When The Mean Is Greater Than The Median
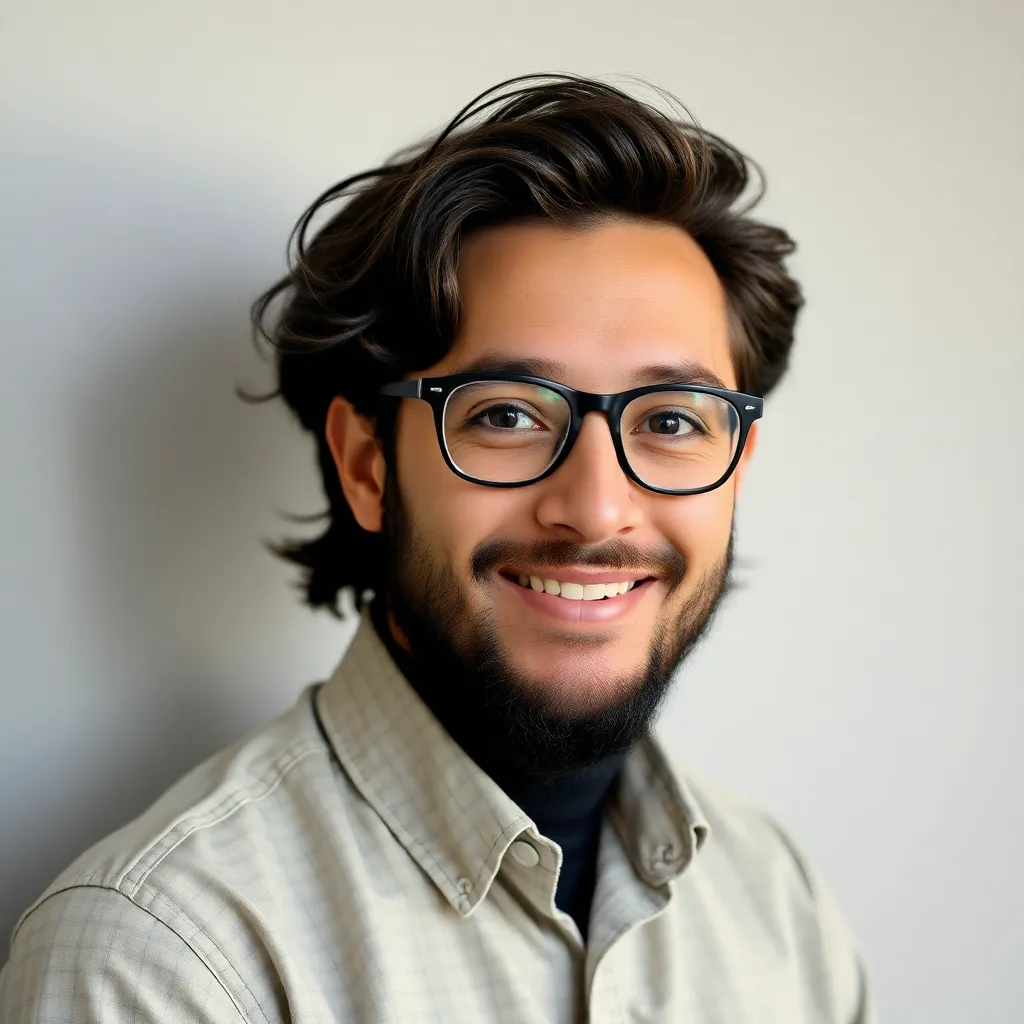
Muz Play
Apr 09, 2025 · 5 min read

Table of Contents
When the Mean is Greater Than the Median: Understanding Right-Skewed Distributions
The relationship between the mean and the median of a dataset provides valuable insights into the underlying distribution's shape. While a symmetrical distribution will have a mean and median that are equal, an asymmetrical distribution will show a difference. This article delves into the scenario where the mean is greater than the median, a characteristic of right-skewed distributions, also known as positively skewed distributions. We'll explore why this happens, the implications, and real-world examples to solidify your understanding.
Understanding Mean, Median, and Skewness
Before diving into the specifics of a right-skewed distribution, let's briefly revisit the definitions of mean, median, and skewness:
-
Mean: The average of all values in a dataset. It's calculated by summing all the values and dividing by the total number of values. Highly susceptible to outliers.
-
Median: The middle value in a dataset when it's ordered from least to greatest. It's less sensitive to outliers than the mean.
-
Skewness: A measure of the asymmetry of a probability distribution. A symmetrical distribution has zero skewness. A right-skewed distribution has positive skewness, and a left-skewed distribution has negative skewness.
Why the Mean is Greater Than the Median in Right-Skewed Distributions
In a right-skewed distribution, the tail on the right-hand side is longer and stretches further than the left-hand tail. This long right tail is often caused by the presence of a few extremely high values, often called outliers. These outliers significantly pull the mean towards the right, increasing its value compared to the median, which remains relatively unaffected by these extreme values.
