Critical Value Of Chi Square Table
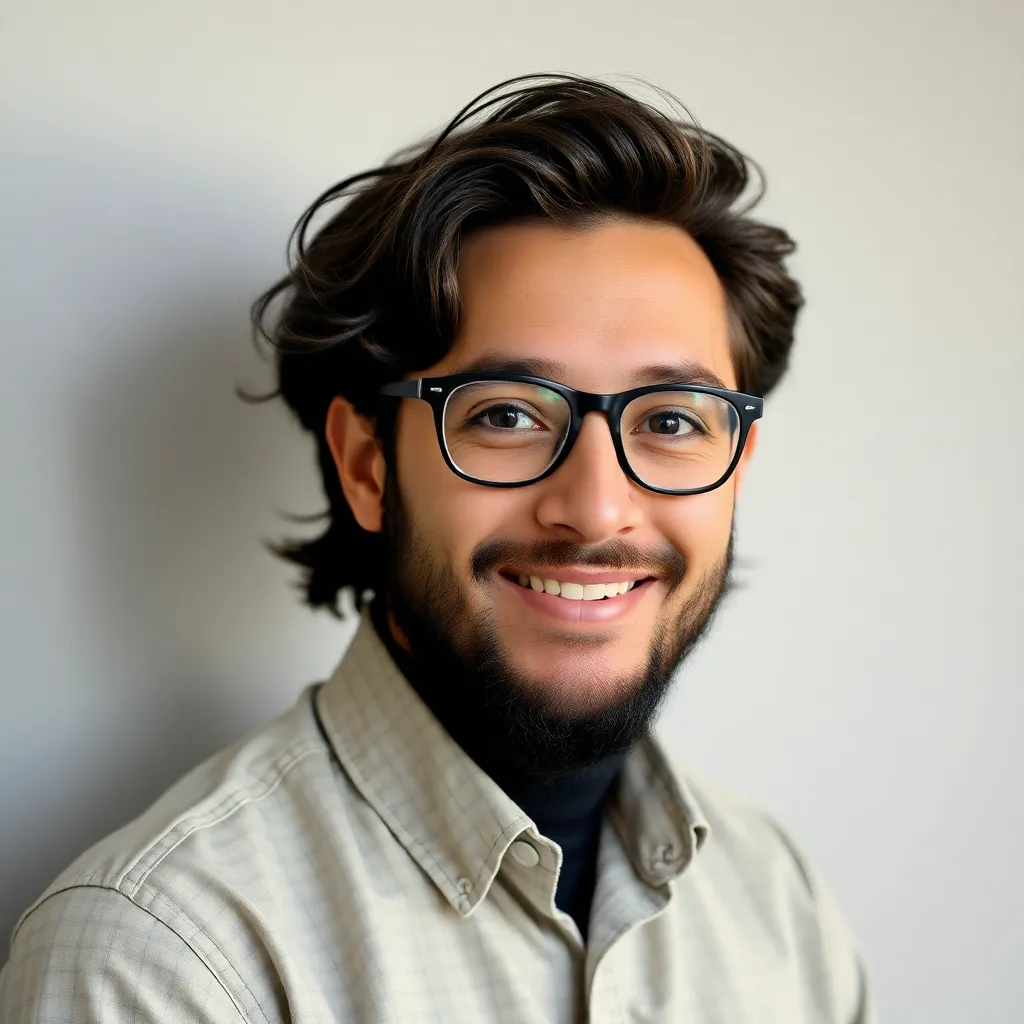
Muz Play
May 12, 2025 · 6 min read
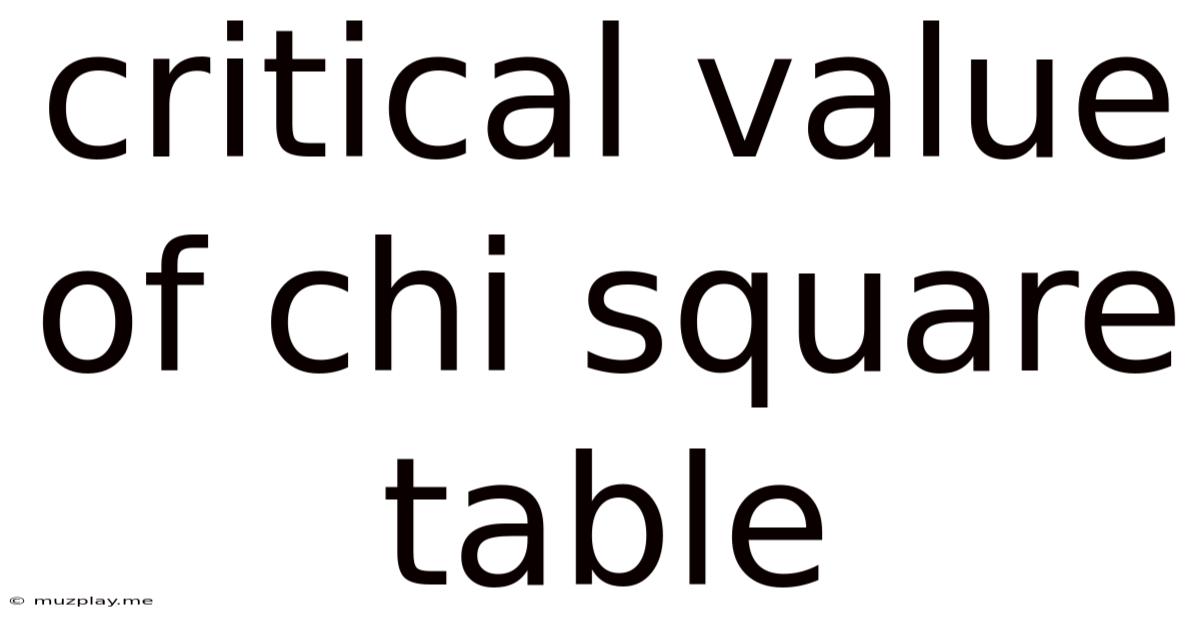
Table of Contents
The Critical Value of the Chi-Square Table: A Comprehensive Guide
The chi-square (χ²) distribution is a crucial tool in statistical analysis, widely used to test hypotheses about categorical data. Understanding the chi-square table and its critical values is fundamental to correctly interpreting the results of these tests. This comprehensive guide will delve into the intricacies of the chi-square table, exploring its applications, interpretation, and practical implications.
Understanding the Chi-Square Distribution
Before diving into the critical values, let's establish a foundational understanding of the chi-square distribution itself. It's a probability distribution that arises frequently in statistical hypothesis testing. Unlike the normal distribution which is symmetrical, the chi-square distribution is asymmetrical, skewed to the right. This means that the tail on the right-hand side extends further than the tail on the left. The shape of the chi-square distribution is determined by its degrees of freedom (df). The degrees of freedom represent the number of independent pieces of information available to estimate a parameter.
The higher the degrees of freedom, the more the chi-square distribution resembles a normal distribution. This is because as the degrees of freedom increase, the skewness diminishes. This characteristic is important when considering the critical values, as their position along the distribution changes with the degrees of freedom.
What are Critical Values?
Critical values are the threshold values that define the region of rejection in a hypothesis test. In essence, they act as boundaries separating the region where the null hypothesis is likely to be true from the region where it's likely to be false. If the calculated chi-square statistic from your test falls within the rejection region (i.e., exceeds the critical value), you reject the null hypothesis. Conversely, if the calculated statistic falls outside the rejection region, you fail to reject the null hypothesis. This decision is made at a predetermined significance level (alpha), typically 0.05 (5%).
The significance level (α) represents the probability of rejecting the null hypothesis when it is actually true (a Type I error). A lower significance level reduces the chance of a Type I error but increases the chance of a Type II error (failing to reject a false null hypothesis). The choice of significance level depends on the context of the study and the consequences of each type of error.
Locating Critical Values on the Chi-Square Table
The chi-square table is a readily available resource that provides critical values for different degrees of freedom and significance levels. The table is structured with rows representing degrees of freedom and columns representing significance levels (α). The intersection of a specific row and column gives the corresponding critical value.
For instance, if you have a chi-square test with 5 degrees of freedom and a significance level of 0.05, you would locate the row corresponding to df = 5 and the column corresponding to α = 0.05. The value at their intersection is the critical value for your test.
