How To Find The F Ratio
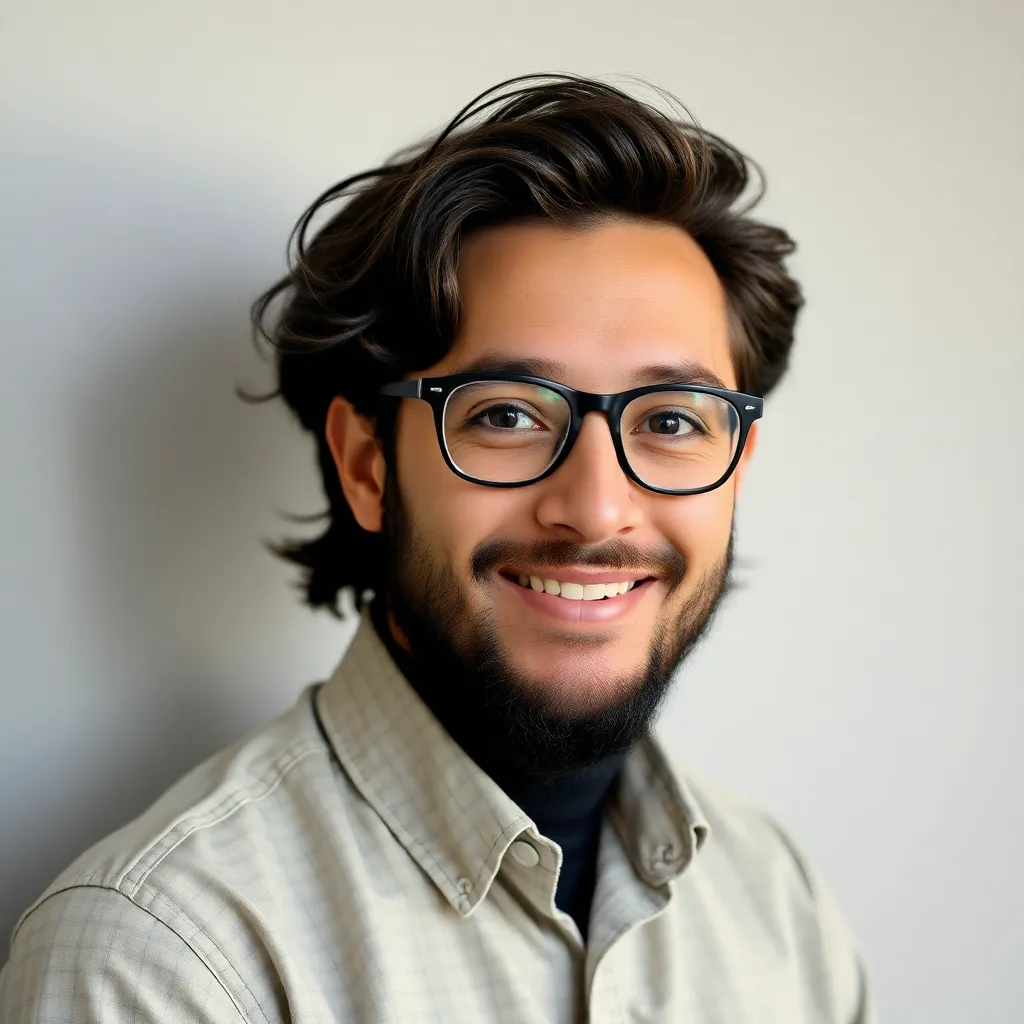
Muz Play
May 11, 2025 · 6 min read
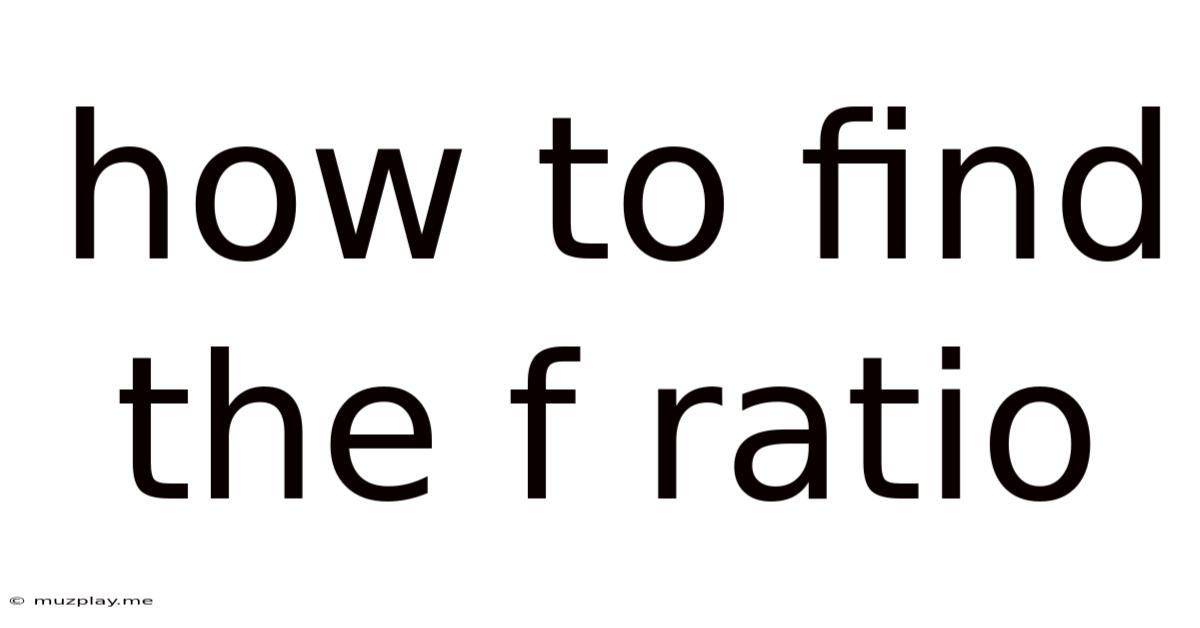
Table of Contents
How to Find the F-Ratio: A Comprehensive Guide
The F-ratio, a cornerstone of ANOVA (Analysis of Variance) and other statistical tests, is a crucial tool for comparing variances between different groups. Understanding how to calculate and interpret the F-ratio is essential for researchers across various disciplines. This comprehensive guide will delve into the intricacies of finding the F-ratio, explaining the underlying concepts, the step-by-step calculation process, and its practical applications.
Understanding the F-Ratio: A Conceptual Overview
The F-ratio is essentially a ratio of two variances: the variance between groups and the variance within groups. It's designed to test the null hypothesis that there's no significant difference between the means of several groups. A large F-ratio suggests that the variance between groups is significantly larger than the variance within groups, implying a strong likelihood that the group means are different. Conversely, a small F-ratio indicates that the differences between group means are likely due to random chance.
In simpler terms: Imagine you're comparing the heights of students from three different schools. The F-ratio helps determine if the difference in average heights between schools is statistically significant or simply due to random variation within each school's student population. A high F-ratio suggests a significant difference; a low F-ratio suggests the differences are negligible.
Components of the F-Ratio: Dissecting the Formula
The F-ratio is calculated using the following formula:
F = Mean Square Between Groups (MSB) / Mean Square Within Groups (MSW)
Let's break down each component:
1. Mean Square Between Groups (MSB)
MSB measures the variability between the different groups being compared. It reflects how much the group means differ from the overall mean of all groups. A large MSB indicates substantial differences between the group means. The formula for MSB is:
MSB = SSB / df_between
