Which Statistic Is The Best Unbiased Estimator For Mu
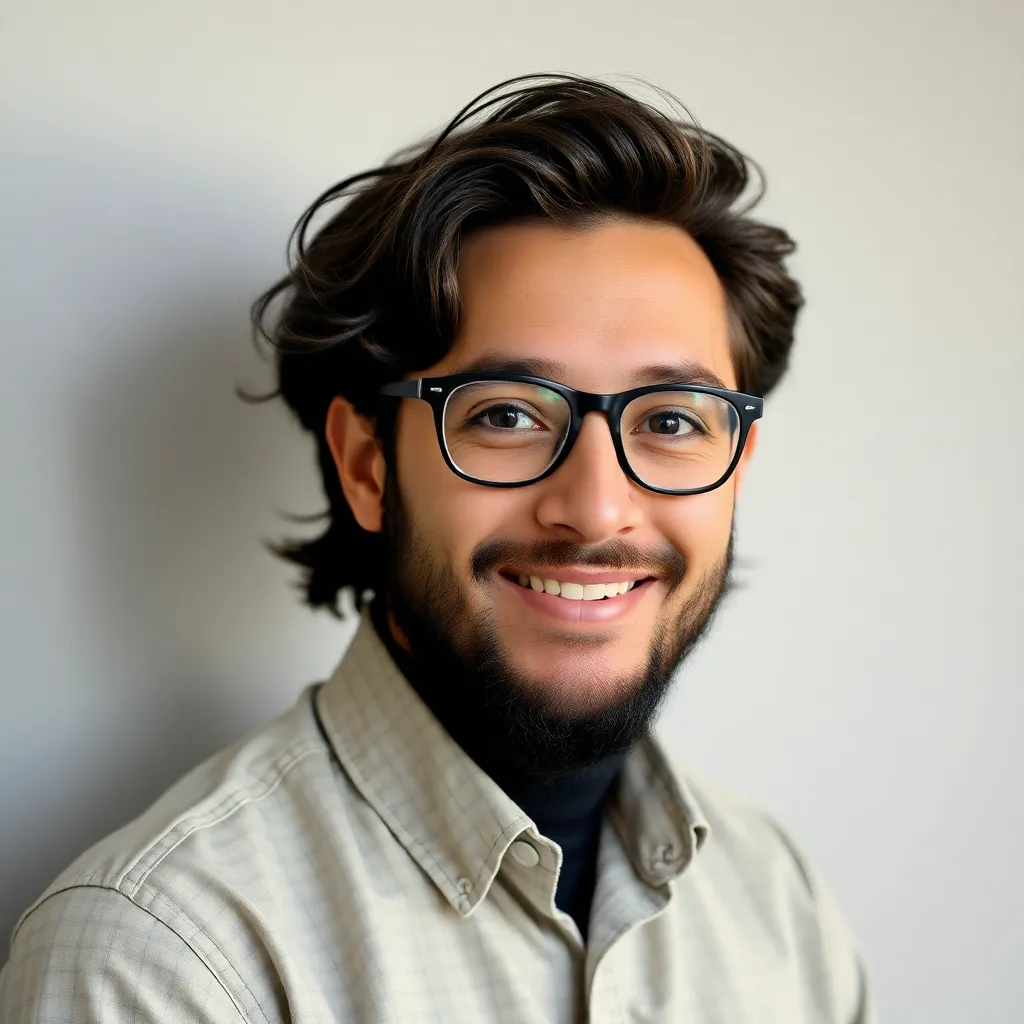
Muz Play
May 09, 2025 · 6 min read
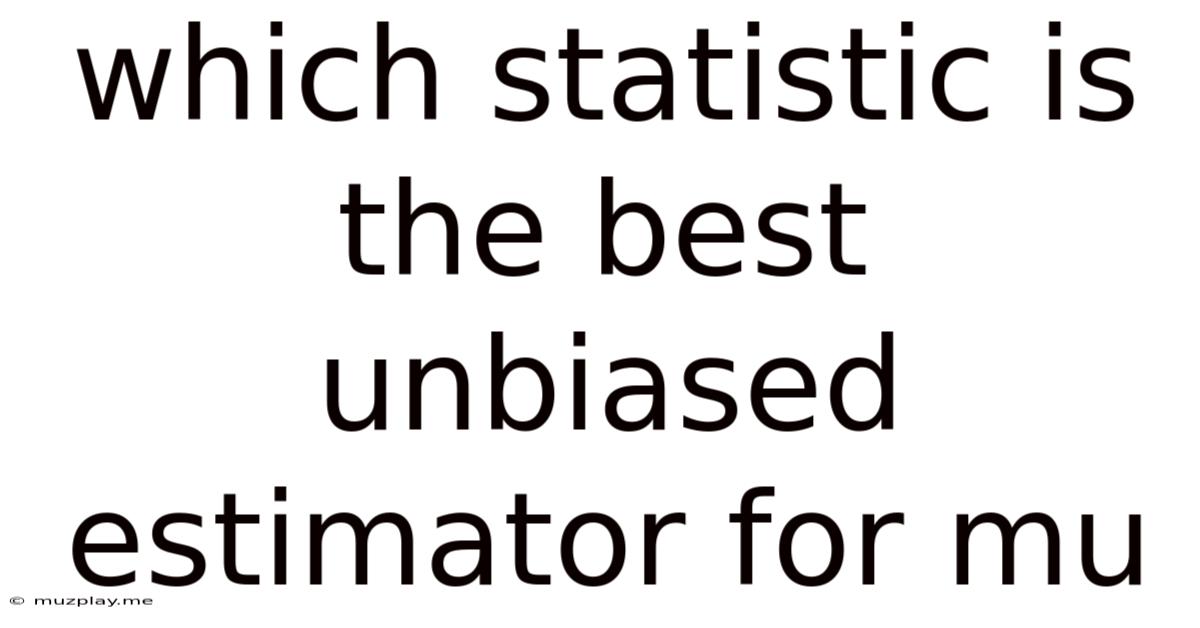
Table of Contents
Which Statistic is the Best Unbiased Estimator for μ? A Deep Dive into Statistical Inference
The question of which statistic serves as the best unbiased estimator for the population mean (μ) is a cornerstone of statistical inference. While the sample mean (x̄) is frequently used and often considered the "best," a nuanced understanding requires exploring the underlying assumptions, potential biases, and the broader context of the problem. This article delves into this crucial topic, comparing the sample mean with other estimators and examining scenarios where alternative approaches might prove superior.
Understanding Unbiased Estimators and the Population Mean (μ)
Before diving into specific estimators, let's clarify some fundamental concepts.
-
Population Mean (μ): This represents the true average of a characteristic within an entire population. It's often unknown and what we aim to estimate.
-
Sample Mean (x̄): Calculated as the sum of observations in a sample divided by the sample size (n), it's a common point estimate for μ.
-
Unbiased Estimator: An estimator is unbiased if its expected value is equal to the true population parameter. In simpler terms, if we were to repeatedly sample from the population and calculate the estimator each time, the average of these estimations would converge to the true value. Bias indicates a systematic overestimation or underestimation of the parameter.
-