Important Note: Many chi-square tables only provide critical values for the right tail of the distribution (one-tailed test). For two-tailed tests, you need to adjust the significance level. For a two-tailed test with a significance level of 0.05, you would consult the table for α = 0.025 (0.05/2) in each tail.
Applications of Chi-Square Tests and Critical Values
The chi-square test has diverse applications across various fields, including:
1. Goodness-of-Fit Test:
This test assesses whether a sample distribution matches a hypothesized distribution. For example, you might use a goodness-of-fit test to see if the distribution of colors in a bag of candies matches the manufacturer's stated proportions. The critical value helps determine whether the observed deviations from the expected distribution are statistically significant.
2. Test of Independence:
This test examines the relationship between two categorical variables. It determines whether the variables are independent or if there's an association between them. For instance, you could use this test to see if there is a relationship between smoking habits and lung cancer. Again, the critical value helps you decide if the observed association is statistically significant.
3. Test of Homogeneity:
This test compares the distribution of a single categorical variable across different populations or groups. For example, you could compare the distribution of political affiliations among different age groups. The critical value plays the same role as in the previous tests – determining if observed differences are statistically significant.
Interpreting Results and Making Decisions
Once you've calculated the chi-square statistic from your data and found the critical value from the table, you can make a decision about your hypothesis:
-
If the calculated chi-square statistic is greater than the critical value: You reject the null hypothesis. This indicates that the observed results are unlikely to have occurred by chance alone and there is evidence to support the alternative hypothesis.
-
If the calculated chi-square statistic is less than or equal to the critical value: You fail to reject the null hypothesis. This means there is not enough evidence to reject the null hypothesis based on the data. However, this does not necessarily mean the null hypothesis is true; it simply means that the evidence is insufficient to reject it.
Beyond the Table: Software and Calculations
While the chi-square table is a valuable tool, statistical software packages like R, SPSS, SAS, and Python (with libraries like SciPy) can perform chi-square tests and provide precise p-values. The p-value represents the probability of obtaining results as extreme as, or more extreme than, the observed results if the null hypothesis is true. If the p-value is less than your chosen significance level (e.g., 0.05), you reject the null hypothesis. Software is particularly useful for more complex scenarios or large datasets.
Assumptions of the Chi-Square Test
It's crucial to remember that the chi-square test relies on certain assumptions:
- Independence of observations: Each observation should be independent of the others.
- Expected cell frequencies: The expected frequency in each cell of the contingency table should be sufficiently large (generally, at least 5). If expected frequencies are too low, the chi-square approximation may not be accurate, and alternative methods might be necessary (like Fisher's exact test).
- Categorical data: The data must be categorical, meaning it represents counts or frequencies of observations in different categories.
Violating these assumptions can lead to inaccurate results.
Conclusion: Mastering the Chi-Square Table for Effective Analysis
The chi-square table and its critical values are indispensable tools for conducting hypothesis tests on categorical data. Understanding the principles of the chi-square distribution, correctly locating critical values on the table, interpreting results, and being mindful of the test's assumptions are vital for drawing valid conclusions from your statistical analyses. While software can automate calculations, a solid grasp of the underlying principles remains crucial for effective data analysis and interpretation. This comprehensive guide serves as a valuable resource for researchers and students alike, empowering them to confidently apply the chi-square test in their work. By understanding the critical value's role in decision-making within the context of the chi-square test, you can significantly enhance the rigor and reliability of your statistical analyses. Remember always to consider the limitations of the test and interpret the results cautiously within the context of your research question.
Latest Posts
Latest Posts
-
Equal Sharing Of Electrons Between Atoms
May 12, 2025
-
Are Lysosomes In Eukaryotes Or Prokaryotes
May 12, 2025
-
How Did Thomas Malthus Influence Darwin
May 12, 2025
-
What Is An Example Of Possibilism
May 12, 2025
-
Two Sample T Test With Equal Variances
May 12, 2025
Related Post
Thank you for visiting our website which covers about Critical Value Of Chi Square Table . We hope the information provided has been useful to you. Feel free to contact us if you have any questions or need further assistance. See you next time and don't miss to bookmark.