Two Sample T Test With Equal Variances
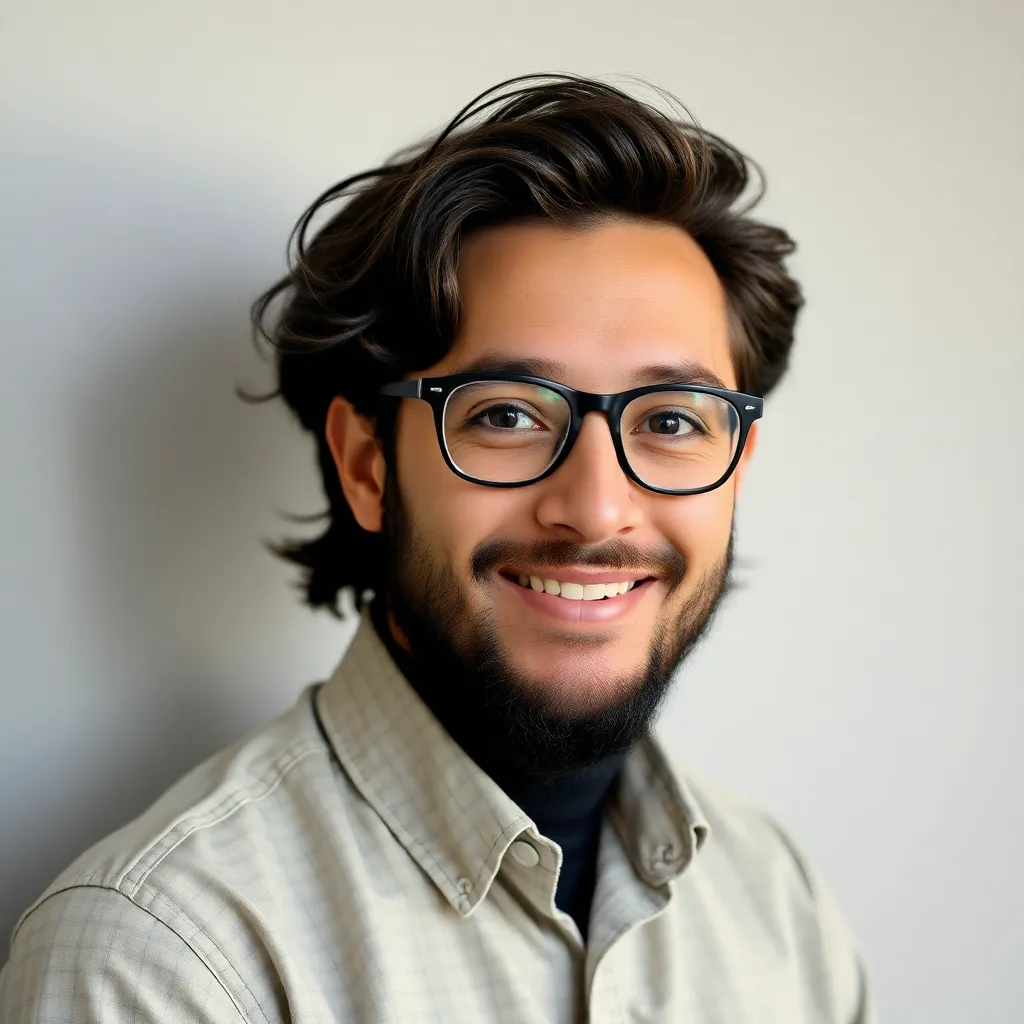
Muz Play
May 12, 2025 · 6 min read

Table of Contents
Two-Sample t-Test with Equal Variances: A Comprehensive Guide
The two-sample t-test is a fundamental statistical test used to compare the means of two independent groups. This article delves deep into the two-sample t-test assuming equal variances, explaining its underlying assumptions, the step-by-step calculation process, interpretation of results, and common applications. We’ll also explore its limitations and potential alternatives.
Understanding the Two-Sample t-Test with Equal Variances
The two-sample t-test with equal variances, also known as the pooled t-test, is employed when we want to determine if there's a statistically significant difference between the means of two independent groups, assuming that the population variances of these two groups are equal. This assumption of equal variances is crucial; if violated, a different type of t-test (the Welch's t-test) should be used.
This test is particularly useful in scenarios where you are comparing:
- Treatment and control groups: Evaluating the effectiveness of a new drug by comparing the mean improvement in a treatment group against a control group receiving a placebo.
- Different populations: Comparing the average income of males and females in a particular region.
- Experimental conditions: Assessing the performance of two different teaching methods by comparing the average test scores of students in each group.
Key Assumption: The fundamental assumption of this test is that the population variances of the two groups are equal (homoscedasticity). Before performing the test, you should check this assumption using tests like Levene's test or Bartlett's test. If the assumption of equal variances is violated, the results of the pooled t-test become unreliable.
