What Is The Best Measure Of Variability
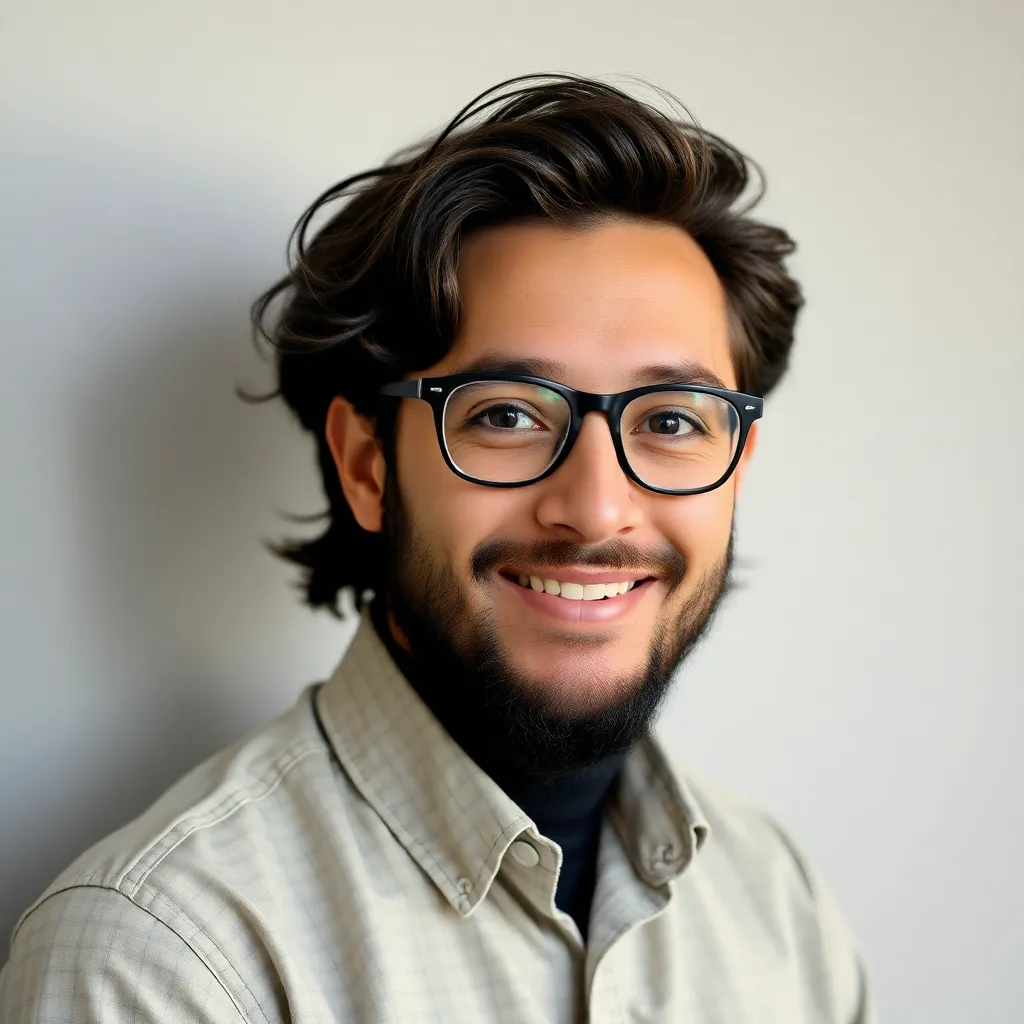
Muz Play
Apr 23, 2025 · 7 min read

Table of Contents
What is the Best Measure of Variability? A Deep Dive into Dispersion Statistics
Choosing the "best" measure of variability depends entirely on the context. There's no single universally superior metric; the optimal choice hinges on the specific characteristics of your data and the goals of your analysis. This comprehensive guide explores the most common measures of variability – range, interquartile range, variance, and standard deviation – detailing their strengths, weaknesses, and appropriate applications. We’ll also delve into less frequently discussed measures like the mean absolute deviation, shedding light on when each is most effective. By the end, you'll be equipped to make informed decisions about which measure of variability best suits your statistical needs.
Understanding Variability: Why It Matters
Variability, or dispersion, describes the spread or scatter of data points around a central tendency (usually the mean or median). Understanding variability is crucial because it reveals:
- Data Distribution: How tightly clustered or widely dispersed the data is. A low variability suggests homogeneity, while high variability indicates heterogeneity.
- Data Reliability: Highly variable data might indicate measurement error or inherent randomness, impacting the reliability of conclusions drawn from the data.
- Data Comparison: Comparing the variability of different datasets allows for insightful comparisons between groups or populations.
- Outlier Detection: Measures of variability help identify unusually large or small data points that could skew the analysis.
Common Measures of Variability: A Comparative Analysis
Let's examine the most frequently used measures of variability in detail:
1. Range: Simple, but Sensitive to Outliers
The range is the simplest measure of variability, calculated as the difference between the maximum and minimum values in a dataset.
Formula: Range = Maximum Value – Minimum Value
Strengths:
- Easy to calculate and understand. It requires minimal computational effort and is intuitively grasped.
Weaknesses:
- Highly sensitive to outliers. A single extreme value can drastically inflate the range, providing a misleading picture of the overall variability.
- Ignores the distribution of data points. It only considers the two most extreme values, disregarding the spread of the remaining data.
When to use: The range is best suited for small datasets with minimal outliers, or as a quick preliminary assessment of variability before employing more robust measures.
2. Interquartile Range (IQR): Robust to Outliers
The IQR is the difference between the third quartile (Q3) – the value separating the top 25% of the data – and the first quartile (Q1) – the value separating the bottom 25% of the data.
