When Is Mean Greater Than Median
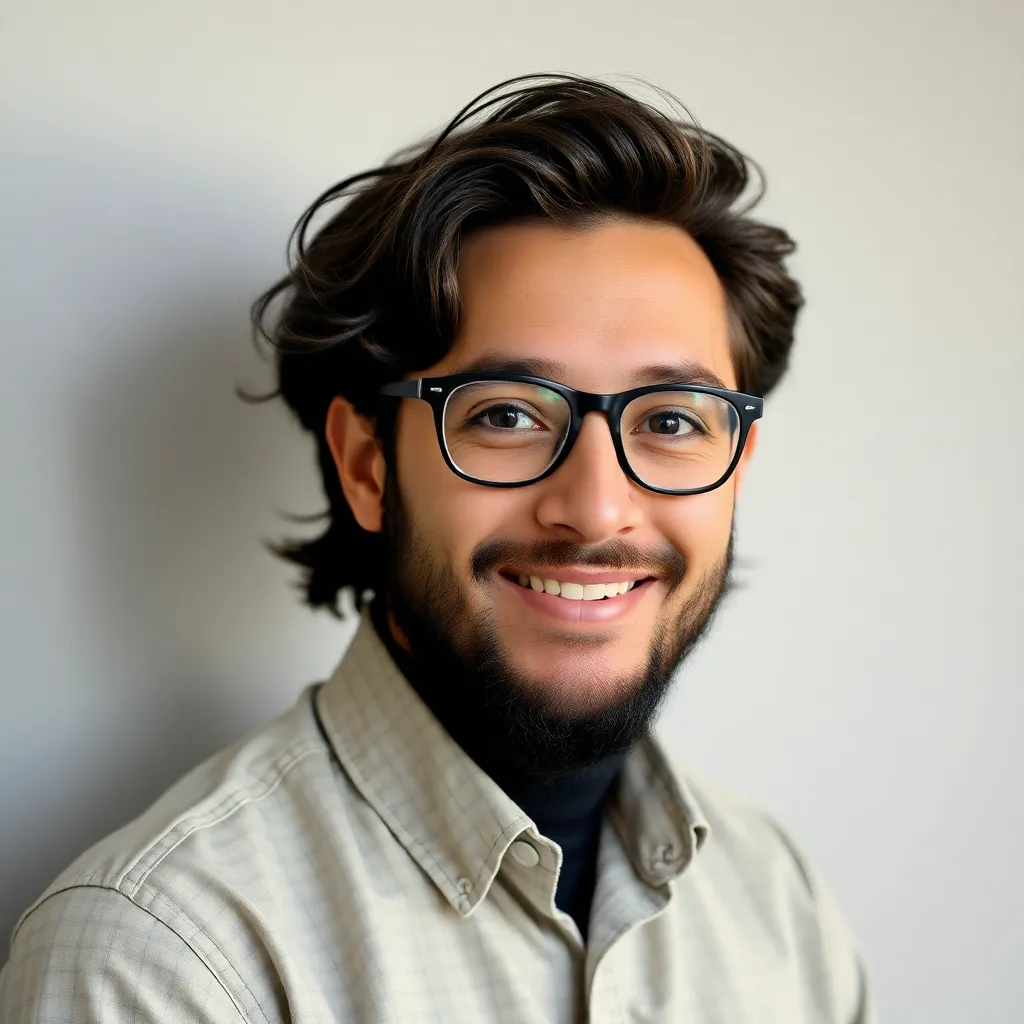
Muz Play
May 11, 2025 · 6 min read
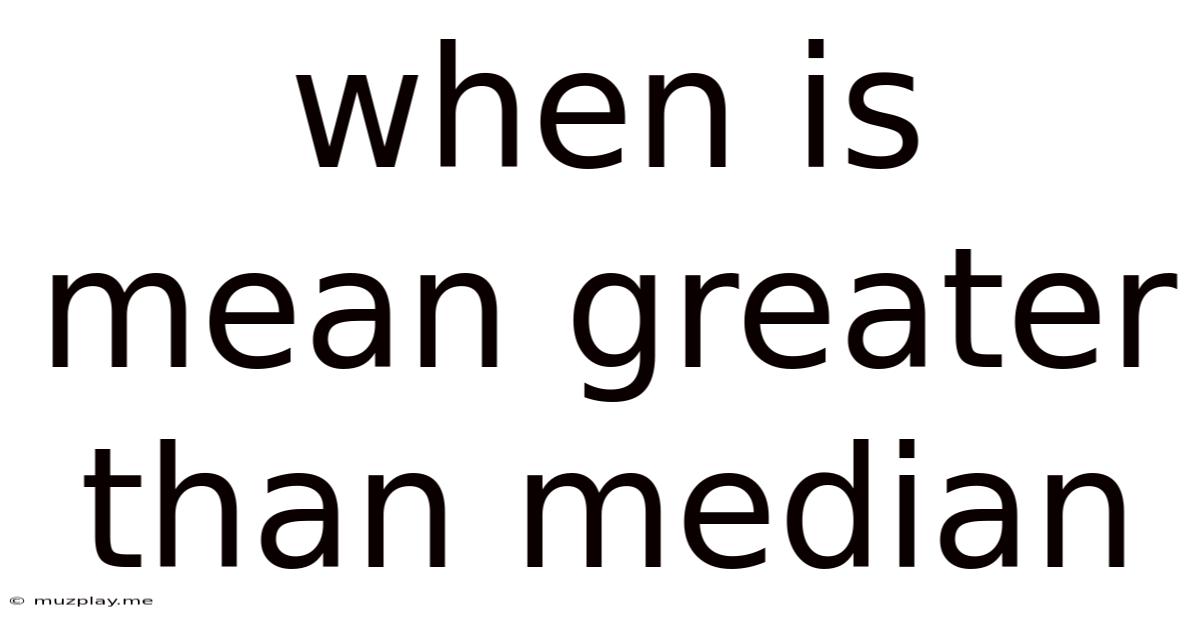
Table of Contents
When is the Mean Greater Than the Median? Understanding Skewed Distributions
The mean and the median are two fundamental measures of central tendency in statistics. While both aim to describe the "average" of a dataset, they do so in different ways. The mean is the average of all values, calculated by summing all values and dividing by the number of values. The median is the middle value when the data is ordered. Understanding when the mean is greater than the median provides crucial insights into the shape and nature of your data distribution. This article will delve deep into this relationship, exploring its implications and providing real-world examples.
The Significance of Mean vs. Median
The relationship between the mean and the median offers a powerful visual representation of the data's skewness. Skewness describes the asymmetry of a probability distribution. A perfectly symmetrical distribution, like a normal distribution, will have an equal mean and median. However, in many real-world datasets, this symmetry is absent.
- Symmetrical Distribution: Mean = Median. The data is evenly distributed around the central point.
- Right-Skewed Distribution (Positive Skew): Mean > Median. The tail extends towards higher values. A few extremely high values pull the mean upwards, while the median remains relatively unaffected by these outliers.
- Left-Skewed Distribution (Negative Skew): Mean < Median. The tail extends towards lower values. A few extremely low values pull the mean downwards, while the median stays relatively stable.
Understanding this relationship is critical for accurate data interpretation. Relying solely on the mean in a skewed distribution can be misleading, as it can be disproportionately influenced by extreme values (outliers). The median, being less sensitive to outliers, provides a more robust measure of central tendency in such cases.
Why the Mean is Greater Than the Median in Right-Skewed Distributions
In a right-skewed distribution, the long tail on the right side contains a few exceptionally high values. These high values significantly inflate the sum used to calculate the mean. The median, however, is unaffected by these extreme values; it simply represents the middle value in the ordered dataset. Therefore, the mean is pulled further to the right than the median, resulting in a mean greater than the median.
