Why Is Mol The Abbreviation To Mle
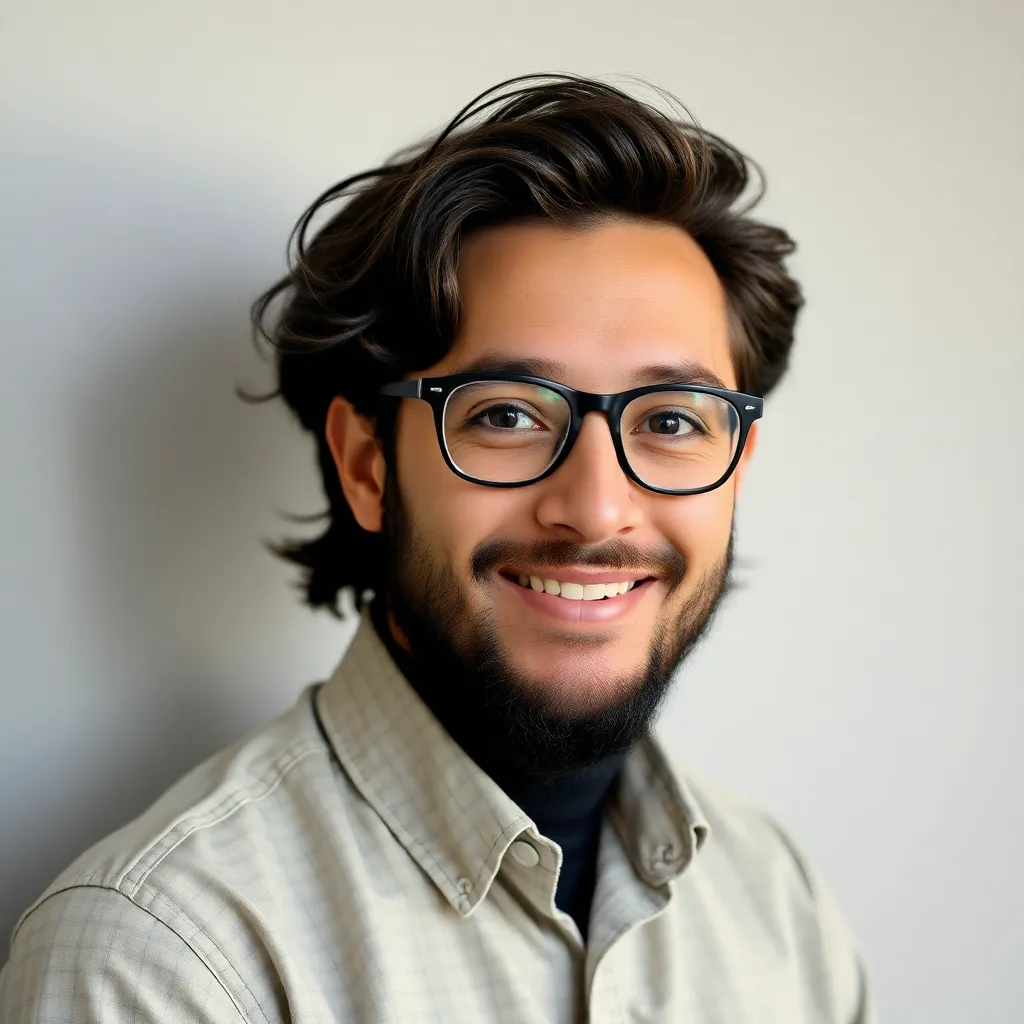
Muz Play
Mar 31, 2025 · 5 min read
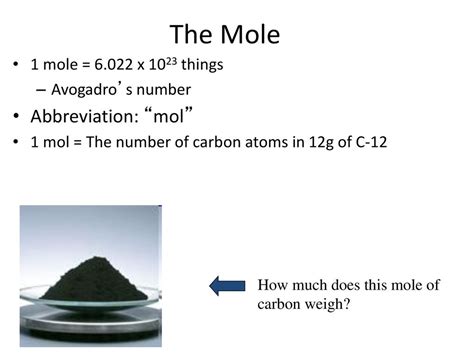
Table of Contents
Why is MOL the Abbreviation for MLE? Unraveling the Mystery Behind Maximum Likelihood Estimation
The abbreviation MOL for Maximum Likelihood Estimation (MLE) isn't standard or widely accepted within the statistical or machine learning communities. The common and universally understood abbreviation is MLE. There's no established or documented use of "MOL" as a substitute. Therefore, this article will explore the potential reasons why someone might mistakenly use "MOL" instead of "MLE," examining the context in which such a misattribution might arise, and clarifying the core concepts of Maximum Likelihood Estimation itself.
While we won't find a definitive answer to the question of why someone would use "MOL" for MLE, exploring the surrounding topics will offer a comprehensive understanding of the method and its importance.