Efficiency: An efficient estimator has a smaller variance than other unbiased estimators. A smaller variance means the estimator's values are clustered more tightly around the true value, providing greater precision.
The Sample Mean (x̄): The Usual Suspect
The sample mean, x̄, is frequently the first choice for estimating the population mean. Its popularity stems from several key properties:
-
Unbiasedness: Under simple random sampling, the sample mean is an unbiased estimator of the population mean. This means E(x̄) = μ.
-
Consistency: As the sample size (n) increases, the sample mean converges to the population mean. This means larger samples provide more accurate estimations.
-
Relative Simplicity: Calculating the sample mean is straightforward and computationally inexpensive.
Limitations of the Sample Mean
Despite its desirable properties, the sample mean isn't universally the best choice. Its effectiveness depends on several factors:
-
Sample Size: With small sample sizes, the sample mean can be quite variable, leading to less precise estimations. Larger samples are generally preferred.
-
Distribution of the Population: The sample mean's performance is highly dependent on the underlying distribution of the population. If the population is heavily skewed or has outliers, the sample mean can be significantly influenced by these extreme values, potentially making it a less reliable estimate.
-
Presence of Outliers: Outliers disproportionately affect the sample mean, pulling it away from the true population mean. Robust estimators, discussed below, are less sensitive to outliers.
