Hypothesis Test For A Population Mean
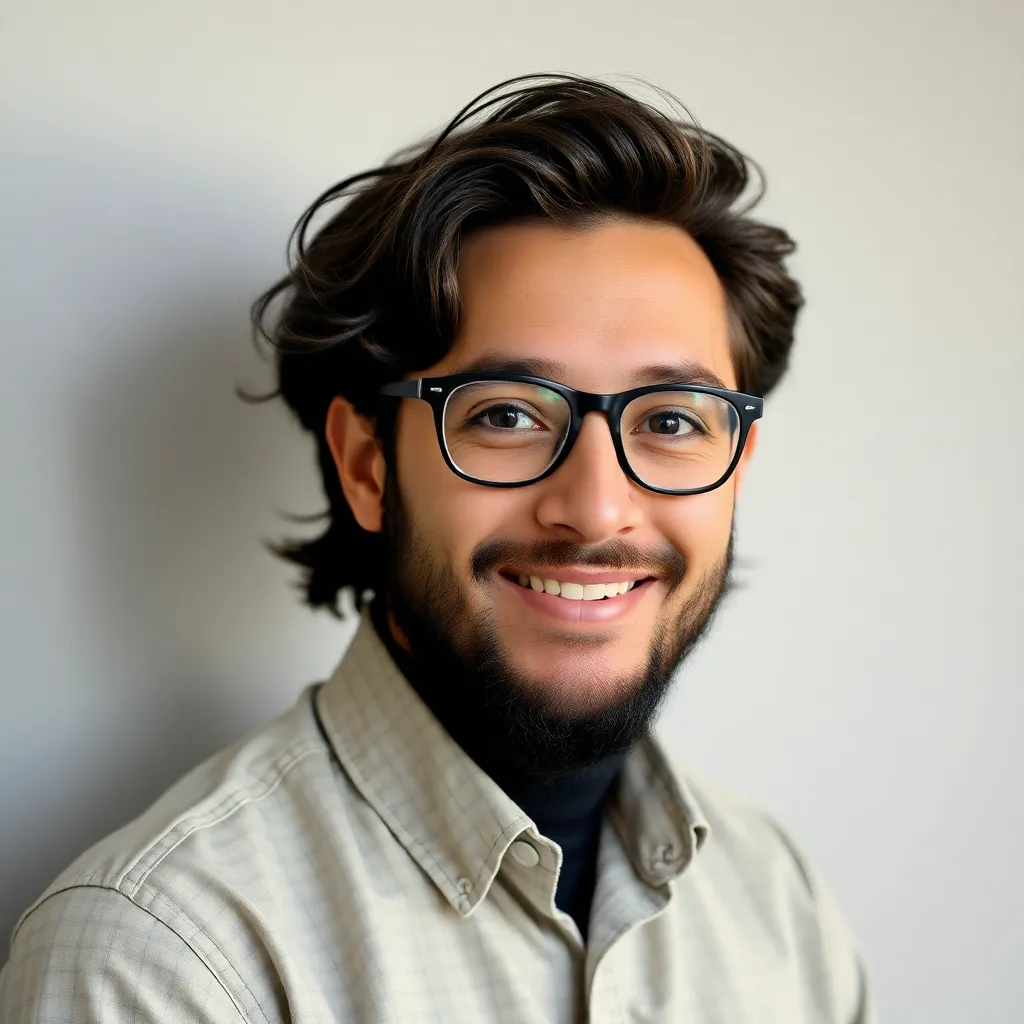
Muz Play
Apr 03, 2025 · 6 min read
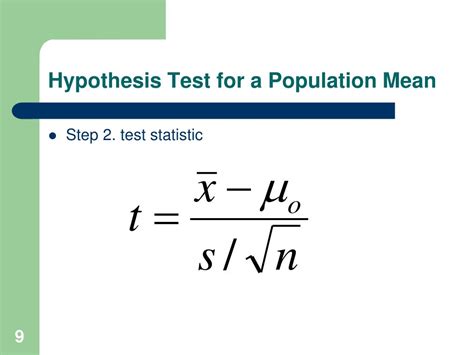
Table of Contents
Hypothesis Test for a Population Mean: A Comprehensive Guide
Understanding how to conduct a hypothesis test for a population mean is crucial in many fields, from statistics and data science to business and healthcare. This comprehensive guide will walk you through the entire process, explaining the underlying concepts, different test types, and the interpretation of results. We'll cover everything from formulating hypotheses to making informed conclusions based on your data analysis.
