If The Median Is Greater Than The Mean
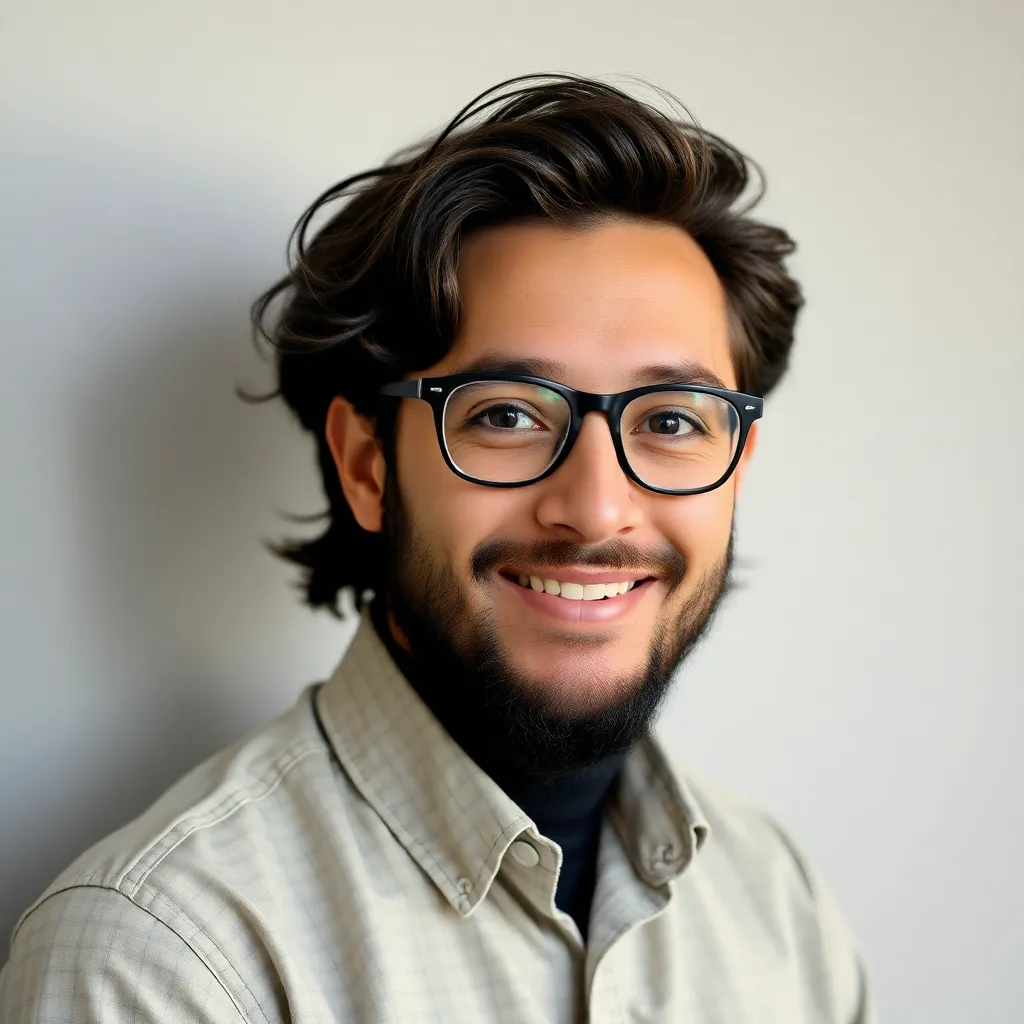
Muz Play
Apr 22, 2025 · 6 min read

Table of Contents
If the Median is Greater Than the Mean: Understanding Skewed Distributions
The relationship between the mean, median, and mode of a dataset provides valuable insights into its underlying distribution. While the mean represents the average value, the median signifies the central value when data is ordered, and the mode indicates the most frequent value. A key observation that can reveal much about the data's shape is when the median exceeds the mean. This scenario signifies a specific type of distribution—a left-skewed distribution, also known as a negatively skewed distribution. Understanding why this happens and what it implies is crucial for accurate data interpretation and informed decision-making across various fields.
Understanding Mean, Median, and Skewness
Before delving into the implications of a median greater than the mean, let's briefly review these key statistical measures:
The Mean: The Average Value
The mean is calculated by summing all values in a dataset and dividing by the number of values. It's the most commonly used measure of central tendency, but it's highly susceptible to outliers—extreme values that can significantly distort the average.
The Median: The Middle Value
The median represents the middle value when the data is arranged in ascending order. If the dataset has an even number of values, the median is the average of the two middle values. The median is less sensitive to outliers than the mean.
Skewness: The Asymmetry of the Distribution
Skewness measures the asymmetry of a probability distribution. A symmetrical distribution (like a normal distribution) has a mean, median, and mode that are all equal. However, skewed distributions have these measures at different values.
