What Is An Individual In Statistics
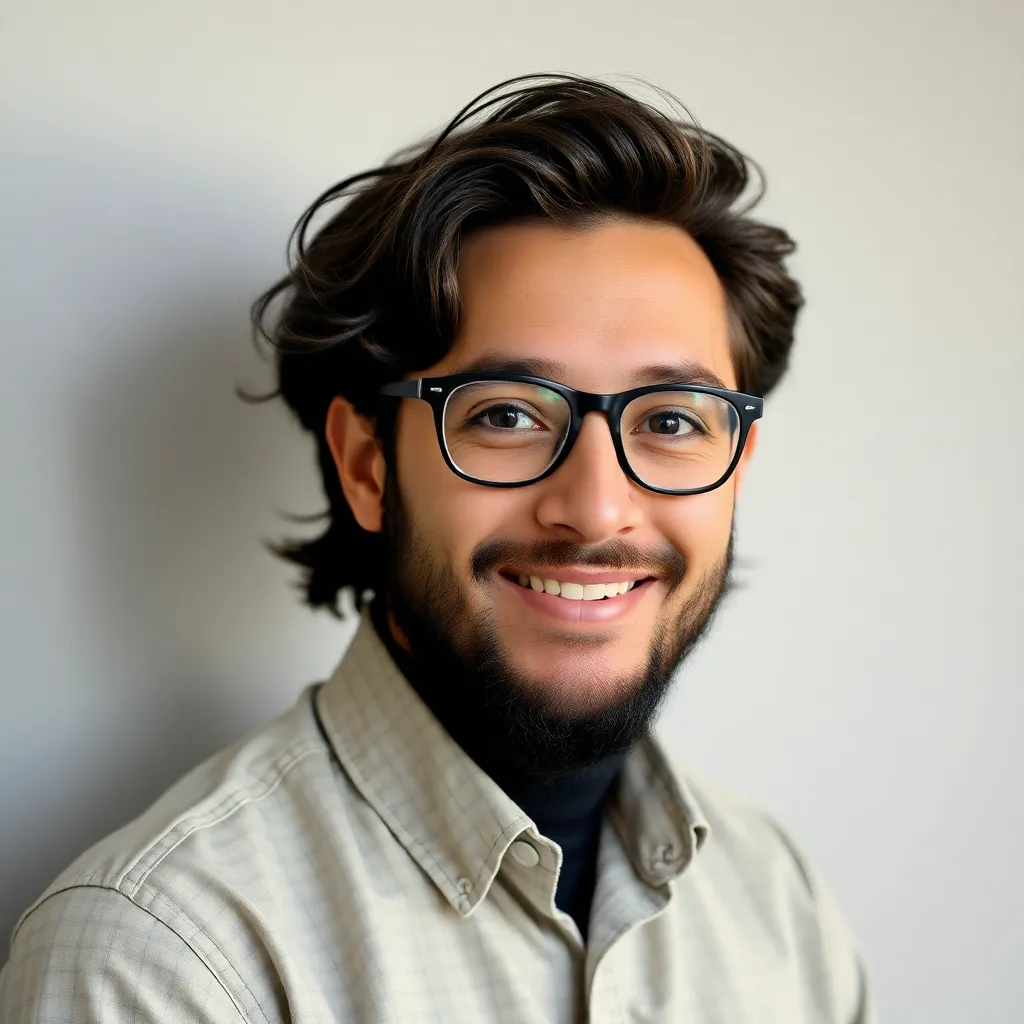
Muz Play
Apr 08, 2025 · 7 min read

Table of Contents
What is an Individual in Statistics? A Deep Dive into Statistical Units
Understanding the fundamental concepts in statistics is crucial for anyone looking to analyze data effectively. One of the most basic, yet often overlooked, concepts is the individual, also known as a statistical unit, observational unit, or element. This seemingly simple term forms the bedrock upon which all statistical analysis rests. This comprehensive guide will explore the multifaceted nature of the individual in statistics, clarifying its meaning, significance, and implications for various statistical procedures.
