What Is The Difference Between Discovery Science And Hypothesis-driven Science
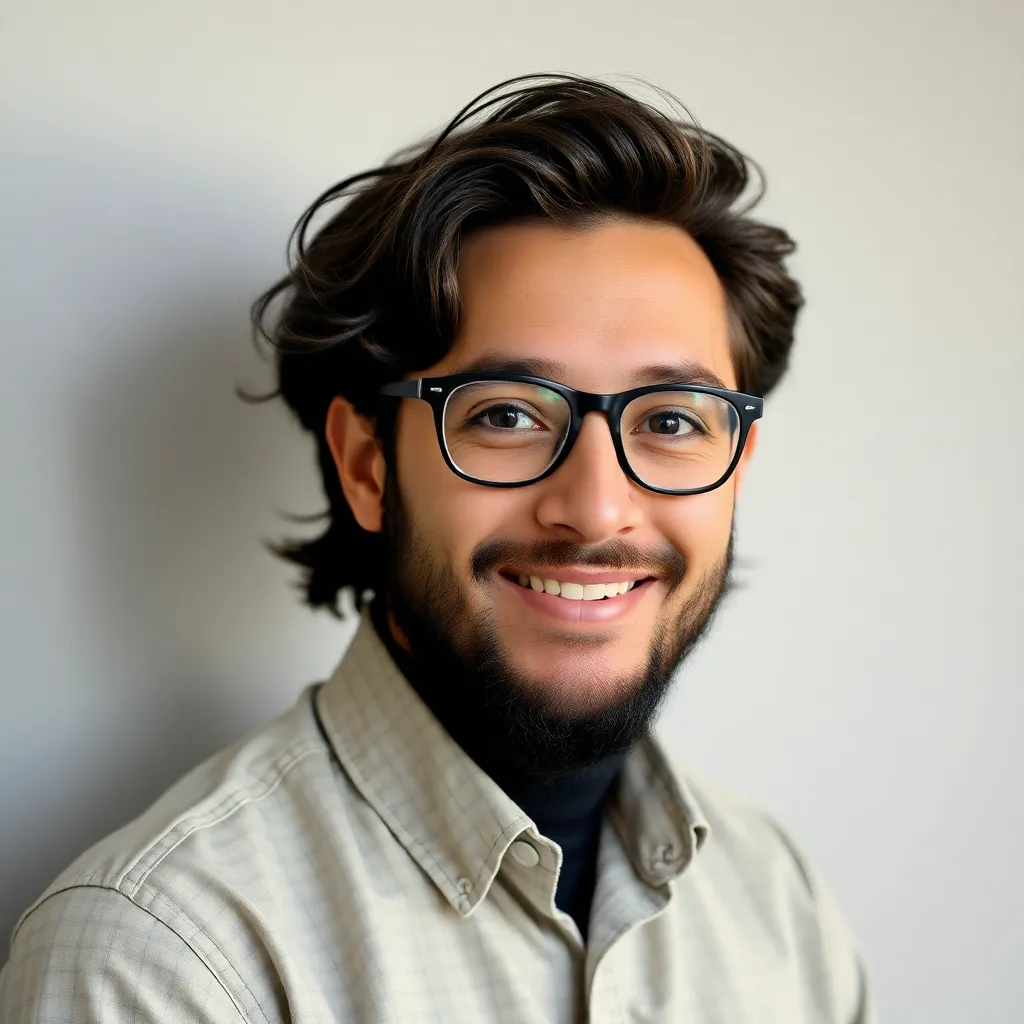
Muz Play
Apr 22, 2025 · 6 min read

Table of Contents
Delving into the Core Differences: Discovery Science vs. Hypothesis-Driven Science
The scientific method, a cornerstone of our understanding of the world, isn't a monolithic entity. It encompasses diverse approaches, each with its own strengths and limitations. Two prominent approaches are discovery science and hypothesis-driven science. While both contribute significantly to scientific advancement, their methodologies and goals differ considerably. This article will delve into the core distinctions between these two approaches, exploring their methodologies, applications, and the crucial role each plays in expanding our knowledge.
Discovery Science: Unveiling Nature's Secrets Through Observation
Discovery science, also known as descriptive science, is an exploratory approach that emphasizes observation and data collection without preconceived notions or hypotheses. It's about letting the data speak for itself, uncovering patterns and correlations that might lead to further investigation. Think of it as the "what" phase of scientific inquiry. It's the foundation upon which more targeted, hypothesis-driven research is often built.
Key Characteristics of Discovery Science:
- Observation-driven: The cornerstone of discovery science is meticulous observation of natural phenomena. This can involve qualitative observations (descriptions of color, texture, behavior) or quantitative measurements (numerical data).
- Data-centric: The focus is on collecting vast amounts of data, often using sophisticated technologies and techniques. This data is then analyzed to identify patterns, trends, and correlations.
- Exploratory: It's not constrained by pre-existing hypotheses. Researchers are open to unexpected findings and are willing to follow where the data leads.
- Inductive Reasoning: Discovery science employs inductive reasoning, moving from specific observations to broader generalizations. The goal is to identify patterns and formulate potential explanations, which can then be tested further.
- Examples: Many fields rely heavily on discovery science, including:
- Astronomy: Observing celestial bodies and their movements, leading to discoveries of new planets, galaxies, and phenomena.
- Biodiversity studies: Documenting the diversity of life on Earth, identifying new species, and understanding their relationships.
- Genomics: Sequencing genomes to understand the genetic makeup of organisms and identify disease-related genes.
- Big Data Analysis: Mining vast datasets to identify correlations and patterns related to climate change, epidemiology, etc.
