When Is The Mean Greater Than The Median
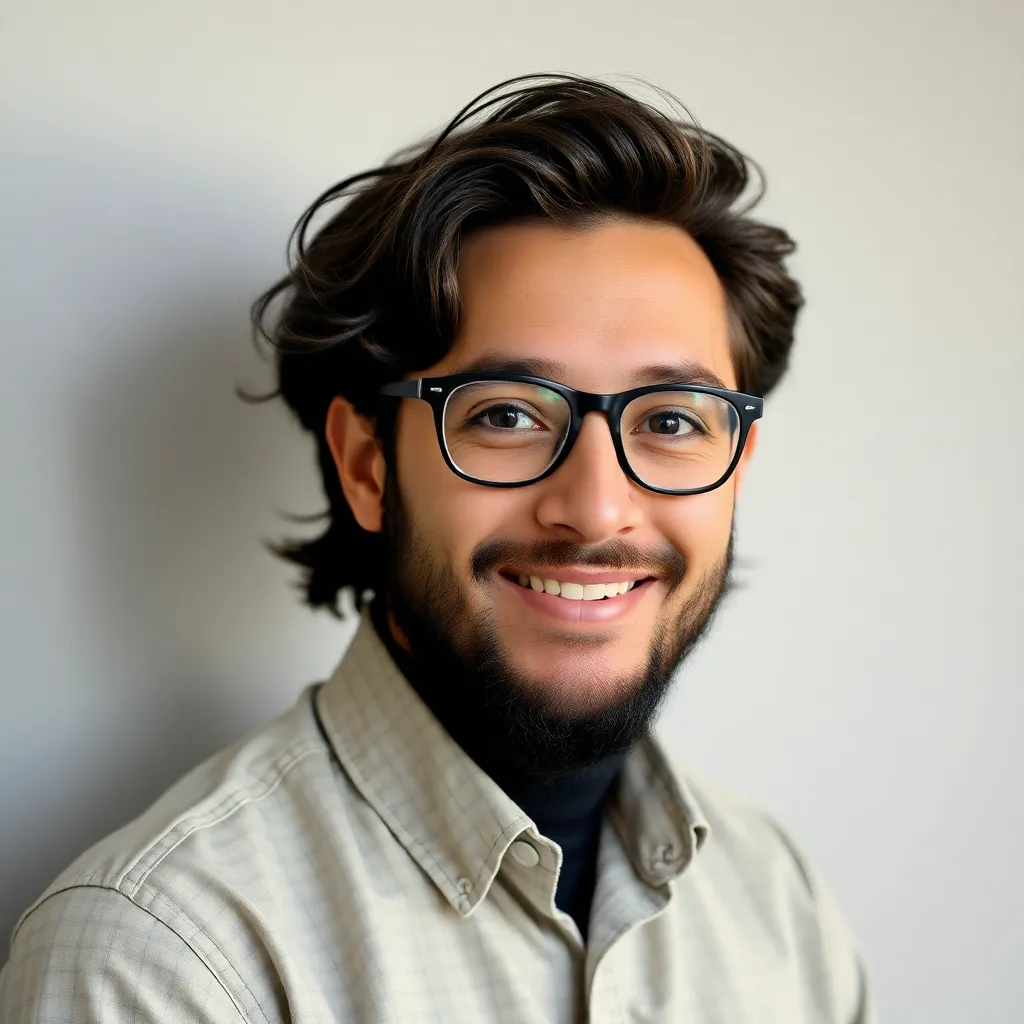
Muz Play
Apr 21, 2025 · 5 min read

Table of Contents
When is the Mean Greater Than the Median? Understanding Right-Skewed Distributions
The mean and the median are two fundamental measures of central tendency in statistics, both offering insights into the "typical" value within a dataset. While often close in value, especially in symmetrical distributions, they can diverge significantly, providing valuable clues about the underlying data's shape and distribution. Understanding when the mean is greater than the median is crucial for interpreting data correctly and making informed decisions. This article delves into this specific scenario, exploring its implications and providing practical examples.
Understanding Mean and Median
Before diving into the circumstances where the mean surpasses the median, let's quickly revisit the definitions:
-
Mean: The arithmetic average of a dataset. Calculated by summing all values and dividing by the number of values. Highly susceptible to outliers.
-
Median: The middle value in a dataset when arranged in ascending order. Unaffected by extreme values. If the dataset has an even number of values, the median is the average of the two middle values.
The Crucial Role of Skewness
The relationship between the mean and median is intrinsically linked to the skewness of a distribution. Skewness describes the asymmetry of a probability distribution. We can categorize distributions as:
-
Symmetrical: The mean and median are equal. The distribution is perfectly balanced around its center. A classic example is a normal distribution.
-
Right-Skewed (Positively Skewed): The mean is greater than the median. The tail on the right side of the distribution is longer, indicating a few high values are pulling the mean upwards.
