What Does Area Under The Curve Mean In Statistics
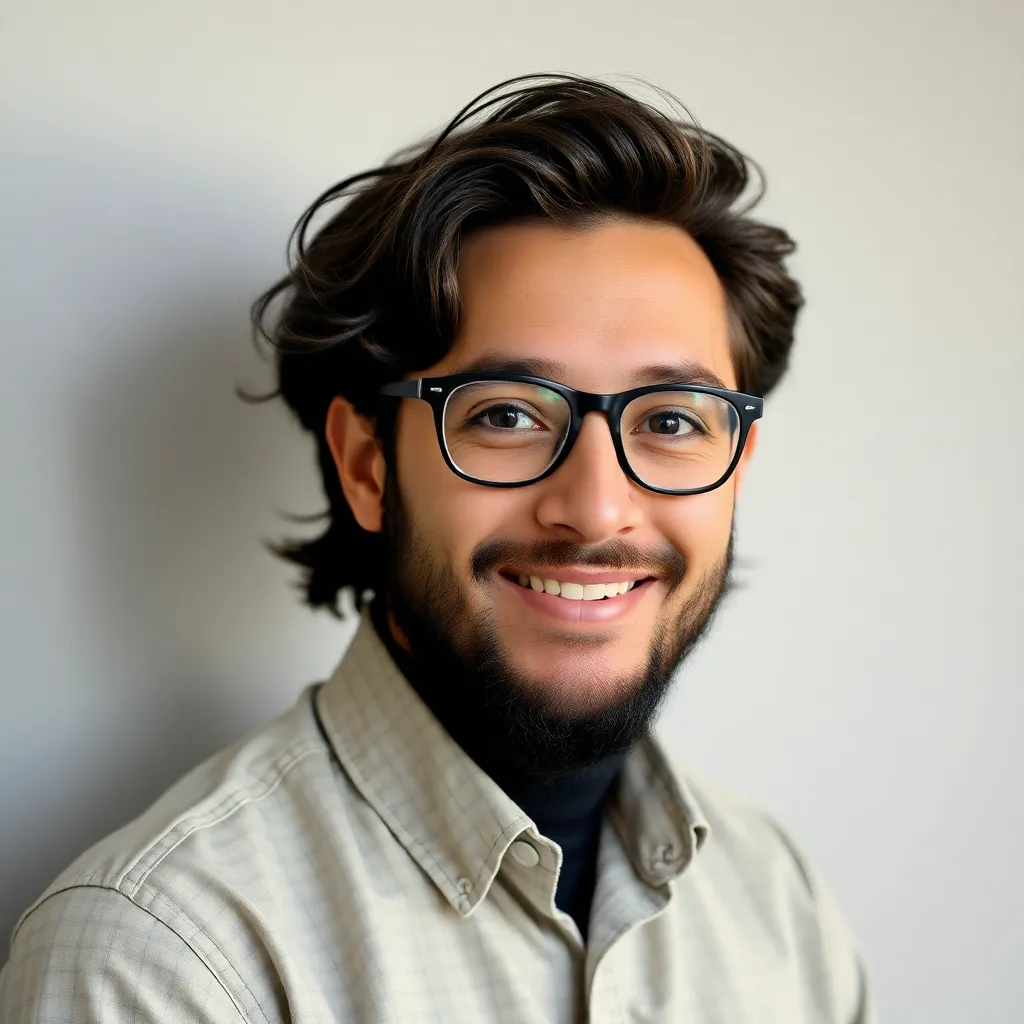
Muz Play
May 12, 2025 · 5 min read
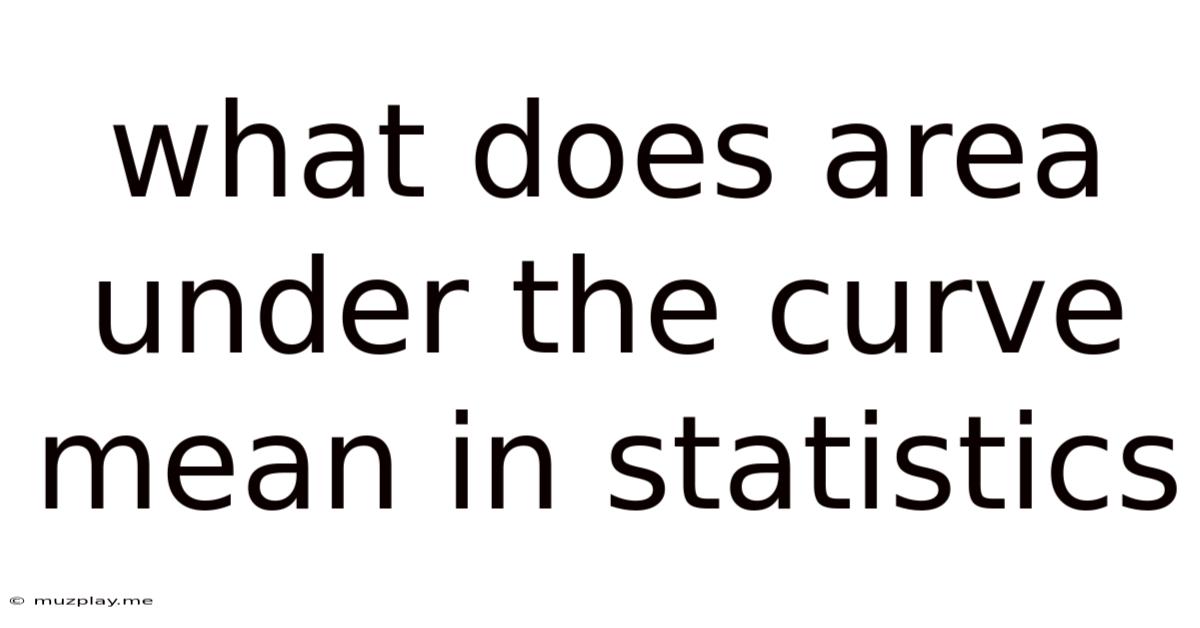
Table of Contents
What Does Area Under the Curve Mean in Statistics?
The area under the curve (AUC) is a fundamental concept in statistics, particularly in the context of probability distributions and hypothesis testing. It represents the probability associated with a given range of values within a distribution. While seemingly simple, understanding the AUC has wide-reaching implications across various statistical applications, from evaluating model performance to interpreting confidence intervals. This comprehensive guide will delve into the intricacies of AUC, explaining its meaning, calculation methods, and applications across different statistical domains.
Understanding Probability Distributions and their Curves
Before diving into the AUC, we need to grasp the concept of a probability distribution. A probability distribution describes the likelihood of different outcomes for a random variable. This is often visually represented as a curve, where the area under the curve (AUC) for a specific interval corresponds to the probability of the random variable falling within that interval.
Types of Probability Distributions
Various types of probability distributions exist, each with unique characteristics and applications. Some common examples include:
-
Normal Distribution: Also known as the Gaussian distribution, it's a bell-shaped curve, symmetrical around the mean. Many natural phenomena follow a normal distribution. The AUC for a given range under the normal curve represents the probability of observing a value within that range.
-
Exponential Distribution: This distribution is used to model the time until an event occurs. It's characterized by a decaying curve. The AUC for a specific interval represents the probability of the event occurring within that time frame.
-
Uniform Distribution: This distribution assigns equal probability to all values within a specific range. The curve is a horizontal line, and the AUC is simply the area of a rectangle.
-
Binomial Distribution: This discrete distribution models the probability of a certain number of successes in a fixed number of independent Bernoulli trials (experiments with only two outcomes). The AUC in this case is the sum of probabilities for specific success counts.
Calculating the Area Under the Curve (AUC)
Calculating the AUC depends on the type of distribution and the complexity of the curve. Several methods exist:
1. Analytical Methods:
For well-defined distributions like the normal distribution, analytical formulas exist to calculate the AUC. These formulas rely on integral calculus, directly computing the area under the curve. This approach is precise but requires knowledge of the specific distribution and its mathematical function.
