Which Measure Of Center Best Represents The Data
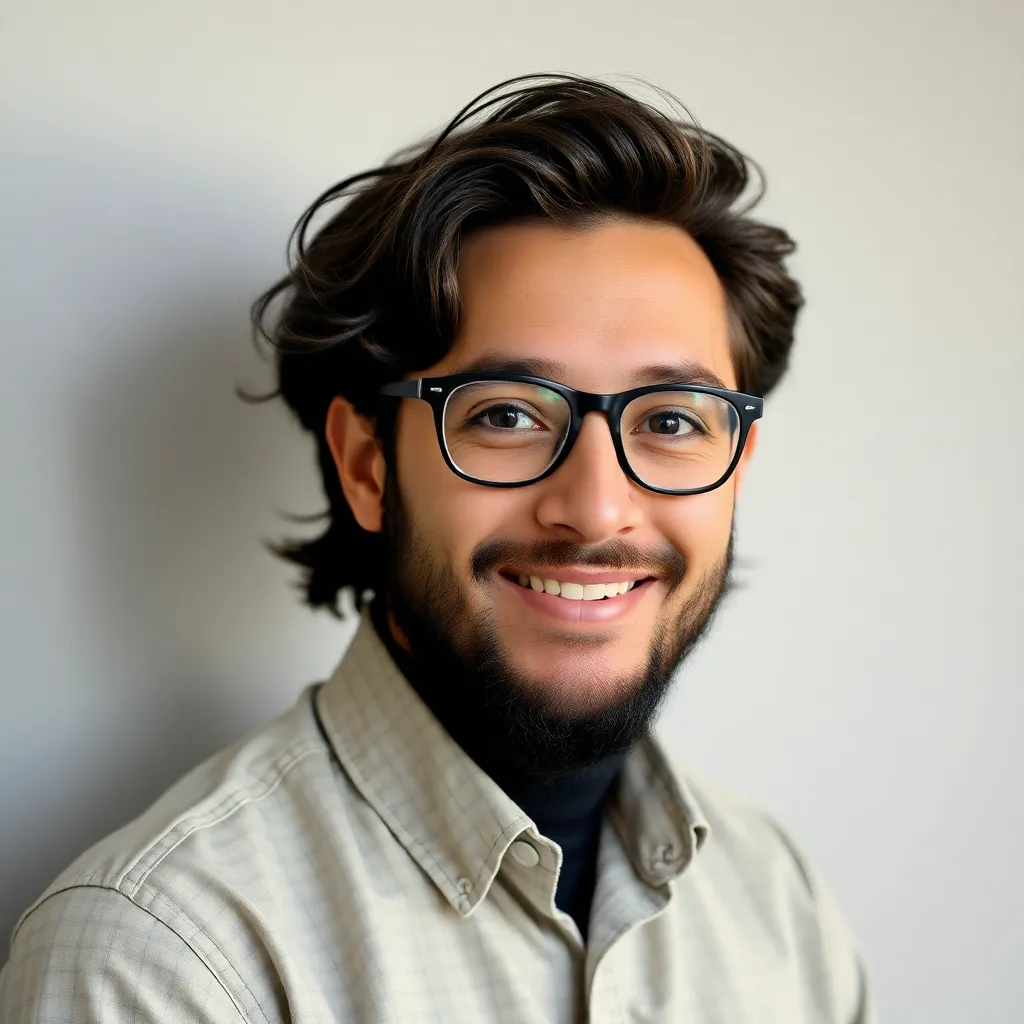
Muz Play
Apr 10, 2025 · 6 min read

Table of Contents
Which Measure of Center Best Represents the Data? A Deep Dive into Mean, Median, and Mode
Choosing the right measure of center is crucial for accurately representing and interpreting data. The three most common measures – mean, median, and mode – each offer unique insights, but their appropriateness depends heavily on the characteristics of your dataset and the specific question you're trying to answer. This comprehensive guide will explore each measure, highlight their strengths and weaknesses, and guide you in selecting the most suitable option for your data analysis.
Understanding the Measures of Central Tendency
Before delving into the specifics, let's define our key terms:
-
Mean: The arithmetic average, calculated by summing all data points and dividing by the total number of data points. It's highly sensitive to outliers.
-
Median: The middle value in a dataset when it's ordered from least to greatest. If the dataset has an even number of data points, the median is the average of the two middle values. It's less sensitive to outliers than the mean.
-
Mode: The value that appears most frequently in a dataset. A dataset can have multiple modes (bimodal, multimodal) or no mode at all. It's useful for categorical data and identifying the most common value.
Mean: The Average We All Know
The mean, often simply called the "average," is the most familiar measure of central tendency. It's straightforward to calculate and provides a single value representing the typical value in the dataset.
Strengths of the Mean:
- Simplicity: Easy to calculate and understand.
- Widely Used: A standard measure used across various fields.
- Mathematical Properties: Useful for further statistical calculations and inferences. It's the basis for many other statistical measures.
Weaknesses of the Mean:
- Susceptibility to Outliers: Extreme values (outliers) can significantly skew the mean, providing a misleading representation of the central tendency. Consider a dataset of salaries: a few extremely high salaries can inflate the mean, making it seem like the average salary is much higher than it actually is for most employees.