Defining the Individual in Statistics
In the simplest terms, an individual in statistics refers to a single entity being observed or measured in a study. It's the basic unit of observation from which data is collected. This entity can take many forms, depending on the context of the research. Let's consider some examples:
Examples of Individuals in Different Contexts:
- Medical Research: An individual might be a single patient participating in a clinical trial, whose blood pressure, heart rate, and other vital signs are measured.
- Social Sciences: An individual could be a person responding to a survey about their political views, consumer habits, or social attitudes.
- Environmental Science: An individual could be a single tree in a forest, its height and diameter being measured to assess the health of the ecosystem.
- Business Analytics: An individual could be a single customer whose purchase history, website activity, and customer service interactions are tracked to improve marketing strategies.
- Educational Research: An individual might be a student whose test scores, attendance rate, and engagement in class are analyzed to evaluate the effectiveness of a teaching method.
It's important to note that the definition of an "individual" is context-dependent. What constitutes an individual in one study might be a group in another. For example, while a single patient is an individual in a medical trial, a family unit might be considered the individual in a study on family dynamics. Similarly, a single tree is the individual in a forestry study, but a forest itself could be the individual unit in a broader ecological investigation comparing forest characteristics across various regions.
The Importance of Identifying the Individual
Accurately identifying the individual is paramount for several reasons:
-
Data Collection: The definition of the individual dictates how data is collected. If the individual is a patient, data will be collected from individual medical records. If the individual is a household, data might involve questionnaires completed by each household member or a representative of the household. Misidentifying the individual can lead to biased or inaccurate data collection.
-
Data Analysis: The choice of statistical methods depends heavily on the characteristics of the individual. Methods suitable for analyzing data from individual patients might not be appropriate for analyzing data from family units. Understanding the characteristics of your individuals allows you to choose the appropriate statistical tests.
-
Interpretation of Results: The conclusions drawn from a statistical analysis are directly related to the individual being studied. Understanding the nature of the individual is crucial for interpreting the results in a meaningful and contextually appropriate manner. Incorrect identification of the individual can lead to erroneous interpretations and flawed conclusions.
-
Generalizability of Findings: The representativeness of the sample of individuals is key to generalizing the findings to a larger population. Careful selection of individuals ensures the results can be reliably extrapolated to the wider population of interest.
Levels of Measurement and the Individual
The type of data collected from individuals also depends on the level of measurement. There are four main levels of measurement:
-
Nominal: This involves categorizing individuals into distinct groups. Examples include gender (male/female), eye color (blue, brown, green), or type of car owned (sedan, SUV, truck).
-
Ordinal: This involves ranking individuals based on some characteristic. Examples include educational attainment (high school, bachelor's, master's), customer satisfaction ratings (very satisfied, satisfied, neutral, dissatisfied, very dissatisfied), or socioeconomic status (low, middle, high).
-
Interval: This involves measuring individuals on a scale with equal intervals between values but without a true zero point. Examples include temperature in Celsius or Fahrenheit, or year of birth.
-
Ratio: This involves measuring individuals on a scale with equal intervals and a true zero point. Examples include height, weight, income, or age.
The level of measurement dictates the types of statistical analyses that can be performed. For example, you cannot calculate a meaningful average for nominal data (e.g., average eye color), but you can calculate the average height (ratio data). Understanding the level of measurement of your data is crucial for choosing the appropriate statistical techniques.
Sampling and the Individual
In most statistical studies, it’s impractical or impossible to collect data from every individual in the population of interest. This is where sampling comes in. A sample is a subset of the population selected to represent the entire population. The way individuals are selected for a sample greatly impacts the validity and generalizability of the results. Common sampling methods include:
- Simple Random Sampling: Each individual in the population has an equal chance of being selected.
