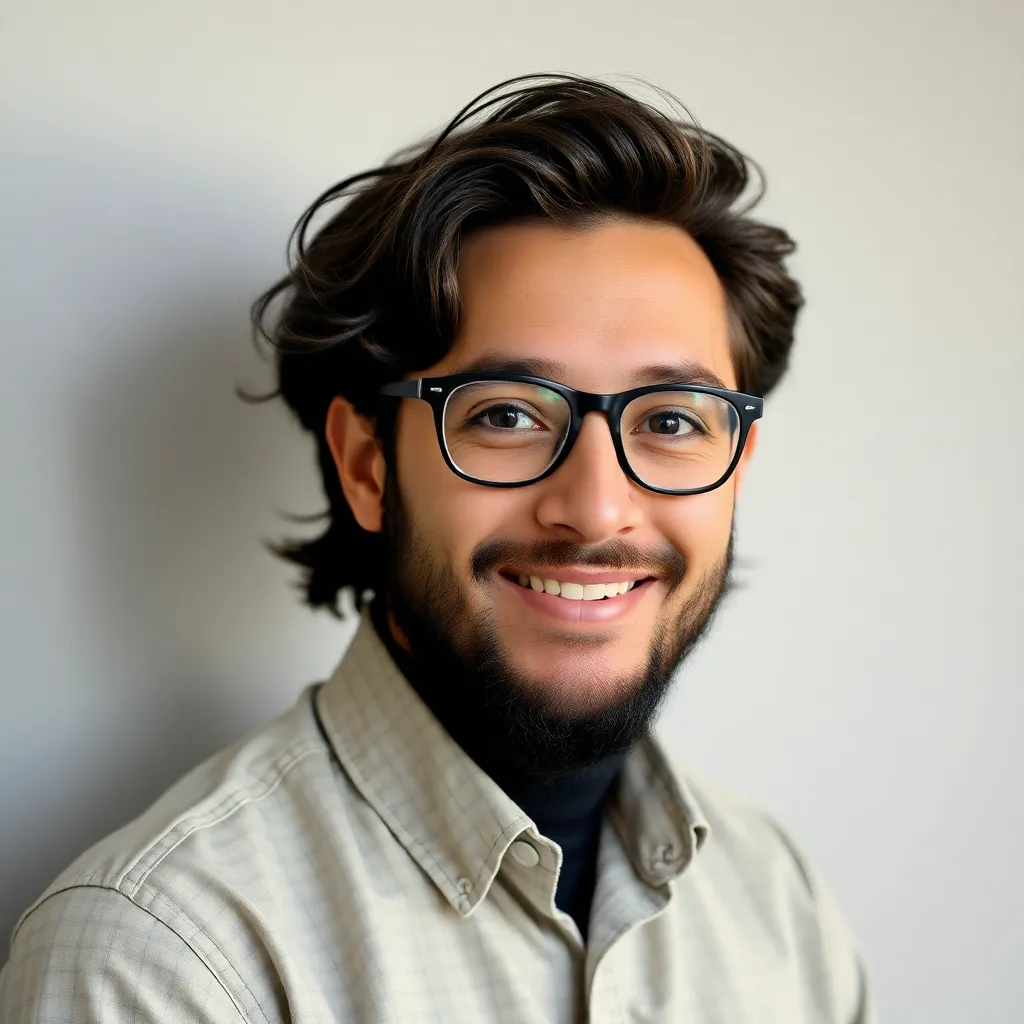
Muz Play
May 10, 2025 · 5 min read
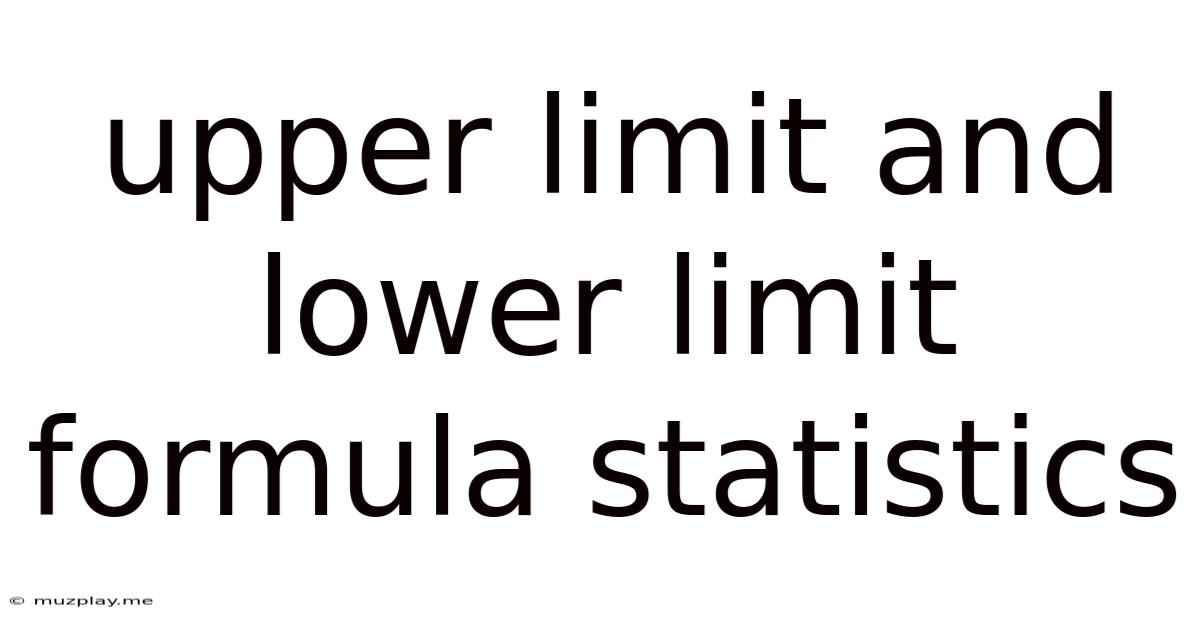
Table of Contents
Understanding Upper and Lower Limits in Statistics: A Comprehensive Guide
Understanding upper and lower limits is crucial in statistics, particularly when dealing with descriptive statistics, confidence intervals, and control charts. These limits define the boundaries within which a certain percentage of data points are expected to fall. This comprehensive guide will delve into the formulas, applications, and interpretations of upper and lower limits in various statistical contexts.
What are Upper and Lower Limits?
Upper and lower limits define the range within which a variable is expected to lie. They are not arbitrary boundaries; instead, their calculation is based on statistical principles, using data characteristics like the mean and standard deviation. The specific formulas vary depending on the context, but the core concept remains consistent: to define a range that encompasses a predetermined portion of the data.
Importance of Upper and Lower Limits
Understanding and correctly applying upper and lower limits is vital for several reasons:
- Data Interpretation: They help us understand the spread and variability of data. Outliers, values falling outside these limits, can be identified and investigated for potential errors or anomalies.
- Process Control: In quality control, upper and lower control limits are crucial for monitoring processes and identifying deviations that signal potential problems.
- Confidence Intervals: In inferential statistics, upper and lower limits form the confidence intervals, providing a range within which a population parameter (e.g., mean) is likely to lie.
- Prediction: Limits can provide a range of plausible values for future observations or predictions.
Formulas for Upper and Lower Limits: Various Contexts
The formulas for calculating upper and lower limits change depending on the specific statistical context. Here are some of the most common scenarios:
1. Confidence Intervals for the Population Mean
This is arguably the most common application of upper and lower limits. When estimating the population mean from a sample, we construct a confidence interval. The formula is:
Upper Limit = Sample Mean + (Critical Value * Standard Error)
Lower Limit = Sample Mean - (Critical Value * Standard Error)
Where:
- Sample Mean (x̄): The average of the sample data.
- Critical Value: This depends on the desired confidence level (e.g., 95%, 99%) and the degrees of freedom (n-1 for a t-distribution, often used for smaller sample sizes). It's obtained from a t-table or using statistical software. For large samples (generally n>30), the Z-score from a standard normal distribution can be used as an approximation.