2. Numerical Integration:
When analytical formulas aren't available, numerical integration techniques are used. These methods approximate the area under the curve by breaking it down into smaller shapes (rectangles, trapezoids) and summing their areas. Common techniques include:
- Trapezoidal Rule: Approximates the curve as a series of trapezoids.
- Simpson's Rule: Uses parabolic curves to approximate segments of the curve.
- Gaussian Quadrature: Employs strategically chosen points to improve accuracy.
3. Monte Carlo Simulation:
For complex distributions or high-dimensional problems, Monte Carlo simulation can be employed. This method involves generating a large number of random samples from the distribution and using the proportion of samples falling within the interval of interest to estimate the AUC. The accuracy increases with the number of samples.
AUC in Different Statistical Contexts
The AUC finds applications across numerous statistical domains:
1. Hypothesis Testing:
In hypothesis testing, the AUC can be used to determine the p-value, the probability of observing the obtained results (or more extreme results) if the null hypothesis is true. A low p-value (typically below a significance level like 0.05) provides evidence against the null hypothesis. The AUC is intrinsically linked to the p-value's calculation in many statistical tests.
2. Receiver Operating Characteristic (ROC) Curve Analysis:
In machine learning and diagnostic testing, the AUC of the ROC curve is a crucial metric for evaluating the performance of a binary classification model. The ROC curve plots the true positive rate (sensitivity) against the false positive rate (1-specificity) at various classification thresholds. The AUC of the ROC curve represents the probability that the model will rank a randomly chosen positive instance higher than a randomly chosen negative instance. A higher AUC indicates better discriminative power of the model.
Understanding the ROC Curve and AUC: A perfect classifier would have an AUC of 1.0, indicating it perfectly separates positive and negative instances. A random classifier would have an AUC of 0.5. Values between 0.5 and 1.0 reflect varying degrees of classifier performance.
3. Confidence Intervals:
Confidence intervals provide a range of plausible values for a population parameter. The AUC plays a role in constructing confidence intervals for parameters estimated from sample data. For example, the AUC under a sampling distribution can be used to determine the width of the confidence interval.
4. Survival Analysis:
In survival analysis, the AUC is relevant in evaluating the predictive performance of models that estimate the probability of an event (like death or failure) occurring within a specific time frame. The AUC of a survival curve provides insights into the model's ability to discriminate between individuals with different survival probabilities.
Interpretation and Limitations of AUC
While the AUC provides valuable information, it's crucial to interpret it within its context and acknowledge its limitations:
-
Context is Key: The AUC's meaning and importance vary depending on the specific application. A high AUC might be crucial in medical diagnosis but less critical in other domains.
-
Not a Substitute for Other Metrics: The AUC should be considered alongside other relevant metrics. In classification tasks, for example, accuracy, precision, and recall provide a more comprehensive picture of model performance.
-
Sensitivity to Class Imbalance: In datasets with highly imbalanced classes (significantly more instances of one class than another), the AUC might not accurately reflect the model's true performance.
Conclusion
The area under the curve is a powerful and versatile concept in statistics with applications spanning diverse fields. Understanding its meaning, calculation methods, and interpretations is essential for correctly interpreting statistical results and making informed decisions based on data analysis. From hypothesis testing to model evaluation, the AUC provides valuable insights into probability distributions and the performance of statistical models. However, remember to always consider the AUC in conjunction with other relevant metrics and understand its limitations to avoid misinterpretations. Careful consideration of the specific context and limitations ensures the effective use of the AUC in making sound data-driven decisions.
Latest Posts
Latest Posts
-
What Is Used To Measure Electric Current
May 12, 2025
-
Energy From Food Must Be Transformed Into The Bonds Of
May 12, 2025
-
How Do Enzymes Recognize Their Substrates
May 12, 2025
-
Monocot Leaf Vs Dicot Leaf Cross Section
May 12, 2025
-
The Final Acceptor For Electrons In Aerobic Cellular Respiration Is
May 12, 2025
Related Post
Thank you for visiting our website which covers about What Does Area Under The Curve Mean In Statistics . We hope the information provided has been useful to you. Feel free to contact us if you have any questions or need further assistance. See you next time and don't miss to bookmark.