What Is An Experiment In Statistics
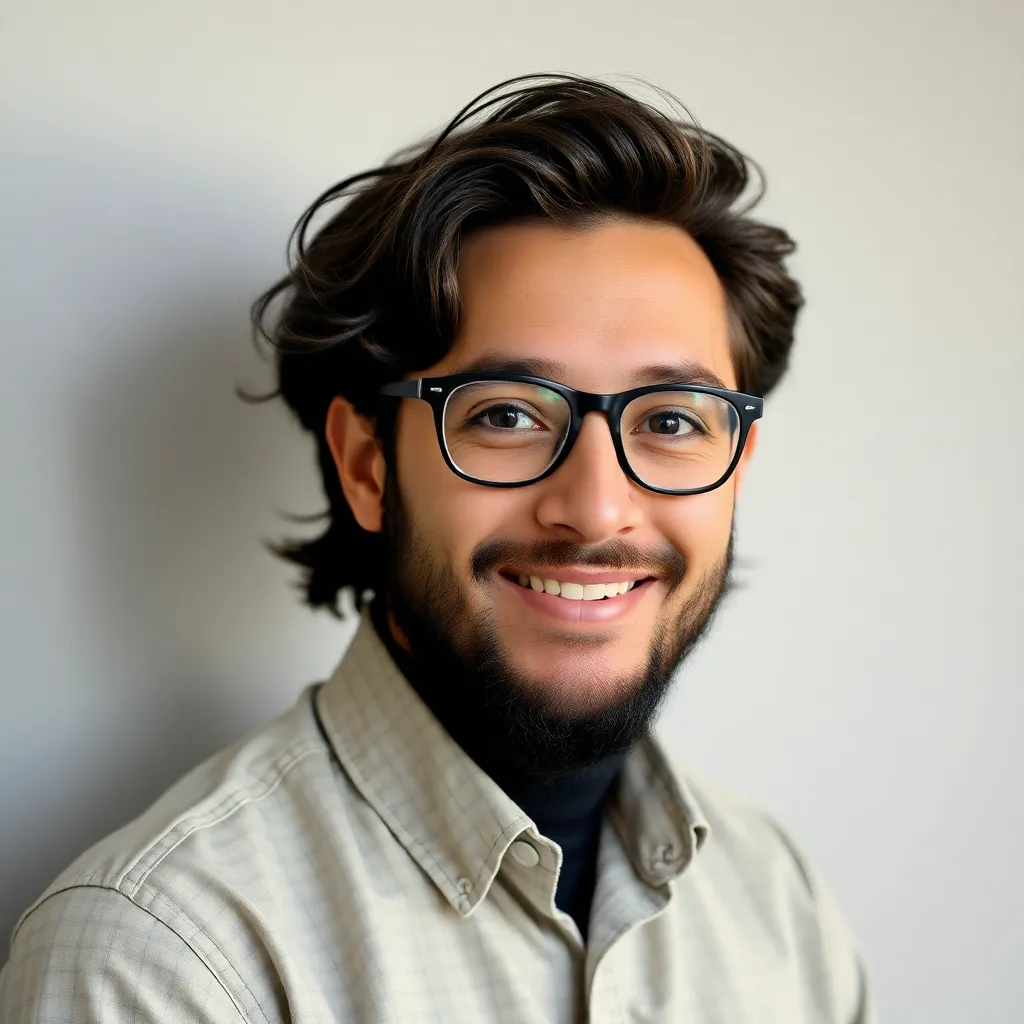
Muz Play
Apr 15, 2025 · 7 min read

Table of Contents
What is an Experiment in Statistics? A Comprehensive Guide
Understanding the concept of an experiment in statistics is crucial for anyone involved in data analysis, research, or decision-making. It's the cornerstone of causal inference, allowing us to move beyond simple observation and delve into the realm of cause and effect. This comprehensive guide will delve into the intricacies of statistical experimentation, covering everything from its fundamental definition to advanced design considerations.
Defining a Statistical Experiment
At its core, a statistical experiment is a controlled study where researchers manipulate one or more variables (independent variables) to observe their effect on another variable (dependent variable). Unlike observational studies, where researchers merely observe existing data, experiments actively intervene to establish a causal relationship. This intervention involves assigning subjects to different groups and manipulating the independent variable(s) systematically. The key differentiator is the random assignment of subjects, which is critical for minimizing bias and drawing valid conclusions.
Key Components of a Statistical Experiment
Several key elements define a well-designed statistical experiment:
-
Treatment Group(s): This group receives the treatment or intervention being studied. For example, in a medical trial, this could be the group receiving a new drug.
-
Control Group: This group does not receive the treatment and serves as a baseline for comparison. In our medical trial example, this would be the group receiving a placebo.
-
Independent Variable (IV): The variable manipulated by the researcher. This is the treatment or intervention. In our example, the independent variable is the drug.
-
Dependent Variable (DV): The variable being measured or observed. This is the outcome of interest. In the medical trial, the dependent variable could be blood pressure or symptom reduction.
-
Random Assignment: The process of assigning subjects to different groups randomly. This helps ensure that the groups are comparable at the start of the experiment and minimizes the risk of confounding variables.
-
Replication: Repeating the experiment multiple times with different subjects to confirm the results and increase the reliability of the findings.
Types of Experimental Designs
The design of an experiment is crucial to its success. Several types of experimental designs exist, each with its strengths and weaknesses:
1. Completely Randomized Design (CRD)
This is the simplest experimental design. Subjects are randomly assigned to treatment groups. It's suitable when there's no significant variability among the experimental units.