- Standard Error (SE): The standard deviation of the sampling distribution of the mean. It's calculated as: SE = Sample Standard Deviation / √(Sample Size)
Example: Suppose we have a sample of 50 observations with a mean of 100 and a standard deviation of 15. For a 95% confidence interval, the critical value (t or Z) is approximately 1.96.
- SE = 15 / √50 ≈ 2.12
- Upper Limit = 100 + (1.96 * 2.12) ≈ 104.16
- Lower Limit = 100 - (1.96 * 2.12) ≈ 95.84
Therefore, we are 95% confident that the true population mean lies between 95.84 and 104.16.
2. Control Charts (Process Capability Analysis)
Control charts are used in quality control to monitor a process over time. They typically have upper and lower control limits (UCL and LCL) that define the acceptable range of variation. Common control charts include:
- X-bar and R charts: Monitor the average (X-bar) and range (R) of a process.
- p-charts: Monitor the proportion of defective items.
- c-charts: Monitor the number of defects per unit.
The formulas for UCL and LCL vary depending on the type of control chart. They generally involve the process mean and standard deviation (or estimates thereof) and factors derived from statistical tables (e.g., A2, D3, D4 factors for X-bar and R charts). These factors account for the variability inherent in the sampling process.
Example (X-bar chart):
UCL = X̄ + A2 * R̄
LCL = X̄ - A2 * R̄
Where:
- X̄ is the average of the sample means.
- R̄ is the average of the sample ranges.
- A2 is a constant obtained from a control chart table.
3. Tolerance Intervals
Tolerance intervals provide a range that contains a specified proportion of the population with a certain confidence level. They differ from confidence intervals, which focus on estimating a population parameter. Tolerance intervals provide a range encompassing a specific percentage of the data itself. Formulas for tolerance intervals are more complex and often require specialized statistical software.
4. Prediction Intervals
Prediction intervals provide a range within which a future observation is expected to fall, given a certain confidence level. They account for both the uncertainty in estimating the population mean and the inherent variability within the population. The formula incorporates the standard error of the mean and an additional term representing the variability of individual observations.
Interpreting Upper and Lower Limits
The interpretation of upper and lower limits depends entirely on their context.
- Confidence Intervals: They provide a range of plausible values for a population parameter. A wider interval indicates greater uncertainty.
- Control Charts: Points consistently falling outside the UCL or LCL suggest that the process is out of control and requires investigation.
- Tolerance Intervals: These show the range within which a specific percentage of the population is expected to lie.
- Prediction Intervals: These define a range where a single future observation is likely to be found.
Identifying and Dealing with Outliers
Values falling outside the calculated upper and lower limits are often considered outliers. They require careful consideration:
- Investigate the Cause: Are they due to measurement error, data entry mistakes, or genuinely unusual events?
- Data Cleaning: If an outlier is due to an error, it should be corrected or removed.
- Robust Statistical Methods: If the outlier is deemed legitimate, consider using robust statistical methods less sensitive to extreme values.
Conclusion: Upper and Lower Limits – Essential Tools in Statistics
Upper and lower limits are fundamental statistical tools used extensively in various fields. Understanding their calculation, interpretation, and limitations is vital for effective data analysis, process control, and decision-making. Remember that the choice of the appropriate formula and its interpretation are highly dependent on the specific statistical problem at hand. Always consider the context and the implications of your results. Properly calculated and interpreted upper and lower limits provide invaluable insights into data variability and help in drawing reliable conclusions.
Latest Posts
Latest Posts
-
Exercise 12 Microscopic Anatomy And Organization
May 10, 2025
-
Kirchhoffs Loop Rule Is A Statement Of
May 10, 2025
-
How Is Compound Similar To A Homogeneous Mixture
May 10, 2025
-
Neither Enzyme Works At A Ph Of
May 10, 2025
-
Complete The Abundance Diagram Below By Identifying
May 10, 2025
Related Post
Thank you for visiting our website which covers about Upper Limit And Lower Limit Formula Statistics . We hope the information provided has been useful to you. Feel free to contact us if you have any questions or need further assistance. See you next time and don't miss to bookmark.