Understanding Maximum Likelihood Estimation (MLE)
Maximum Likelihood Estimation is a fundamental statistical method used to estimate the parameters of a probability distribution given some observed data. In simpler terms, it helps us figure out the most likely values of unknown parameters based on the data we have. Imagine you have a coin, and you want to know if it's fair (50% chance of heads, 50% chance of tails). You flip it 10 times, and you get 7 heads. MLE would help you estimate the probability of getting heads based on this data.
The Core Idea: Maximizing the Likelihood
The heart of MLE lies in the likelihood function. This function describes the probability of observing the data given specific values of the parameters. The goal of MLE is to find the parameter values that maximize this likelihood function. In essence, we're looking for the parameter values that make the observed data most probable.
Example: Let's say we're modeling the height of students in a class using a normal distribution. The normal distribution has two parameters: the mean (average height) and the standard deviation (spread of heights). The likelihood function would tell us the probability of observing the heights of all students in the class, given different values of the mean and standard deviation. MLE would find the mean and standard deviation that make this probability the highest.
Why MLE is Widely Used
MLE enjoys widespread use due to several attractive properties:
- Intuitive and relatively easy to understand: The core concept of finding the most likely parameters is conceptually straightforward.
- Consistent estimators: As the amount of data increases, the MLE estimates tend to converge towards the true parameter values.
- Asymptotically efficient: Under certain conditions, MLE provides the most efficient estimators among all consistent estimators.
- Wide applicability: MLE can be applied to a vast range of probability distributions and data types.
Applications of MLE
MLE finds applications in numerous fields, including:
- Machine learning: Estimating parameters in various models like linear regression, logistic regression, and neural networks.
- Statistics: Estimating parameters in different probability distributions, such as normal, binomial, Poisson, etc.
- Image processing: Estimating parameters in image models for tasks like denoising and segmentation.
- Bioinformatics: Estimating parameters in models of biological sequences.
- Finance: Estimating parameters in financial models for risk management and asset pricing.
Potential Reasons for the "MOL" Misattribution
Given the absence of any established usage of "MOL" for MLE, its appearance likely stems from one of the following:
- Typographical Error: The most straightforward explanation is a simple typing mistake. "MOL" and "MLE" are visually similar, and a quick typo could easily lead to the incorrect abbreviation.
- Misunderstanding or Neologism: Someone might have coined "MOL" as a shorthand within a very specific, niche context, unaware that "MLE" is the standard abbreviation. This is highly unlikely given the widespread use of MLE.
- Informal Notation: In informal settings or casual conversations, abbreviations might be slightly altered or personalized. However, this doesn't translate to formal or published work.
- Regional Variation (Highly Unlikely): While regional variations in terminology exist, they usually involve different terms altogether, not just a minor letter change in an established abbreviation.
Distinguishing MLE from Other Estimation Methods
It's important to differentiate MLE from other statistical estimation methods, such as:
- Method of Moments (MOM): MOM equates sample moments (e.g., mean, variance) with population moments to estimate parameters. While simpler than MLE, it's often less efficient.
- Bayesian Estimation: Bayesian estimation incorporates prior knowledge about the parameters into the estimation process, updating beliefs as new data becomes available. MLE, on the other hand, is frequentist and doesn't utilize prior knowledge.
Advanced Concepts in MLE
Understanding MLE fully involves delving into some more advanced statistical concepts:
- Likelihood Function and its properties: A deep dive into the mathematical definition and behavior of the likelihood function.
- Maximum Likelihood Estimators (MLEs): The properties of MLEs, including consistency, efficiency, and asymptotic normality.
- Optimization Algorithms: The methods used to find the parameter values that maximize the likelihood function, such as gradient ascent, Newton-Raphson, and Expectation-Maximization (EM) algorithm.
- Bias and Variance in MLE: Understanding and managing bias and variance in MLE estimates.
- Dealing with Overfitting: Strategies to prevent overfitting in models estimated using MLE.
Conclusion: Stick with MLE
In conclusion, the use of "MOL" as an abbreviation for Maximum Likelihood Estimation (MLE) is not a standard or accepted practice within the statistical or machine learning community. "MLE" remains the universally recognized and preferred abbreviation. If you encounter "MOL" in any context, it's highly probable that it's a typographical error, a localized and informal usage, or a misunderstanding. Always prioritize using the correct and widely understood abbreviation, "MLE," to ensure clear and effective communication within the field. Understanding the nuances of MLE, its advantages, and its relation to other estimation methods is crucial for any serious student or practitioner of statistics or machine learning. This article aims to provide a comprehensive and accessible overview of this powerful and widely used technique.
Latest Posts
Latest Posts
-
Law Of Sines Worksheet And Answers
Apr 01, 2025
-
Northern Blot Southern Blot Western Blot
Apr 01, 2025
-
Map Of N Africa And Sw Asia
Apr 01, 2025
-
Converting Water From A Liquid To A Gas Requires
Apr 01, 2025
-
Comparison Of Somatic And Autonomic Nervous Systems
Apr 01, 2025
Related Post
Thank you for visiting our website which covers about Why Is Mol The Abbreviation To Mle . We hope the information provided has been useful to you. Feel free to contact us if you have any questions or need further assistance. See you next time and don't miss to bookmark.