Imagine a dataset representing the incomes of individuals in a specific city. A few extremely high earners (e.g., CEOs, celebrities) significantly inflate the mean income. However, the median income, representing the income of the middle individual, remains relatively unaffected by these outliers. This scenario perfectly illustrates why the mean is greater than the median in a right-skewed distribution.
Visualizing Right Skewness
Consider a simple histogram. A right-skewed histogram shows a concentration of data points towards the lower end of the range, with a long tail extending to the right. The median is located near the peak of the distribution, while the mean is pulled towards the right tail, resulting in a mean that is greater than the median.
Mathematical Explanation
The mean is influenced by the magnitude of each data point. Outliers, which are disproportionately high in right-skewed distributions, have a larger effect on the sum of the values used to calculate the mean. The median, on the other hand, only considers the position of the data points, making it less sensitive to extreme values. Therefore, the presence of outliers in a right-skewed distribution leads to a mean that is greater than the median.
Implications of a Right-Skewed Distribution
Understanding that a dataset has a right-skewed distribution has several important implications:
-
Descriptive Statistics: Using the mean as the sole measure of central tendency can be misleading in right-skewed distributions. The median provides a more robust and representative measure of the "typical" value. Reporting both the mean and the median is crucial for a complete picture.
-
Inferential Statistics: Many statistical tests assume a normal distribution (symmetrical). Right-skewed data may violate these assumptions, potentially leading to inaccurate conclusions. Transformations, such as logarithmic or square root transformations, can sometimes help normalize the data.
-
Data Interpretation: Understanding the skewness helps in interpreting the data correctly. A right-skewed distribution may indicate the presence of influential factors or underlying processes that lead to a few exceptionally high values. Investigating these factors can provide crucial insights.
Real-World Examples of Right-Skewed Distributions
Right-skewed distributions are prevalent in numerous real-world scenarios:
-
Income Distribution: As previously mentioned, income data typically shows a right skew, with a small percentage of high earners significantly influencing the mean income.
-
House Prices: In a particular area, a few luxury homes can drastically increase the average house price, while the median price remains relatively stable.
-
Company Sizes: The number of employees in companies often follows a right-skewed distribution. Most companies are small to medium-sized, while a few large corporations dominate the upper end.
-
Insurance Claims: Most insurance claims are relatively small, but a few large claims (catastrophic events) heavily influence the average claim size.
-
Wealth Distribution: The distribution of wealth is often heavily right-skewed, with a small percentage of the population holding a disproportionately large share of the total wealth.
-
Website Traffic: Many websites have a small number of highly popular pages that receive a disproportionate amount of traffic, resulting in a right-skewed distribution of page views.
Dealing with Right-Skewed Data
When analyzing right-skewed data, several strategies can be employed:
-
Report Both Mean and Median: Always report both the mean and the median to give a complete picture of the central tendency. The difference between them highlights the degree of skewness.
-
Use the Median as the Primary Measure: In many situations, the median is a more appropriate and robust measure of central tendency for right-skewed data.
-
Data Transformation: Transforming the data using a logarithmic or square root transformation can sometimes make the distribution more symmetrical and suitable for standard statistical methods.
-
Non-parametric Methods: Consider using non-parametric statistical methods that don't assume a normal distribution. These methods are often more robust to outliers and skewness.
-
Explore the Outliers: Investigate the reasons behind the outliers. They might represent valuable insights or errors in the data collection process.
Conclusion: Understanding the Significance of Skewness
The relationship between the mean and median provides a valuable indicator of the shape of a distribution. When the mean is greater than the median, it's a strong signal of a right-skewed distribution. Understanding this relationship is crucial for correctly interpreting data, choosing appropriate statistical methods, and drawing meaningful conclusions. By carefully considering the implications of skewness and employing suitable analytical techniques, you can gain a more accurate and nuanced understanding of your data. Remember to always consider both the mean and median, alongside visual representations like histograms, to fully grasp the characteristics of your dataset. Ignoring the presence of right skewness can lead to misinterpretations and flawed conclusions in your analysis. Careful consideration of these factors ensures a more robust and insightful understanding of your data.
Latest Posts
Latest Posts
-
Examples Of Working Backwards Problem Solving
Apr 17, 2025
-
Concentrated Solution Of A Strong Acid
Apr 17, 2025
-
Fatty Acid Synthesis Vs Beta Oxidation
Apr 17, 2025
-
Biological Views Of Psychological Disorders Fall Into Three Main Categories
Apr 17, 2025
-
Isotopes Of An Element Have The Same Number Of
Apr 17, 2025
Related Post
Thank you for visiting our website which covers about When The Mean Is Greater Than The Median . We hope the information provided has been useful to you. Feel free to contact us if you have any questions or need further assistance. See you next time and don't miss to bookmark.