Alternatives to the Sample Mean: Exploring Robust Estimators
When dealing with skewed distributions or the presence of outliers, robust estimators offer a more reliable alternative to the sample mean. These estimators are less susceptible to the influence of extreme values.
The Median
The median is the middle value in a dataset when it's ordered. It's a robust measure of central tendency, less sensitive to outliers than the mean. While the median is not an unbiased estimator of μ in all cases, its robustness makes it a preferable choice in certain situations. Specifically:
-
Skewed Distributions: In skewed distributions, the median provides a more representative measure of central tendency than the mean, which is easily pulled towards the tail.
-
Outlier Presence: The median is unaffected by outliers, making it a more stable estimator when dealing with contaminated data.
However, the median's efficiency is generally lower than that of the mean, meaning its variance is higher for the same sample size.
Trimmed Mean
The trimmed mean involves removing a certain percentage of the highest and lowest values from the dataset before calculating the mean. This mitigates the influence of outliers while retaining more information than the median. The percentage trimmed depends on the dataset and the suspected level of contamination. A 10% trimmed mean, for example, removes the top and bottom 10% of values. Like the median, it's more robust than the sample mean.
Winsorized Mean
Similar to the trimmed mean, the Winsorized mean replaces the extreme values with less extreme values (often the highest and lowest values that remain after trimming) before calculating the mean. This approach retains more information from the extremes than trimming but still reduces their impact.
M-Estimators
M-estimators represent a broad class of robust estimators that minimize a particular function of the data. These functions are designed to be less sensitive to outliers than the squared error function used in calculating the mean. Examples include Huber loss and Tukey's biweight function. These M-estimators often offer a good balance between robustness and efficiency.
Choosing the Best Estimator: Context Matters
The "best" unbiased estimator for μ depends heavily on the specific context:
-
Normally Distributed Data: If the data is normally distributed or approximately so, the sample mean is generally the most efficient and unbiased estimator.
-
Skewed or Contaminated Data: For skewed data or data with outliers, robust estimators like the median, trimmed mean, Winsorized mean, or M-estimators are preferred. The optimal choice among these robust estimators often depends on the degree of skewness and the number of outliers.
-
Large Sample Size: With large sample sizes, the influence of outliers is often diminished, and the sample mean becomes a more reliable estimator even in non-normal distributions due to the Central Limit Theorem.
-
Computational Resources: The sample mean is computationally simplest. More complex robust estimators require more computational power.
Beyond Point Estimation: Interval Estimation and Confidence Intervals
While point estimators provide a single value estimate of μ, interval estimation provides a range of values within which the true mean is likely to lie. Confidence intervals are crucial for understanding the uncertainty associated with our estimations. The sample mean forms the basis of many commonly used confidence intervals, particularly when assuming normality. However, for non-normal distributions or the presence of outliers, bootstrapping methods can be used to construct more robust confidence intervals that are not reliant on specific distributional assumptions.
Conclusion: A Pragmatic Approach
There's no single "best" unbiased estimator for μ applicable in all situations. The optimal choice depends critically on the characteristics of the data, the sample size, the computational resources available, and the desired level of robustness. The sample mean remains a valuable and often efficient estimator, especially for large samples and normally distributed data. However, when faced with non-normality, outliers, or a need for robust results, employing alternative estimators like the median, trimmed mean, Winsorized mean, or M-estimators should be considered. A thorough understanding of the data and careful consideration of the potential biases are vital for selecting the most appropriate estimator and drawing meaningful conclusions. Remember to always assess your data's characteristics before choosing a method, and consider the implications of your chosen estimator on your interpretation of results. By combining statistical knowledge with practical considerations, you can ensure the accuracy and reliability of your estimations.
Latest Posts
Latest Posts
-
Atoms Combine In Simple Whole Number Ratios To Form Compounds
May 10, 2025
-
What Happens To Electrons In Any Charging Process
May 10, 2025
-
Is Salt A Mixture Or A Compound
May 10, 2025
-
Carbohydrates Are Composed Of What Elements
May 10, 2025
-
Is The Derivative Of Velocity Acceleration
May 10, 2025
Related Post
Thank you for visiting our website which covers about Which Statistic Is The Best Unbiased Estimator For Mu . We hope the information provided has been useful to you. Feel free to contact us if you have any questions or need further assistance. See you next time and don't miss to bookmark.