What is a Hypothesis Test for a Population Mean?
A hypothesis test for a population mean is a statistical procedure used to determine whether there is enough evidence to reject a null hypothesis about the population mean. The null hypothesis (H₀) typically states that there is no significant difference between the population mean and a hypothesized value. The alternative hypothesis (H₁) proposes a different state – often that there is a significant difference. The choice between a one-tailed or two-tailed test depends on the specific research question.
Key Concepts:
- Population Mean (μ): The average value of a characteristic within the entire population. This is often unknown and what we're trying to infer.
- Sample Mean (x̄): The average value of the characteristic within a sample drawn from the population. This is calculable and forms the basis of our inference.
- Significance Level (α): The probability of rejecting the null hypothesis when it is actually true (Type I error). Common values are 0.05 (5%) and 0.01 (1%).
- P-value: The probability of observing a sample mean as extreme as, or more extreme than, the one obtained, assuming the null hypothesis is true.
- Test Statistic: A value calculated from the sample data used to assess the evidence against the null hypothesis.
- Critical Value: The boundary value that separates the rejection region from the non-rejection region.
Types of Hypothesis Tests for a Population Mean
The choice of test depends primarily on whether the population standard deviation (σ) is known or unknown, and the sample size (n).
1. Z-test (Population Standard Deviation Known)
When the population standard deviation is known, or the sample size is large (generally n ≥ 30), a Z-test is appropriate. This test uses the standard normal distribution to determine the probability of obtaining the sample mean if the null hypothesis is true.
Formula for the Z-test statistic:
Z = (x̄ - μ₀) / (σ / √n)
Where:
- x̄ is the sample mean
- μ₀ is the hypothesized population mean
- σ is the population standard deviation
- n is the sample size
Procedure:
- State the hypotheses: Formulate the null (H₀) and alternative (H₁) hypotheses.
- Set the significance level (α): Choose a significance level (e.g., 0.05).
- Calculate the test statistic (Z): Use the formula above.
- Determine the critical value: Find the critical Z-value from the standard normal distribution table corresponding to the chosen significance level and whether it's a one-tailed or two-tailed test.
- Make a decision: If the absolute value of the calculated Z-statistic is greater than the critical Z-value, reject the null hypothesis. Otherwise, fail to reject the null hypothesis.
- Interpret the results: State the conclusion in the context of the problem.
2. t-test (Population Standard Deviation Unknown)
When the population standard deviation is unknown, which is usually the case, a t-test is used. The t-test uses the t-distribution, which accounts for the uncertainty introduced by estimating the population standard deviation from the sample.
Formula for the t-test statistic:
t = (x̄ - μ₀) / (s / √n)
Where:
- x̄ is the sample mean
- μ₀ is the hypothesized population mean
- s is the sample standard deviation
- n is the sample size
Procedure: The procedure is identical to the Z-test, except that the t-distribution is used instead of the standard normal distribution to determine the critical value. The degrees of freedom for the t-distribution are n - 1.
Choosing Between Z-test and t-test
- Large Sample Size (n ≥ 30): Both Z-test and t-test will yield similar results. The Z-test is often preferred for simplicity.
- Small Sample Size (n < 30): The t-test is the appropriate choice because it accounts for the additional uncertainty associated with estimating the population standard deviation from a small sample.
