Why Is A Hypothesis Considered A Model
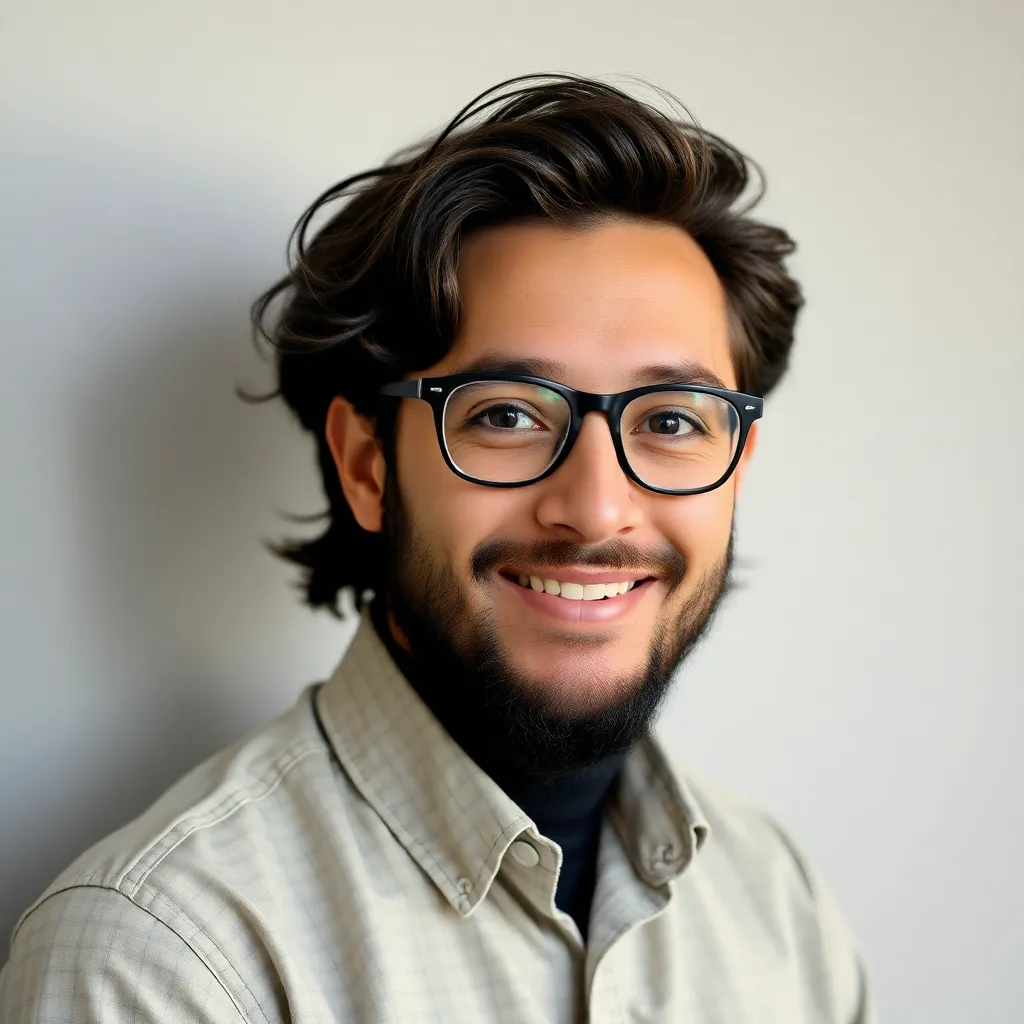
Muz Play
May 10, 2025 · 6 min read
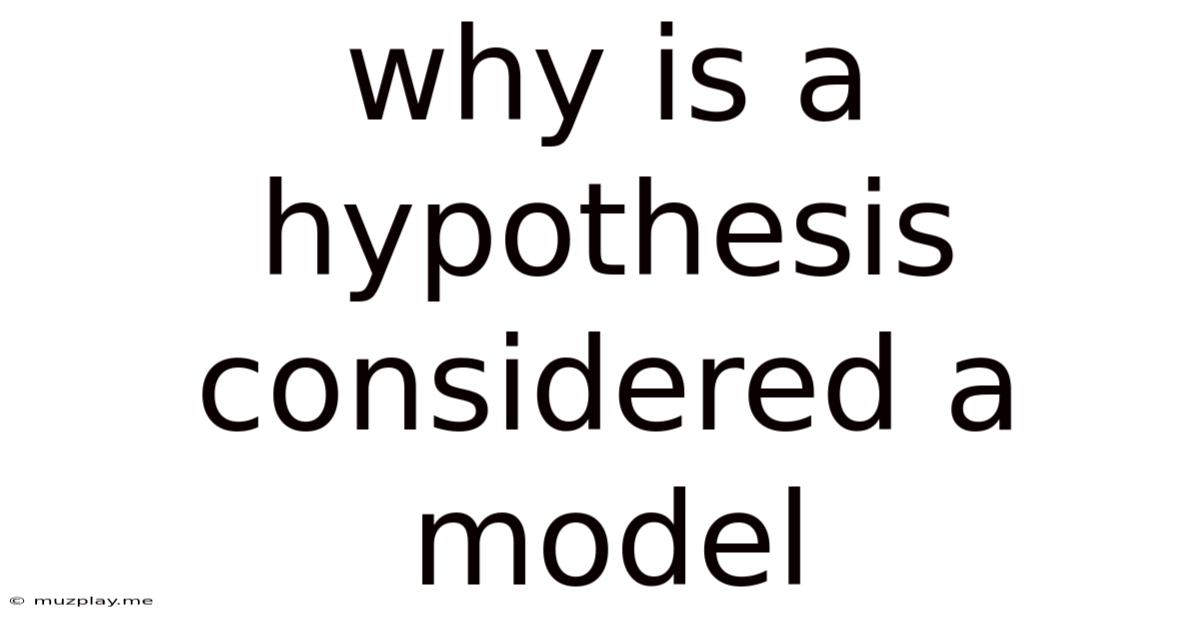
Table of Contents
Why is a Hypothesis Considered a Model? Unpacking the Relationship Between Hypotheses and Models in Scientific Inquiry
A hypothesis, at its core, is a proposed explanation for an observable phenomenon. But what often goes unnoticed is its implicit function as a model. This article delves deep into the nuanced relationship between hypotheses and models, clarifying why considering a hypothesis as a model offers a powerful framework for understanding scientific inquiry and the advancement of knowledge. We will explore the different types of models, the role of simplification and abstraction in model building, and how hypotheses function as testable, simplified representations of reality.
The Nature of Scientific Models: Abstractions and Simplifications
Before dissecting the hypothesis-model connection, it's crucial to understand the essence of scientific models. A scientific model is not a literal replica of the real world. Instead, it's a simplified representation designed to capture the essential features of a system or phenomenon while omitting unnecessary details. This simplification is vital because complex systems often contain a bewildering array of variables and interactions. Models allow us to focus on the most critical aspects, making them manageable and easier to study.
Models come in various forms, including:
Types of Scientific Models:
- Physical Models: These are tangible representations, like a miniature replica of the solar system or a scale model of a building.
- Conceptual Models: These are abstract representations, often expressed verbally or visually (e.g., diagrams, flowcharts), explaining relationships and processes.
- Mathematical Models: These use mathematical equations to describe relationships and predict outcomes. Many models combine elements from different categories.