Step-by-Step Calculation of the Two-Sample t-Test with Equal Variances
Let's walk through the process of conducting a two-sample t-test with equal variances, using the following notation:
- n₁: Sample size of group 1
- n₂: Sample size of group 2
- x̄₁: Sample mean of group 1
- x̄₂: Sample mean of group 2
- s₁²: Sample variance of group 1
- s₂²: Sample variance of group 2
1. Calculate the Pooled Variance:
The pooled variance (s<sub>p</sub>²) is an estimate of the common population variance, combining information from both samples. The formula is:
s<sub>p</sub>² = [(n₁ - 1)s₁² + (n₂ - 1)s₂²] / (n₁ + n₂ - 2)
2. Calculate the Standard Error of the Difference Between Means:
The standard error measures the variability of the difference between the sample means. The formula is:
SE = √[s<sub>p</sub>²(1/n₁ + 1/n₂)]
3. Calculate the t-Statistic:
The t-statistic measures the difference between the sample means relative to the standard error. The formula is:
t = (x̄₁ - x̄₂) / SE
4. Determine the Degrees of Freedom:
The degrees of freedom (df) indicate the number of independent pieces of information available to estimate the population parameters. For the pooled t-test:
df = n₁ + n₂ - 2
5. Determine the p-value:
The p-value is the probability of observing a t-statistic as extreme as the one calculated, assuming the null hypothesis (no difference between population means) is true. You can find the p-value using a t-distribution table or statistical software. The p-value is compared to a pre-determined significance level (alpha), typically set at 0.05.
6. Make a Decision:
- If the p-value ≤ alpha: Reject the null hypothesis. There is statistically significant evidence to suggest a difference between the population means of the two groups.
- If the p-value > alpha: Fail to reject the null hypothesis. There is not enough evidence to suggest a difference between the population means.
Example Calculation
Let's consider an example. Suppose we want to compare the average heights of male and female students. We have the following data:
- Males (Group 1): n₁ = 25, x̄₁ = 175 cm, s₁² = 25 cm²
- Females (Group 2): n₂ = 30, x̄₂ = 165 cm, s₂² = 20 cm²
1. Pooled Variance:
s<sub>p</sub>² = [(25 - 1) * 25 + (30 - 1) * 20] / (25 + 30 - 2) = 22.22 cm²
2. Standard Error:
SE = √[22.22 * (1/25 + 1/30)] = 1.24 cm
3. t-Statistic:
t = (175 - 165) / 1.24 = 8.06
4. Degrees of Freedom:
df = 25 + 30 - 2 = 53
5. p-value:
Using a t-distribution table or statistical software, we find that the p-value associated with a t-statistic of 8.06 and 53 degrees of freedom is extremely small (much less than 0.001).
6. Decision:
Since the p-value is much less than 0.05, we reject the null hypothesis. We conclude that there is a statistically significant difference in the average heights of male and female students.
Interpreting the Results
The p-value is the key to interpreting the results. A small p-value (typically less than 0.05) indicates strong evidence against the null hypothesis, suggesting a statistically significant difference between the means. However, statistical significance doesn't necessarily imply practical significance. The magnitude of the difference between the means should also be considered in the context of the research question. A small difference might be statistically significant with a large sample size, but it might not be practically meaningful.
Limitations and Alternatives
The two-sample t-test with equal variances has several limitations:
- Assumption of Normality: The test assumes that the data within each group are approximately normally distributed. Violations of this assumption can affect the validity of the results, especially with small sample sizes. Transformations or non-parametric alternatives might be necessary.
- Assumption of Independence: Observations within each group must be independent of each other. If observations are correlated, the test results will be unreliable.
- Assumption of Equal Variances: As already stressed, this is a critical assumption. If the variances are significantly different, using the Welch's t-test, which doesn't assume equal variances, is more appropriate.
Alternatives to the pooled t-test include:
- Welch's t-test: This test does not assume equal variances and is more robust to violations of this assumption.
- Mann-Whitney U test: This is a non-parametric test, meaning it does not assume normality. It compares the ranks of the data in the two groups. It's useful when the data are not normally distributed or when the assumptions of the t-test are violated.
Conclusion
The two-sample t-test with equal variances is a powerful tool for comparing the means of two independent groups when the assumption of equal variances holds. However, it's crucial to carefully check the assumptions before conducting the test and consider alternative tests if the assumptions are violated. Always remember to interpret the results in the context of the research question and consider both statistical and practical significance. Understanding the limitations and alternatives will help you choose the most appropriate statistical test for your data. Remember to always consult with a statistician if you have complex research questions or uncertainties about data analysis. This detailed guide provides a solid foundation for understanding and applying this crucial statistical test. By carefully considering the assumptions, calculations, and interpretations, you can draw accurate and reliable conclusions from your data analysis.
Latest Posts
Latest Posts
-
How To Do Bohr Rutherford Diagrams
May 12, 2025
-
Is Milk Pure Substance Or Mixture
May 12, 2025
-
Power Series Of 1 1 X
May 12, 2025
-
Is Boron Trifluoride Polar Or Nonpolar
May 12, 2025
-
Which Point Of The Beam Experiences The Most Compression
May 12, 2025
Related Post
Thank you for visiting our website which covers about Two Sample T Test With Equal Variances . We hope the information provided has been useful to you. Feel free to contact us if you have any questions or need further assistance. See you next time and don't miss to bookmark.