Where:
-
SSB (Sum of Squares Between Groups): This represents the sum of squared differences between each group mean and the overall grand mean, weighted by the number of observations in each group. It quantifies the total variability attributable to differences between groups. The formula for SSB is:
SSB = Σ nᵢ (x̄ᵢ - x̄)²
nᵢ
= number of observations in group ix̄ᵢ
= mean of group ix̄
= overall grand mean of all groups
-
df_between (Degrees of Freedom Between Groups): This represents the number of groups minus 1. It reflects the number of independent pieces of information used to estimate the variability between groups. The formula is:
df_between = k - 1
k
= number of groups
2. Mean Square Within Groups (MSW)
MSW measures the variability within each group. It represents the average variability of individual data points around their respective group means. A small MSW suggests that the data points within each group are closely clustered around their means. The formula for MSW is:
MSW = SSW / df_within
Where:
-
SSW (Sum of Squares Within Groups): This represents the sum of squared differences between each individual data point and its group mean. It quantifies the total variability attributable to differences within groups. The formula for SSW is:
SSW = Σ Σ (xᵢⱼ - x̄ᵢ)²
xᵢⱼ
= individual data point j in group ix̄ᵢ
= mean of group i
-
df_within (Degrees of Freedom Within Groups): This represents the total number of observations minus the number of groups. It reflects the number of independent pieces of information used to estimate the variability within groups. The formula is:
df_within = N - k
N
= total number of observationsk
= number of groups
Step-by-Step Calculation of the F-Ratio: A Practical Example
Let's illustrate the F-ratio calculation with a hypothetical example. Suppose we're comparing the average test scores of students from three different teaching methods (Method A, Method B, and Method C). We have the following data:
Method A | Method B | Method C |
---|---|---|
80 | 75 | 90 |
85 | 80 | 85 |
90 | 85 | 95 |
75 | 70 | 80 |
Step 1: Calculate the means for each group and the overall grand mean.