Hypothesis-Driven Science: Testing Predictions and Refining Understanding
Hypothesis-driven science, in contrast, is a more targeted and structured approach. It starts with a hypothesis, a testable explanation for an observed phenomenon. The primary goal is to test this hypothesis through carefully designed experiments and rigorous analysis. This approach is often described as the "why" phase of scientific inquiry, aiming to explain the "what" discovered through discovery science.
Key Characteristics of Hypothesis-Driven Science:
- Hypothesis-based: The process begins with a specific, testable hypothesis, often based on prior knowledge and observations.
- Experimental design: Researchers design experiments to specifically test their hypotheses, manipulating variables to observe the effects.
- Deductive Reasoning: Hypothesis-driven science uses deductive reasoning, moving from general principles (the hypothesis) to specific predictions that can be tested.
- Falsifiability: A crucial aspect is that the hypothesis must be falsifiable—meaning that it's possible to design an experiment that could disprove it. If a hypothesis cannot be falsified, it's not scientifically testable.
- Control Groups: Experiments often involve control groups to provide a baseline for comparison, allowing researchers to isolate the effects of the manipulated variable.
- Statistical Analysis: Statistical methods are used to analyze the data and determine whether the results support or refute the hypothesis.
- Iteration and Refinement: The process is iterative. Results may lead to revisions of the hypothesis, further experimentation, and refinement of the understanding.
- Examples:
- Clinical trials: Testing the effectiveness of new drugs or treatments.
- Laboratory experiments: Investigating the effects of environmental factors on cell growth or chemical reactions.
- Controlled field studies: Assessing the impact of an intervention (e.g., conservation program) on an ecological community.
The Interplay and Synergy Between Discovery and Hypothesis-Driven Science
While seemingly distinct, discovery and hypothesis-driven science are not mutually exclusive. They often work in tandem, informing and complementing each other in a cyclical process. Discovery science can generate hypotheses, providing the raw material for hypothesis-driven research. Conversely, hypothesis-driven research can lead to new observations and further discovery.
For instance, the discovery of new species through biodiversity surveys (discovery science) could lead to hypotheses about their evolutionary relationships, habitat preferences, or roles in ecosystems. Testing these hypotheses through controlled experiments (hypothesis-driven science) might reveal unexpected patterns, further fueling discovery science through new observations and questions.
This cyclical relationship is crucial for scientific progress. Discovery science casts a wide net, uncovering new phenomena and generating hypotheses, while hypothesis-driven science provides the rigorous testing and refinement needed to validate or refute those hypotheses. Both approaches are essential for building a comprehensive understanding of the natural world.
The Importance of Data in Both Approaches
Data lies at the heart of both discovery and hypothesis-driven science. However, the nature and use of data differ. In discovery science, the data is primarily exploratory, used to identify patterns and correlations without pre-defined expectations. The focus is on descriptive statistics and visualization techniques to reveal meaningful relationships.
In contrast, hypothesis-driven science uses data to test specific predictions. Inferential statistics are employed to determine whether the observed results are likely due to chance or are truly indicative of a causal relationship between the variables. The focus is on controlled experiments and rigorous data analysis to validate or refute the hypothesis.
Overlapping Aspects: The Grey Area
While the distinction between the two is generally clear, there are situations where the line blurs. Some studies might start with a broad exploratory phase (discovery science) before focusing on specific hypotheses (hypothesis-driven science). Similarly, the results of a hypothesis-driven study might lead to new questions and further exploratory research.
The key difference remains in the primary goal: discovery science seeks to describe and identify patterns, while hypothesis-driven science seeks to test explanations and build causal models. The methodology employed will often reflect this central objective.
Limitations and Challenges
Each approach has its limitations. Discovery science can be limited by the scope of observations made and the potential biases influencing data collection. The sheer volume of data in many domains can also make pattern identification challenging without clear methodological frameworks.
Hypothesis-driven science can be restricted by the specific nature of hypotheses and the possibility of overlooking other influencing factors. It might also suffer from confirmation bias, where researchers tend to favor data supporting their hypothesis and neglect contradicting evidence. Furthermore, poorly designed experiments can lead to flawed conclusions.
Overcoming these limitations often involves integrating both approaches, employing rigorous methodologies, critically evaluating data, and remaining open to unexpected findings.
Conclusion: A Powerful Partnership for Scientific Advancement
Discovery science and hypothesis-driven science represent complementary approaches to scientific inquiry. Discovery science lays the groundwork by uncovering patterns and generating hypotheses, while hypothesis-driven science provides the rigorous testing and refinement needed to build a robust understanding of the world. Their synergy is crucial for advancing scientific knowledge across all disciplines. Recognizing their unique characteristics and their capacity for collaboration is vital for anyone engaged in scientific research or wishing to understand the ever-evolving landscape of scientific understanding. By understanding these differences, researchers can better design their investigations and approach the task of uncovering truth in a more effective manner, pushing the boundaries of human knowledge. The journey of scientific discovery is, indeed, a dynamic dance between observation, exploration, and rigorous testing.
Latest Posts
Latest Posts
-
Phospholipids Differ From Triglycerides In That Phospholipids
Apr 22, 2025
-
Is The Forward Reaction Endothermic Or Exothermic
Apr 22, 2025
-
The Brachial Surface Region Pertains To The Wrist
Apr 22, 2025
-
Similarities Between Sheep Brain And Human Brain
Apr 22, 2025
-
Give The Si Base Unit Of Each Of These Quantities
Apr 22, 2025
Related Post
Thank you for visiting our website which covers about What Is The Difference Between Discovery Science And Hypothesis-driven Science . We hope the information provided has been useful to you. Feel free to contact us if you have any questions or need further assistance. See you next time and don't miss to bookmark.