Formula: IQR = Q3 – Q1
Strengths:
- Robust to outliers. Unlike the range, the IQR is less affected by extreme values, providing a more stable measure of variability.
- Focuses on the central 50% of data. This makes it a more representative measure of the typical spread, excluding the influence of extreme outliers.
Weaknesses:
- Ignores information beyond the quartiles. It doesn't fully capture the variability of the entire dataset.
- Slightly more complex to calculate compared to the range, requiring the determination of quartiles.
When to use: The IQR is preferred when the dataset contains outliers or when a robust measure of variability is required.
3. Variance: The Foundation of Many Statistical Tests
The variance measures the average squared deviation of each data point from the mean. It quantifies how far the data points are spread from the mean, on average.
Formula: Variance (σ²) = Σ(xᵢ - μ)² / N (for population variance) s² = Σ(xᵢ - x̄)² / (n-1) (for sample variance)
Where:
- xᵢ represents each data point
- μ represents the population mean
- x̄ represents the sample mean
- N represents the population size
- n represents the sample size
Strengths:
- Considers all data points: The variance uses all data points in the calculation, providing a comprehensive measure of dispersion.
- Mathematical foundation for many statistical tests: Variance is a fundamental component in numerous statistical analyses, such as ANOVA and regression.
Weaknesses:
- Sensitive to outliers: Similar to the range, extreme values can significantly inflate the variance.
- Units are squared: The variance is expressed in squared units, which can be difficult to interpret directly compared to the original data units.
When to use: Variance is commonly used in statistical inference, particularly when dealing with normally distributed data and employing methods like ANOVA or regression.
4. Standard Deviation: The Most Widely Used Measure
The standard deviation is the square root of the variance. It shares the same strengths and weaknesses as the variance but offers a more easily interpretable result, expressed in the original units of the data.
Formula: Standard Deviation (σ) = √Variance (for population standard deviation) s = √s² (for sample standard deviation)
Strengths:
- Interpretable units: The standard deviation is expressed in the same units as the original data, making it easier to understand the magnitude of variability.
- Widely used and understood: It's the most commonly used measure of variability in many fields.
- Useful for comparison: Standard deviations from different datasets can be compared directly.
Weaknesses:
- Sensitive to outliers: Extreme values can still significantly influence the standard deviation.
- Assumes a normal distribution: While not strictly required, the interpretation of standard deviation is most meaningful when data follows a roughly normal distribution.
When to use: The standard deviation is the most popular measure of variability and is appropriate for many applications, particularly when dealing with approximately normally distributed data. It is commonly used to describe the spread of data in descriptive statistics and is crucial for many inferential statistical tests.
5. Mean Absolute Deviation (MAD): An Alternative to Standard Deviation
The MAD calculates the average absolute deviation of each data point from the mean. It provides a measure of variability that is less sensitive to outliers compared to the standard deviation.
Formula: MAD = Σ|xᵢ - μ| / N (for population MAD) MAD = Σ|xᵢ - x̄| / n (for sample MAD)
Strengths:
- Robust to outliers: Outliers have less impact on the MAD compared to the standard deviation.
- Easy to interpret: Like the standard deviation, it's expressed in the original units of measurement.
Weaknesses:
- Less commonly used: Compared to the standard deviation, it's less frequently used in statistical analysis.
- Less efficient estimator: It's less efficient than the standard deviation in estimating population parameters when the data is normally distributed.
When to use: The MAD is a suitable alternative to the standard deviation when outliers are a significant concern and robustness is prioritized over statistical efficiency.
Choosing the Right Measure: A Practical Guide
The selection of the best measure of variability depends on several factors:
- Data Distribution: For normally distributed data, the standard deviation is usually preferred. For skewed data, the IQR or MAD might be more appropriate.
- Presence of Outliers: If outliers are present, the IQR or MAD provide more robust measures than the range, variance, or standard deviation.
- Purpose of Analysis: For simple descriptive summaries, the range or IQR might suffice. For more advanced statistical analysis, the variance or standard deviation are generally necessary.
- Audience: The complexity of the chosen measure should be appropriate for the intended audience. For a non-technical audience, simpler measures like the range or IQR might be more easily understood.
Beyond the Basics: Advanced Measures of Variability
While the measures above are widely used, other specialized measures exist:
- Coefficient of Variation: This expresses variability relative to the mean, allowing for comparisons across datasets with different scales.
- Standard Error of the Mean: This measures the variability of sample means around the population mean, used in hypothesis testing.
- Skewness and Kurtosis: These describe the asymmetry and peakedness of the data distribution, respectively, which influence the interpretation of variability measures.
Conclusion: Context is Key
Selecting the "best" measure of variability isn't about choosing a single superior metric. Instead, the optimal choice depends critically on the specific context of your data and analysis goals. By carefully considering data distribution, the presence of outliers, the purpose of your analysis, and the audience for your results, you can confidently select the most appropriate measure of variability to accurately and effectively represent the spread in your data. This informed approach ensures that your statistical conclusions are reliable and meaningful. Remember to always consider the context and the limitations of each measure to obtain a robust and insightful understanding of your data's variability.
Latest Posts
Latest Posts
-
Organic Chemistry Is The Study Of What
Apr 23, 2025
-
Absorption Of Uv Visible Energy By A Molecule Results In
Apr 23, 2025
-
Why Do Scientists Apply The Concept Of Maximum Parsimony
Apr 23, 2025
-
Example Of A Short Informative Speech
Apr 23, 2025
-
Definition Of Scientific Method In Sociology
Apr 23, 2025
Related Post
Thank you for visiting our website which covers about What Is The Best Measure Of Variability . We hope the information provided has been useful to you. Feel free to contact us if you have any questions or need further assistance. See you next time and don't miss to bookmark.