The key characteristic uniting all models is their inherent abstraction. They select specific aspects of reality, neglecting others. This isn't a flaw but a necessity. Perfect replication is typically impossible and often impractical. The effectiveness of a model hinges on its ability to capture the relevant features while ignoring the irrelevant ones. The choice of which aspects to include or exclude is a critical step in the modeling process and demonstrates the inherent subjectivity within scientific endeavors. This subjectivity is often tempered by rigorous testing and refinement.
Hypotheses as Testable Models: A Deeper Dive
Now, let's connect the dots. A hypothesis, in its essence, is a type of conceptual model. It provides a simplified representation of how a specific aspect of the world works. Consider the following example:
Hypothesis: Increased exposure to sunlight leads to increased plant growth.
This hypothesis isn't a perfect description of plant growth. It omits factors like soil quality, water availability, and the specific plant species. However, it isolates a single variable (sunlight exposure) and proposes a relationship with another (plant growth). This simplification makes it testable.
We can design an experiment to test this hypothesis by exposing different groups of plants to varying amounts of sunlight, controlling other factors as much as possible. The results of the experiment will either support or refute the hypothesis, refining our understanding of the relationship between sunlight and plant growth. The hypothesis acted as a simplified model, guiding the research and offering a framework for interpretation.
Characteristics of Hypotheses as Models:
- Predictive Power: A strong hypothesis generates testable predictions. If the hypothesis is correct, we should observe specific outcomes under specific conditions.