- Method A mean (x̄ₐ) = 82.5
- Method B mean (x̄բ) = 77.5
- Method C mean (x̄꜀) = 87.5
- Overall grand mean (x̄) = 82.5
Step 2: Calculate SSB (Sum of Squares Between Groups).
SSB = 4(82.5 - 82.5)² + 4(77.5 - 82.5)² + 4(87.5 - 82.5)² = 200
Step 3: Calculate SSW (Sum of Squares Within Groups).
SSW = (80-82.5)² + (85-82.5)² + (90-82.5)² + (75-82.5)² + ... + (80-87.5)² = 150
Step 4: Calculate df_between and df_within.
- df_between = 3 - 1 = 2
- df_within = 12 - 3 = 9
Step 5: Calculate MSB and MSW.
- MSB = SSB / df_between = 200 / 2 = 100
- MSW = SSW / df_within = 150 / 9 = 16.67
Step 6: Calculate the F-ratio.
F = MSB / MSW = 100 / 16.67 ≈ 6
Interpreting the F-Ratio: Significance and p-values
The calculated F-ratio (6 in our example) needs to be compared to a critical F-value obtained from an F-distribution table or statistical software. This critical value depends on the degrees of freedom (df_between and df_within) and the chosen significance level (typically 0.05).
If the calculated F-ratio is greater than the critical F-value, you reject the null hypothesis. This means there's a statistically significant difference between the group means. The p-value associated with the F-ratio further quantifies the strength of evidence against the null hypothesis. A p-value less than the significance level (e.g., p < 0.05) provides strong evidence to reject the null hypothesis.
In our example, if the critical F-value at a 0.05 significance level with df_between = 2 and df_within = 9 is, say, 4.26, then our calculated F-ratio of 6 is greater. We would reject the null hypothesis, concluding that there is a statistically significant difference in test scores among the three teaching methods.
Practical Applications of the F-Ratio: Beyond ANOVA
While the F-ratio is prominently used in ANOVA (one-way, two-way, etc.), its applications extend beyond this. It's a crucial component in various statistical tests, including:
- Regression Analysis: The F-ratio assesses the overall significance of the regression model, determining if at least one predictor variable significantly influences the outcome variable.
- MANOVA (Multivariate Analysis of Variance): This extends ANOVA to situations with multiple dependent variables. The F-ratio helps determine if there are significant differences between groups across these multiple variables.
- Discriminant Analysis: Used to find linear combinations of variables that best separate groups. The F-ratio plays a role in assessing the significance of these discriminant functions.
Conclusion: Mastering the F-Ratio for Data Analysis
The F-ratio is a powerful statistical tool for comparing variances and drawing inferences about group differences. Understanding its calculation, components, and interpretation is vital for researchers in diverse fields. This guide provides a comprehensive overview, empowering you to confidently apply the F-ratio in your data analysis and draw meaningful conclusions from your findings. Remember to always consider the context of your data and the limitations of statistical tests when interpreting your results. Utilizing statistical software can significantly simplify the calculation process, allowing you to focus on interpreting the results and drawing meaningful conclusions from your data analysis.
Latest Posts
Latest Posts
-
How To Do Bohr Rutherford Diagrams
May 12, 2025
-
Is Milk Pure Substance Or Mixture
May 12, 2025
-
Power Series Of 1 1 X
May 12, 2025
-
Is Boron Trifluoride Polar Or Nonpolar
May 12, 2025
-
Which Point Of The Beam Experiences The Most Compression
May 12, 2025
Related Post
Thank you for visiting our website which covers about How To Find The F Ratio . We hope the information provided has been useful to you. Feel free to contact us if you have any questions or need further assistance. See you next time and don't miss to bookmark.