- Inappropriate for Skewed Data: In skewed distributions (where data is clustered more towards one end), the mean might not accurately reflect the "typical" value. For example, income data often exhibits right skewness (a long tail to the right), where the mean is pulled upward by a few high earners.
When to Use the Mean:
The mean is best suited for datasets that:
- Are normally distributed (or approximately so).
- Have few or no outliers.
- Are measured on an interval or ratio scale (scales with meaningful numerical values).
Median: The Middle Ground
The median represents the middle value of a dataset when arranged in ascending order. Its resilience to outliers makes it a valuable alternative to the mean in many situations.
Strengths of the Median:
- Robustness to Outliers: Unaffected by extreme values, offering a more stable representation of central tendency in the presence of outliers.
- Suitable for Skewed Data: Provides a more accurate representation of the typical value in skewed distributions compared to the mean.
- Easy to Understand: Intuitive concept easily grasped by non-statisticians.
Weaknesses of the Median:
- Less Informative: Doesn't utilize all data points in the calculation, potentially losing some information.
- Less Useful for Further Calculations: Compared to the mean, it's less suitable for advanced statistical analyses.
- Ambiguous with Even Number of Data Points: Requires averaging the two middle values, adding a small degree of complexity.
When to Use the Median:
The median is preferable when:
- The dataset contains outliers.
- The distribution is skewed.
- The data is ordinal (ranked) or has a mixture of different data types.
Mode: The Most Frequent Value
The mode identifies the most frequently occurring value in a dataset. It's particularly useful for categorical data, but also finds application in numerical datasets.
Strengths of the Mode:
- Applicable to Categorical Data: Unlike the mean and median, the mode can be used with categorical data (e.g., colors, brands, types).
- Simple to Identify: Easy to determine even without complex calculations.
- Identifies Clusters: Highlights the most common values, revealing potential clusters within the data.
Weaknesses of the Mode:
- Multiple Modes: A dataset might have multiple modes (bimodal, multimodal), making interpretation challenging.
- No Mode Possible: A dataset might have no mode if all values occur with equal frequency.
- Not Sensitive to Changes in Distribution: Small changes in the data might not affect the mode, potentially masking important trends.
When to Use the Mode:
The mode is most appropriate for:
- Categorical data.
- Identifying the most popular or frequent item.
- Datasets with multiple modes that represent distinct sub-groups within the data.
Choosing the Best Measure: A Practical Guide
Selecting the appropriate measure of central tendency hinges on understanding the nature of your data and your analytical goals. Here’s a step-by-step guide:
-
Examine Your Data: Check for outliers, skewness, and the data type (categorical, numerical). Create histograms or box plots to visualize the distribution.
-
Consider the Research Question: What are you trying to learn from the data? Do you need a measure that’s robust to outliers? Are you interested in the most frequent value?
-
Apply the Appropriate Measure:
- Symmetrical Data with No Outliers: Use the mean.
- Skewed Data or Data with Outliers: Use the median.
- Categorical Data: Use the mode.
- Mixed Data: The median might be a suitable compromise.
Case Studies: Real-World Applications
Let's illustrate the choice of measure with real-world examples:
Case Study 1: House Prices
Analyzing house prices in a neighborhood, you'll likely find outliers (e.g., a mansion significantly more expensive than other houses). The median price would provide a more accurate representation of the "typical" house price compared to the mean, which would be inflated by the outliers.
Case Study 2: Customer Satisfaction
Measuring customer satisfaction scores (rated on a scale of 1 to 5), the mean could be used if the distribution is fairly symmetrical. However, if the distribution is skewed, the median would offer a more robust measure of central tendency. The mode could also be useful to identify the most frequent satisfaction level.
Case Study 3: Favorite Colors
In a survey asking participants about their favorite colors, the mode is the only applicable measure, as the data is categorical. The mean and median are meaningless in this context.
Beyond the Basics: Considering Other Factors
While mean, median, and mode are the primary measures, other factors influence the choice:
- Sample Size: With small sample sizes, the reliability of any measure of central tendency is reduced.
- Data Distribution: The shape of the distribution significantly impacts the choice of measure.
- Purpose of Analysis: The specific goals of the analysis should guide the selection of the most informative measure.
Conclusion: Informed Decision-Making
Choosing the best measure of center requires careful consideration of your data's characteristics and your analytical objectives. Understanding the strengths and weaknesses of the mean, median, and mode empowers you to make an informed decision, ensuring your analysis accurately reflects the central tendency of your data and supports sound conclusions. Remember that visualizing your data through histograms, box plots, or scatter plots can be instrumental in guiding your choice and revealing hidden patterns. By applying these insights, you can enhance the clarity and accuracy of your data analysis and draw more meaningful conclusions from your findings.
Latest Posts
Latest Posts
-
What Is The Oxidation State Of Iron
Apr 18, 2025
-
What Is Open Sentence In Mathematics
Apr 18, 2025
-
Cation And Anion Held Together By Electrostatic Forces
Apr 18, 2025
-
The Metals In Groups 1a 2a And 3a
Apr 18, 2025
-
Is A Fat Or Phospholipid Less Soluble In Water
Apr 18, 2025
Related Post
Thank you for visiting our website which covers about Which Measure Of Center Best Represents The Data . We hope the information provided has been useful to you. Feel free to contact us if you have any questions or need further assistance. See you next time and don't miss to bookmark.