- Stratified Sampling: The population is divided into strata (subgroups), and a random sample is taken from each stratum. This ensures representation from all subgroups.
- Cluster Sampling: The population is divided into clusters (groups), and a random sample of clusters is selected. All individuals within the selected clusters are included in the sample.
- Convenience Sampling: Individuals are selected based on their availability and accessibility. This method is prone to bias.
The selection of individuals for a sample should be carefully planned to minimize bias and ensure the sample is representative of the population of interest. An unrepresentative sample can lead to inaccurate and misleading conclusions.
Individuals and Data Visualization
Individuals are also central to data visualization. Graphs and charts are used to represent data collected from individuals. Different visualization techniques are suitable for different types of data and levels of measurement. For example:
- Bar charts: Useful for displaying frequencies or proportions of categorical data (nominal data).
- Histograms: Useful for displaying the distribution of numerical data (interval or ratio data).
- Scatter plots: Useful for displaying the relationship between two numerical variables.
- Box plots: Useful for comparing the distribution of a numerical variable across different groups.
The choice of visualization technique depends on the type of data and the research question. Effective visualization techniques help communicate the findings of statistical analysis in a clear and concise manner.
Dealing with Missing Data and Individuals
Missing data is a common problem in statistical analysis. Missing data can occur when information is not collected from some individuals due to various reasons (e.g., non-response in surveys, equipment malfunction, or participant dropout in experiments). Dealing with missing data requires careful consideration, as it can bias the results. Strategies for handling missing data include:
- Deletion: Removing individuals with missing data from the analysis. This can lead to loss of information and bias if the missing data is not missing completely at random (MCAR).
- Imputation: Replacing missing values with estimated values. There are various imputation methods, including mean imputation, regression imputation, and multiple imputation.
- Model-based approaches: Incorporating missing data mechanisms into the statistical model used for analysis.
The best approach for handling missing data depends on the nature of the missing data, the amount of missing data, and the type of statistical analysis being performed. Ignoring missing data can lead to inaccurate and misleading conclusions.
The Individual in Advanced Statistical Methods
The concept of the individual extends beyond basic descriptive and inferential statistics. It plays a crucial role in more advanced methods like:
- Regression Analysis: Individuals are the units upon which the independent and dependent variables are measured.
- Time Series Analysis: Individuals can be individual entities tracked over time (e.g., stock prices, temperature readings).
- Survival Analysis: Individuals are the units being followed over time to observe a specific event (e.g., death, machine failure).
- Multivariate Analysis: Individuals are measured on multiple variables simultaneously.
In all these advanced methods, the accurate identification and understanding of the individual are crucial for appropriate model specification, interpretation of results, and drawing valid conclusions.
Conclusion
The individual, or statistical unit, is the cornerstone of all statistical analyses. A thorough understanding of what constitutes an individual in a specific study is essential for proper data collection, selection of appropriate statistical methods, interpretation of results, and the generalizability of findings. Careful consideration of the individual, its characteristics, and the level of measurement of associated data is paramount to conducting valid and meaningful statistical research. Ignoring this fundamental concept can lead to erroneous conclusions and a misinterpretation of the data. Therefore, always prioritize the accurate identification and understanding of your individuals before embarking on any statistical analysis.
Latest Posts
Latest Posts
-
What Type Of Chemical Bond Stabilizes Crystals Of Table Salt
Apr 17, 2025
-
Energy Is Stored Long Term In The Bonds Of
Apr 17, 2025
-
Which Of The Following Is An Anti Conformation For Butane
Apr 17, 2025
-
Which Of The Following Is Not A Functional Joint Classification
Apr 17, 2025
-
Difference Between Chemical Change And Chemical Property
Apr 17, 2025
Related Post
Thank you for visiting our website which covers about What Is An Individual In Statistics . We hope the information provided has been useful to you. Feel free to contact us if you have any questions or need further assistance. See you next time and don't miss to bookmark.