- Falsifiability: A crucial feature of a good scientific hypothesis is its falsifiability. It must be possible to design an experiment that could potentially disprove the hypothesis. This contrasts with non-falsifiable statements, like "God exists," which are outside the realm of scientific inquiry.
- Refined through Testing: Hypotheses are not static. They are continually refined and improved based on experimental results. A hypothesis supported by repeated testing gains credibility, but it never becomes absolute truth. Scientific knowledge is always provisional, open to revision in light of new evidence. Negative results are equally valuable, guiding the modification or abandonment of a hypothesis.
The Iterative Nature of Hypothesis Testing and Model Refinement: A Cycle of Learning
The process of hypothesis testing and model refinement is iterative. It involves a continuous cycle of:
- Observation: Noticing a phenomenon that needs explanation.
- Hypothesis Formulation: Proposing a simplified explanation (model) for the phenomenon.
- Prediction: Formulating testable predictions based on the hypothesis.
- Experimentation: Designing and conducting experiments to test the predictions.
- Analysis: Analyzing the experimental results to evaluate whether the data support or refute the hypothesis.
- Model Revision: Modifying or abandoning the hypothesis based on the analysis. This often involves developing a new, more nuanced hypothesis or model. This process continues iteratively, with each cycle leading to a better understanding of the phenomenon.
This process is not linear. Scientists often revise their hypotheses even before experimental results are available, leading to a more refined prediction before starting the testing phase. The feedback loop between the hypothesis (model), prediction, experiment, and analysis is crucial for scientific progress. The hypothesis is consistently adjusted to better fit reality.
Beyond Simple Hypotheses: Complex Models and Interacting Variables
While the "sunlight and plant growth" example highlights the basic relationship between hypotheses and models, scientific inquiry often involves far more complex models. Real-world phenomena rarely involve only one variable. Consider a hypothesis about climate change:
Hypothesis: Increased greenhouse gas emissions contribute significantly to global warming.
This hypothesis is a model that simplifies a tremendously complex system involving atmospheric physics, ocean currents, land use changes, and biological interactions. It incorporates numerous variables and acknowledges their interactions. Yet, it remains a model, focusing on the crucial relationship between greenhouse gases and global temperature, abstracting away countless other details. Testing such a hypothesis requires sophisticated modeling techniques and large-scale data analysis.
The complexity of the model increases, but the underlying principles remain the same. The hypothesis provides a simplified, testable representation of a complex reality, guiding research and offering a framework for interpreting vast amounts of data. The model's success lies not in its perfection but in its ability to capture the essential features of the system, allowing us to understand, predict, and potentially mitigate the effects of climate change.
The Role of Assumptions and Limitations in Hypothesis-as-Model
It's important to acknowledge that all models, including those represented by hypotheses, are built on assumptions. These assumptions are simplifications or generalizations about the system being studied. For example, the "sunlight and plant growth" hypothesis implicitly assumes other factors remain relatively constant. The acknowledgment of these assumptions is crucial because they define the limitations of the model.
It's not uncommon for scientists to engage in model selection, comparing the performance of different models, each built upon different assumptions. Ultimately, they choose the model that is best suited for a given purpose and context, which highlights the iterative and multifaceted nature of model-building in scientific inquiry.
Conclusion: Embracing the Power of Simplification in Scientific Inquiry
In conclusion, understanding the hypothesis-as-model framework is essential for grasping the nature of scientific progress. Hypotheses are not merely educated guesses; they are simplified, testable models that guide research, organize data, and advance our understanding of the world. The inherent simplification and abstraction inherent in models are not weaknesses, but rather the tools that enable us to grapple with the complexity of nature. By acknowledging the assumptions and limitations of our hypotheses, we can build more robust models and contribute to the iterative cycle of scientific discovery, progressively refining our understanding of the world around us. The iterative process of testing, revising, and refining hypotheses, which are inherently models, is central to the growth and progression of all scientific fields.
Latest Posts
Latest Posts
-
Random Number Between 1 And 43
May 10, 2025
-
Collection Of Neuron Cell Bodies Outside The Cns
May 10, 2025
-
Nursing Care Plan For Patient With Paranoid Schizophrenia
May 10, 2025
-
An Atom That Has Lost An Electron
May 10, 2025
-
Which Phase Of The Cell Cycle Is The Longest
May 10, 2025
Related Post
Thank you for visiting our website which covers about Why Is A Hypothesis Considered A Model . We hope the information provided has been useful to you. Feel free to contact us if you have any questions or need further assistance. See you next time and don't miss to bookmark.