B. The Symbol For Population Standard Deviation Is
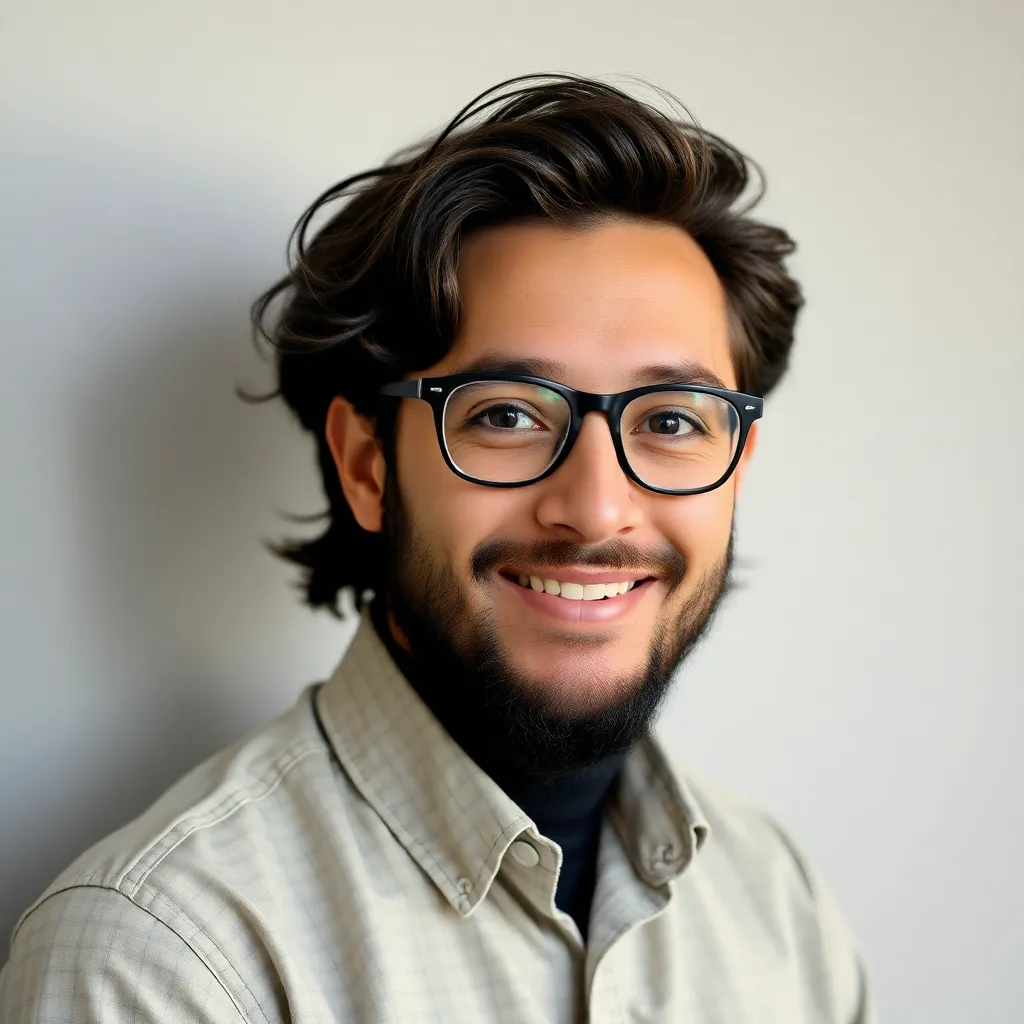
Muz Play
May 12, 2025 · 5 min read
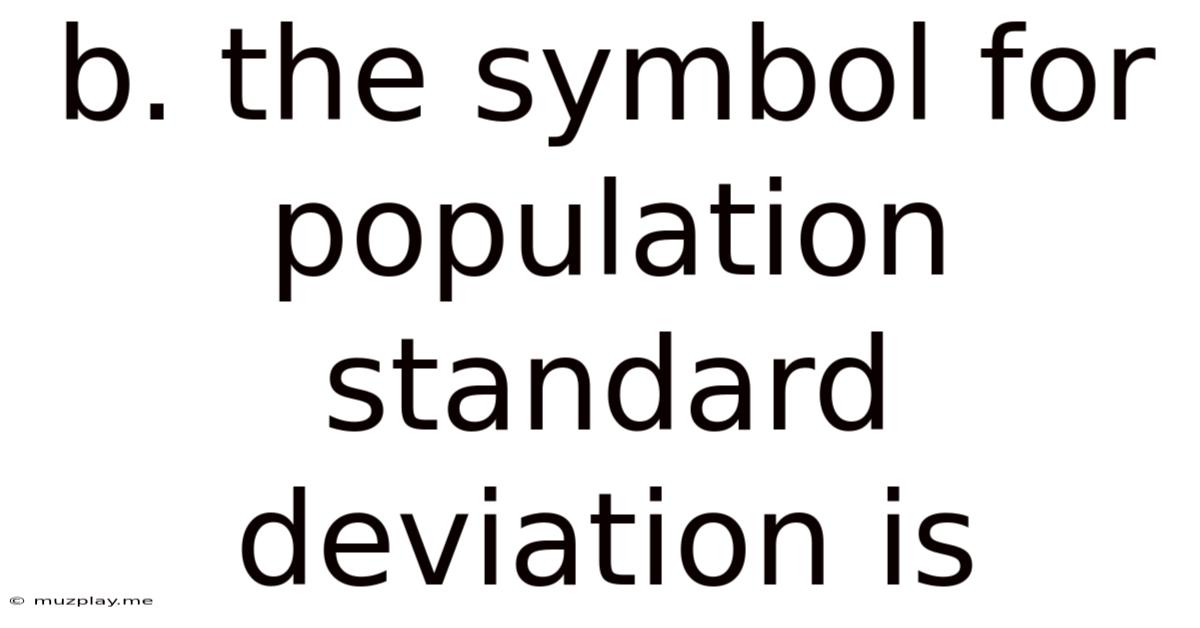
Table of Contents
The Symbol for Population Standard Deviation: A Deep Dive into Statistical Significance
Understanding statistical concepts is crucial for analyzing data effectively, and the population standard deviation is a cornerstone of descriptive statistics. This comprehensive guide will delve into the symbol for population standard deviation, its meaning, calculation, significance, and its relationship to other statistical measures. We'll also explore practical applications and common misconceptions surrounding this important concept.
Understanding the Symbol: σ (Sigma)
The symbol universally used to represent the population standard deviation is the lowercase Greek letter σ (sigma). Why sigma? Because in statistics, sigma often signifies summation or total. While it doesn't directly represent the sum in the standard deviation formula, it's a convenient and widely accepted convention, readily recognized within the statistical community. Using a Greek letter also helps distinguish between population parameters (like σ) and sample statistics (like s, discussed later).
What is Population Standard Deviation?
The population standard deviation (σ) measures the spread or dispersion of a dataset representing the entire population. Unlike a sample, a population includes every single member of the group being studied. This is a crucial distinction because the methods for calculating standard deviation differ slightly depending on whether you're working with the entire population or a subset (a sample).
Imagine you're studying the heights of all the adult women in a specific town. That's your population. The population standard deviation tells you how much the individual heights vary from the population mean (average) height. A large σ indicates high variability (heights are widely spread), while a small σ indicates low variability (heights are clustered closely around the mean).
Key Differences Between Population and Sample Standard Deviation
It's vital to differentiate between population standard deviation (σ) and sample standard deviation (s). While both measure dispersion, they differ in their calculation and interpretation:
- Population Standard Deviation (σ): Calculated using data from the entire population. It's a parameter – a fixed value that describes the population.
- Sample Standard Deviation (s): Calculated using data from a subset of the population (a sample). It's a statistic – an estimate of the population standard deviation. The formula for s includes a denominator of n-1 (where n is the sample size), rather than n, to provide an unbiased estimate of the population standard deviation. This adjustment is known as Bessel's correction.