-
Left-Skewed (Negatively Skewed): The mean is less than the median. The tail on the left side is longer, indicating a few low values are pulling the mean downwards.
When the Mean Exceeds the Median: Right-Skewed Distributions
The core answer to our question lies in right-skewed distributions. In these distributions, the presence of a few extremely high values (outliers) significantly inflates the mean, while the median, being resistant to outliers, remains relatively unaffected. This results in a mean that is substantially larger than the median.
Visualizing Right Skewness
Imagine a dataset representing the income of individuals in a particular town. Most people earn modest incomes, clustering around a lower value. However, a few exceptionally high earners (e.g., CEOs, celebrities) exist. This creates a long right tail, pulling the mean towards the higher values while the median remains representative of the typical income.
Real-World Examples of Right Skewed Data:
-
Income Distribution: As mentioned above, income distributions are often right-skewed, with a few high earners pulling up the average income.
-
House Prices: In a city, most houses might fall within a specific price range, but a small number of luxury properties drastically inflate the average house price.
-
Company Sizes: The majority of businesses might be small to medium-sized enterprises, but a few large corporations significantly inflate the average company size.
-
Insurance Claims: Most claims might be relatively small, but a few catastrophic events (large payouts) will significantly raise the mean claim amount.
-
Stock Prices: While many stocks might exhibit modest gains, a few exceptionally high-performing stocks can increase the average return.
Mathematical Explanation and Intuition
The influence of outliers on the mean is mathematically straightforward. A single extremely high value added to a dataset can substantially increase the sum used to calculate the mean, disproportionately affecting the average. The median, however, remains largely unchanged as it only considers the position of the values, not their magnitude.
Think of it like this: Imagine balancing a seesaw. The median represents the fulcrum – the point of balance. In a right-skewed distribution, the few high values are like heavy weights on one side, pulling the seesaw (the mean) far away from the fulcrum.
Consequences of Ignoring Skewness
Failing to recognize right skewness and the resulting difference between the mean and median can lead to misinterpretations and flawed conclusions. For instance:
-
Misleading Averages: Using the mean to describe the "typical" value in a right-skewed distribution can be highly misleading, presenting a falsely inflated picture.
-
Incorrect Decision Making: Decisions based on the mean, ignoring the skewness, might be biased towards the extreme values and fail to reflect the reality of the majority.
-
Inaccurate Predictions: Models relying on the mean as a central measure could produce inaccurate predictions if the skewness is not properly considered.
How to Detect Right Skewness
Identifying right skewness is crucial to avoid misinterpretations. Several methods exist:
-
Histograms: Visual inspection of a histogram can reveal the asymmetry. A right-skewed histogram will exhibit a longer tail to the right.
-
Box Plots: Box plots show the median and quartiles, providing a visual representation of the data's spread and potential skewness. A longer whisker on the right side indicates potential right skewness.
-
Skewness Coefficient: A statistical measure, the skewness coefficient, quantifies the degree of asymmetry. A positive value indicates right skewness.
-
Q-Q plots: These plots compare the quantiles of your data to the quantiles of a normal distribution. Deviations from the straight diagonal line indicate non-normality, and often skewness.
Beyond Simple Averages: Robust Alternatives
When dealing with potentially right-skewed data, relying solely on the mean can be problematic. Consider these alternatives:
-
Median: As a robust measure of central tendency, the median provides a more representative "typical" value in the presence of outliers.
-
Trimmed Mean: This involves removing a certain percentage of the highest and lowest values before calculating the mean, reducing the influence of extreme values.
-
Winsorized Mean: Similar to the trimmed mean, but instead of removing extreme values, they are replaced with less extreme values (e.g., the highest value is replaced by the highest remaining value after trimming).
Conclusion: The Significance of Context
The relationship between the mean and median offers valuable insights into the underlying data structure. While the mean is a commonly used measure, its sensitivity to outliers means it can be misleading in right-skewed distributions. Understanding when the mean is greater than the median — a clear indicator of right skewness — is crucial for accurate data interpretation, informed decision-making, and building robust statistical models. Always consider the context, utilize appropriate visualization tools, and employ robust measures of central tendency to avoid misinterpretations and make well-supported conclusions. Remember that the choice of the best measure of central tendency always depends on the specific context of the data and the research questions. Careful analysis and understanding of the data distribution are key to selecting the most appropriate statistical measure.
Latest Posts
Latest Posts
-
Find The Domain Of Composite Function
Apr 21, 2025
-
The Natural Tendency Is For Entropy To Over Time
Apr 21, 2025
-
A Distinct Group Of Organisms Is Called A
Apr 21, 2025
-
Identify The Type Of Surface Represented By The Given Equation
Apr 21, 2025
-
Which Chemical Bond Is Most Polar
Apr 21, 2025
Related Post
Thank you for visiting our website which covers about When Is The Mean Greater Than The Median . We hope the information provided has been useful to you. Feel free to contact us if you have any questions or need further assistance. See you next time and don't miss to bookmark.