Let's illustrate this with an example:
Consider the following dataset representing the salaries of employees in a small company:
$30,000, $35,000, $40,000, $45,000, $50,000, $55,000, $1,000,000
- Median: $45,000 (the middle value)
- Mean: Approximately $170,000 (significantly influenced by the outlier of $1,000,000)
In this example, the mean ($170,000) is significantly greater than the median ($45,000) due to the presence of one extremely high salary. This high salary pulls the mean upwards, providing a misleading representation of the typical salary within the company. The median, in this case, offers a more accurate reflection of the typical employee's income.
Real-World Examples of Right-Skewed Data
Many real-world phenomena exhibit right-skewed distributions, leading to situations where the mean is greater than the median. Some examples include:
- Income Distribution: Income distributions in most countries are typically right-skewed. A small percentage of high-income earners significantly inflate the mean income, making it considerably higher than the median income.
- House Prices: Similar to income, house prices often follow a right-skewed distribution. A few extremely expensive houses can dramatically increase the mean house price while the median price remains relatively stable.
- Company Sizes: The distribution of company sizes (measured by revenue or number of employees) often exhibits a right skew. A small number of large corporations can skew the mean towards higher values compared to the median.
- Insurance Claims: The amount of insurance claims often follows a right-skewed distribution. Most claims are relatively small, but a few large claims can dramatically increase the average claim amount, resulting in a mean greater than the median.
- Wealth Distribution: The distribution of wealth across a population is often highly right-skewed, with a small percentage of individuals holding a disproportionately large share of the total wealth. This leads to a mean wealth that is considerably higher than the median wealth.
Understanding the Implications
The fact that the mean is greater than the median is a strong indicator of a right-skewed distribution. This has several important implications:
- Outliers: A significant difference between the mean and the median strongly suggests the presence of outliers or extreme values in the dataset. These outliers can disproportionately influence the mean, potentially leading to inaccurate conclusions.
- Data Interpretation: When dealing with right-skewed data, it's crucial to be cautious when interpreting the mean. The median often provides a more robust and representative measure of central tendency.
- Decision Making: Decisions based solely on the mean in right-skewed data can be flawed. For instance, using the mean income to assess the financial well-being of a population might provide a misleadingly optimistic picture.
- Statistical Modeling: In statistical modeling, the presence of right-skewness needs to be addressed appropriately. Transformations such as logarithmic transformations can be applied to make the data more normally distributed.
Beyond Simple Observations: Analyzing the Gap
While observing that the mean is greater than the median is a useful first step, understanding the magnitude of the difference is equally important. A small difference might indicate a mild skew, whereas a large difference signifies a significant skew with a substantial influence of outliers. This magnitude can be further explored using statistical measures like the coefficient of skewness.
Visualizing Skewness: Histograms and Box Plots
Visualizing data is crucial for understanding its distribution. Histograms and box plots are particularly useful for identifying skewness.
- Histograms: A histogram provides a visual representation of the data's frequency distribution. A right-skewed histogram will have a long tail extending to the right, with the majority of the data concentrated on the left.
- Box Plots: Box plots graphically depict the median, quartiles, and potential outliers. In a right-skewed distribution, the median will be closer to the lower quartile, and the right whisker will be longer than the left whisker, indicating the presence of outliers.
Using these visual tools in conjunction with the mean and median helps create a comprehensive understanding of your data's distribution and potential biases.
Conclusion: Mean vs. Median – A Critical Distinction
The relationship between the mean and the median is a fundamental concept in descriptive statistics. Understanding when the mean is greater than the median, and the implications of this relationship, is crucial for accurate data interpretation and informed decision-making. While the mean provides a valuable overall average, its susceptibility to outliers necessitates careful consideration, particularly when dealing with skewed distributions. The median offers a more robust alternative in such cases, providing a more reliable representation of the typical value. Remember always to visualize your data and consider the magnitude of the difference between the mean and the median to fully understand the nature and implications of your data's distribution. By combining these tools and approaches, you can confidently analyze your data and draw meaningful conclusions.
Latest Posts
Related Post
Thank you for visiting our website which covers about When Is Mean Greater Than Median . We hope the information provided has been useful to you. Feel free to contact us if you have any questions or need further assistance. See you next time and don't miss to bookmark.