Calculating the Population Standard Deviation (σ)
The formula for calculating the population standard deviation is:
σ = √[Σ(xi - μ)² / N]
Where:
- σ: Population standard deviation
- Σ: Summation (add up all the values)
- xi: Each individual data point in the population
- μ: The population mean (average)
- N: The total number of data points in the population
Let's break it down step-by-step:
-
Calculate the population mean (μ): Sum all the data points (xi) and divide by the total number of data points (N).
-
Calculate the deviations from the mean: Subtract the mean (μ) from each data point (xi). These are the deviations.
-
Square the deviations: Square each of the deviations calculated in step 2. This removes negative signs and emphasizes larger deviations.
-
Sum the squared deviations: Add up all the squared deviations.
-
Divide by N: Divide the sum of squared deviations by the total number of data points (N). This is the population variance (σ²).
-
Take the square root: Take the square root of the population variance to obtain the population standard deviation (σ).
Significance and Interpretation of σ
The population standard deviation's significance lies in its ability to:
-
Quantify Variability: It provides a numerical measure of how spread out the data is. A higher σ indicates greater variability, meaning the data points are more dispersed from the mean. A lower σ indicates less variability, meaning the data points are clustered closer to the mean.
-
Compare Datasets: You can use σ to compare the variability of different populations. For example, you could compare the variability of heights in two different cities.
-
Make Statistical Inferences: While often used in descriptive statistics, σ is a fundamental parameter in many inferential statistical tests. It plays a critical role in hypothesis testing, confidence intervals, and other statistical analyses that draw conclusions about a population based on a sample.
-
Assess Data Normality: While not a direct measure of normality, the standard deviation, in conjunction with the mean, helps visualize the distribution of data and allows for assessment of whether the data appears approximately normally distributed.
Applications of Population Standard Deviation
The population standard deviation finds extensive applications in numerous fields:
-
Finance: Assessing the risk associated with investments. A higher standard deviation in stock returns indicates higher volatility and risk.
-
Healthcare: Measuring the variability of patient outcomes, helping to identify factors influencing treatment effectiveness.
-
Manufacturing: Controlling the quality of products by monitoring the variation in production processes. Consistent product quality relies on low standard deviation in critical measurements.
-
Environmental Science: Analyzing the variability of environmental parameters like temperature, rainfall, or pollution levels.
-
Social Sciences: Studying the variability of opinions or behaviors within a population.
Common Misconceptions about σ
-
Confusing σ and s: Failing to distinguish between population and sample standard deviation leads to incorrect calculations and interpretations.
-
Interpreting σ without context: The numerical value of σ is meaningless without considering the units of measurement and the nature of the data.
-
Assuming normality: Many statistical tests assume data is normally distributed. While σ doesn't directly measure normality, examining its value alongside other descriptive statistics can provide clues about the data's distribution.
Conclusion: The Power of σ
The symbol σ (sigma) for population standard deviation is more than just a Greek letter; it represents a powerful tool for understanding data variability. While its calculation can appear complex, understanding its meaning and significance is crucial for accurate data analysis and interpretation. Remembering the distinction between σ and s and avoiding common misconceptions will lead to a clearer understanding of data dispersion and pave the way for more effective statistical analysis across various fields. Mastering this fundamental statistical concept empowers data scientists, researchers, and anyone working with data to draw meaningful conclusions and make informed decisions.
Latest Posts
Latest Posts
-
Equal Sharing Of Electrons Between Atoms
May 12, 2025
-
Are Lysosomes In Eukaryotes Or Prokaryotes
May 12, 2025
-
How Did Thomas Malthus Influence Darwin
May 12, 2025
-
What Is An Example Of Possibilism
May 12, 2025
-
Two Sample T Test With Equal Variances
May 12, 2025
Related Post
Thank you for visiting our website which covers about B. The Symbol For Population Standard Deviation Is . We hope the information provided has been useful to you. Feel free to contact us if you have any questions or need further assistance. See you next time and don't miss to bookmark.