Example: Testing the effectiveness of three different fertilizers on plant growth. Plants are randomly assigned to pots, and each pot receives a different fertilizer.
2. Randomized Block Design (RBD)
In RBD, subjects are grouped into blocks based on a relevant characteristic before random assignment to treatment groups. This helps reduce variability within blocks, improving the precision of the experiment.
Example: Testing the effectiveness of two different teaching methods. Students are first grouped by their prior academic performance (blocks), and then randomly assigned to the two teaching methods within each block.
3. Factorial Design
This design involves more than one independent variable (factor). It allows researchers to examine the effects of each factor individually, as well as their interaction effects.
Example: Testing the effect of both fertilizer type and watering frequency on plant growth. This design would involve multiple treatment groups representing different combinations of fertilizer and watering frequency.
4. Latin Square Design
This is a special type of design used when there are two blocking factors (e.g., time of day and location). It ensures that each treatment appears only once in each row and column.
5. Split-Plot Design
This is used when there are two factors, one applied to whole plots and the other applied to subplots within each whole plot. This design is commonly used in agricultural research.
Controlling for Confounding Variables
A critical aspect of experimental design is controlling for confounding variables – variables that could influence the dependent variable but are not the independent variable of interest. These can significantly bias the results. Several methods help control confounding variables:
-
Randomization: Randomly assigning subjects to treatment groups helps to distribute confounding variables evenly across groups, minimizing their impact.
-
Matching: Matching subjects on relevant characteristics (e.g., age, gender) before assignment to treatment groups can help balance confounding variables.
-
Blocking: Grouping subjects into blocks based on confounding variables before random assignment can reduce their influence.
-
Statistical Control: Using statistical techniques like analysis of covariance (ANCOVA) can control for the effects of confounding variables after the experiment is conducted.
Analyzing Experimental Data
Once the experiment is complete, the data needs to be analyzed to draw valid conclusions. Common statistical methods for analyzing experimental data include:
-
t-tests: Used to compare the means of two groups.
-
Analysis of Variance (ANOVA): Used to compare the means of three or more groups.
-
Regression Analysis: Used to model the relationship between the independent and dependent variables.
-
Chi-square test: Used to analyze categorical data.
The choice of statistical method depends on the type of data collected and the research question. It's crucial to select the appropriate test and interpret the results correctly to avoid misinterpretations.
Ethical Considerations in Experimental Design
Ethical considerations are paramount in any experiment, especially those involving human or animal subjects. Researchers must adhere to strict ethical guidelines, which typically include:
-
Informed consent: Participants must be fully informed about the purpose, procedures, and risks involved in the experiment and provide their voluntary consent.
-
Minimizing harm: Researchers must take all necessary steps to minimize any potential harm to participants.
-
Confidentiality: Participant data must be kept confidential and protected.
-
Debriefing: Participants should be debriefed after the experiment, providing them with information about the study's results and answering any questions they may have.
Institutional Review Boards (IRBs) play a crucial role in overseeing the ethical conduct of research involving human subjects.
Interpreting Results and Drawing Conclusions
The final step in a statistical experiment is interpreting the results and drawing valid conclusions. This involves:
-
Statistical Significance: Determining whether the observed effect is statistically significant, meaning it is unlikely to have occurred by chance. P-values are commonly used to assess statistical significance.
-
Effect Size: Assessing the magnitude of the observed effect. A statistically significant effect may not be practically significant if the effect size is small.
-
Confidence Intervals: Providing a range of values within which the true population effect is likely to fall.
-
Generalizability: Determining the extent to which the findings can be generalized to other populations or settings. The sample size and the representativeness of the sample are crucial factors here.
Examples of Experiments Across Disciplines
Statistical experiments are employed across numerous fields:
-
Medicine: Clinical trials testing the efficacy of new drugs or treatments.
-
Psychology: Experiments investigating the impact of various interventions on behavior or cognitive processes.
-
Education: Experiments comparing different teaching methods or educational programs.
-
Marketing: A/B testing to optimize website design or advertising campaigns.
-
Agriculture: Experiments evaluating the effectiveness of different fertilizers or farming techniques.
Advanced Experimental Designs and Techniques
Beyond the basic designs, researchers utilize advanced techniques to handle more complex research questions:
-
Adaptive Designs: Experiments that adjust their design based on accumulating data.
-
Sequential Analysis: Analyzing data as it becomes available, allowing for early termination of the experiment if significant results are found.
-
Bayesian Experimental Design: Incorporating prior knowledge into the experimental design using Bayesian methods.
-
Meta-analysis: Combining results from multiple experiments to obtain a more precise estimate of an effect.
Conclusion
Statistical experiments are powerful tools for establishing causal relationships and answering research questions. By carefully designing and executing experiments, controlling for confounding variables, and employing appropriate statistical methods, researchers can obtain reliable and valid results that contribute to knowledge and inform decision-making across various disciplines. Understanding the principles of experimental design and analysis is essential for anyone seeking to engage with quantitative research. The careful consideration of ethical implications throughout the entire process is paramount, ensuring the integrity and responsible application of this valuable methodology.
Latest Posts
Latest Posts
-
The Average Kinetic Energy Of The Gas Molecules Is
May 09, 2025
-
Aldehydes And Ketones May Be Reduced To
May 09, 2025
-
Can Rate Constant K Be Negative
May 09, 2025
-
An Electrolyte Will Yield Ions By Undergoing A
May 09, 2025
-
Reactants Of The Electron Transport Chain
May 09, 2025
Related Post
Thank you for visiting our website which covers about What Is An Experiment In Statistics . We hope the information provided has been useful to you. Feel free to contact us if you have any questions or need further assistance. See you next time and don't miss to bookmark.