- Population Standard Deviation Known: Use the Z-test.
- Population Standard Deviation Unknown: Use the t-test.
One-Tailed vs. Two-Tailed Tests
The choice between a one-tailed and a two-tailed test depends on the research question.
-
Two-tailed test: Used when we want to test if the population mean is significantly different from the hypothesized mean, without specifying the direction of the difference (i.e., greater than or less than). The alternative hypothesis is H₁: μ ≠ μ₀.
-
One-tailed test: Used when we want to test if the population mean is significantly greater than or significantly less than the hypothesized mean. The alternative hypothesis is either H₁: μ > μ₀ (right-tailed) or H₁: μ < μ₀ (left-tailed).
Interpreting P-values and Making Conclusions
The p-value provides the probability of obtaining the observed results (or more extreme results) if the null hypothesis is true. A small p-value suggests strong evidence against the null hypothesis.
- P-value ≤ α: Reject the null hypothesis. There is sufficient evidence to support the alternative hypothesis.
- P-value > α: Fail to reject the null hypothesis. There is not enough evidence to support the alternative hypothesis.
It's crucial to remember that "failing to reject the null hypothesis" doesn't mean the null hypothesis is true. It simply means there isn't enough evidence to reject it based on the available data.
Assumptions of Hypothesis Tests for Population Mean
The validity of hypothesis tests for the population mean relies on several assumptions:
- Random Sampling: The sample must be randomly selected from the population to ensure the sample is representative.
- Independence: The observations in the sample must be independent of each other.
- Normality: For small sample sizes (n < 30), the population should be approximately normally distributed. For large sample sizes, the Central Limit Theorem often ensures that the sampling distribution of the mean is approximately normal, even if the population is not.
Practical Example: Testing the Average Weight of Apples
Let's say a farmer wants to test whether the average weight of his apples is 150 grams. He takes a random sample of 50 apples and finds that the sample mean weight is 155 grams, with a sample standard deviation of 10 grams. He wants to conduct a hypothesis test at a significance level of α = 0.05.
Since the population standard deviation is unknown and the sample size is large (n=50), we use a t-test.
-
Hypotheses:
- H₀: μ = 150 grams
- H₁: μ ≠ 150 grams (two-tailed test)
-
Significance level: α = 0.05
-
Test statistic: t = (155 - 150) / (10 / √50) ≈ 3.54
-
Degrees of freedom: df = 50 - 1 = 49
-
Critical value: Using a t-table with df = 49 and α = 0.05 (two-tailed), the critical t-value is approximately ±2.01.
-
Decision: Since the calculated t-value (3.54) is greater than the critical t-value (2.01), we reject the null hypothesis.
-
Conclusion: There is sufficient evidence at the 0.05 significance level to conclude that the average weight of the farmer's apples is significantly different from 150 grams.
Conclusion
Hypothesis testing for a population mean is a powerful statistical tool used to make inferences about populations based on sample data. Understanding the different test types, assumptions, and the interpretation of results is essential for applying this technique correctly and drawing meaningful conclusions. Remember to always consider the context of your research question and choose the appropriate test and significance level. By mastering these concepts, you can effectively analyze data and make data-driven decisions in various fields.
Latest Posts
Latest Posts
-
Prokaryotes That Obtain Their Energy From Chemical Compounds Are Called
Apr 04, 2025
-
What Does High Absorbance Mean In Spectrophotometry
Apr 04, 2025
-
Electric Field Due To A Disk Of Charge
Apr 04, 2025
-
Compare And Contrast Skeletal Smooth And Cardiac Muscle
Apr 04, 2025
-
The Integuments Of The Ovule Develop Into The
Apr 04, 2025
Related Post
Thank you for visiting our website which covers about Hypothesis Test For A Population Mean . We hope the information provided has been useful to you. Feel free to contact us if you have any questions or need further assistance. See you next time and don't miss to bookmark.