-
Left-Skewed (Negatively Skewed): In a left-skewed distribution, the tail extends more to the left. The mean is pulled down by the extreme values on the left, resulting in a mean lower than the median. This is the scenario we'll focus on where Median > Mean.
-
Right-Skewed (Positively Skewed): In a right-skewed distribution, the tail extends more to the right. The mean is pulled up by the extreme values on the right, resulting in a mean higher than the median. Mean > Median.
Why Median > Mean Implies a Left-Skewed Distribution
When the median is greater than the mean, it indicates that the distribution is skewed to the left. The presence of a few extremely low values pulls the mean down significantly, while the median, being resistant to outliers, remains relatively unaffected. These low values create a long tail on the left side of the distribution, leading to a negative skew.
Imagine a dataset representing the income of individuals in a particular town. If a few individuals have extremely low incomes (e.g., due to unemployment or low-paying jobs), these low values will significantly reduce the mean income. However, the median income will remain relatively unaffected as it's solely determined by the middle value. In this scenario, the median income would likely be greater than the mean income, revealing a left-skewed distribution. This distribution would suggest that a large portion of the population earns around the median income, with a small percentage earning considerably less.
Real-World Examples of Left-Skewed Distributions
Left-skewed distributions are common in various real-world scenarios:
-
Income Distribution: As illustrated above, income distributions often exhibit left-skew, with a majority of people earning within a certain range and a smaller proportion earning significantly less.
-
Test Scores: In a relatively easy test, most students achieve high scores, while a few students score significantly lower, resulting in a left-skewed distribution.
-
Asset Values: The value of assets like real estate or stocks might show left skewness. Most assets might hold steady or appreciate at a moderate rate, but a few assets might experience drastic devaluation, skewing the distribution to the left.
-
Age at Death: In countries with good healthcare and living standards, most people live to a relatively old age, while a smaller number die much younger. This pattern results in a left-skewed distribution of age at death.
-
Customer Service Response Time: Many companies aim for fast response times, which leads to most customer support tickets being resolved quickly. A few tickets might experience lengthy delays due to complex issues, producing a left-skewed distribution.
Implications of a Left-Skewed Distribution
The observation that the median is greater than the mean carries significant implications:
-
Outlier Detection: A large difference between the mean and median strongly suggests the presence of outliers or extreme values at the lower end of the distribution. Investigating these outliers is crucial to understand the underlying reasons behind their occurrence. They could represent errors in data collection, exceptional circumstances, or significant structural issues within the system under study.
-
Data Interpretation: Simply using the mean to represent the average can be misleading when dealing with left-skewed data. The median provides a more robust and representative measure of central tendency in such cases. Understanding the distribution is essential for drawing accurate conclusions from the data. Policymakers, for example, might focus on median income rather than mean income to better understand the financial well-being of the population.
-
Model Selection: The choice of statistical models and techniques should be tailored to the nature of the distribution. Using models designed for symmetrical data (like linear regression) on skewed data can lead to inaccurate or misleading results. Transformations such as logarithmic transformations might be necessary to normalize the data before applying suitable models.
Analyzing Left-Skewed Data: Techniques and Considerations
Analyzing data exhibiting a left-skew requires careful consideration:
-
Visualizations: Histograms, box plots, and probability plots are valuable tools for visualizing the distribution and identifying the presence of outliers or skewness. These visualizations provide a clear picture of the data's shape and help in choosing appropriate summary statistics and analytical methods.
-
Robust Statistical Measures: In addition to the median, other robust measures of central tendency, such as trimmed means (means calculated after removing a certain percentage of extreme values), are suitable for summarizing left-skewed data.
-
Data Transformation: To make the data more suitable for standard statistical methods, transformation techniques such as logarithmic or square root transformations can be applied. These transformations compress the range of the data, reducing the impact of outliers and making the distribution closer to a normal distribution.
-
Non-parametric Methods: Non-parametric statistical methods, which do not assume any specific underlying distribution, are often more appropriate for analyzing left-skewed data than parametric methods, which require assumptions about the distribution. Examples of non-parametric methods include the Mann-Whitney U test and the Wilcoxon signed-rank test.
Conclusion: The Significance of Understanding Skewness
The observation that the median is greater than the mean is a clear indicator of a left-skewed distribution. This understanding is not merely an academic exercise; it's essential for accurate data interpretation and sound decision-making in various fields. By recognizing the presence of skewness and applying appropriate analytical methods, researchers and practitioners can avoid misinterpretations and draw more reliable and meaningful conclusions from their data. Furthermore, understanding the implications of left-skewness aids in designing more effective strategies across various disciplines, from social policy to finance and engineering. Always remember to visualize your data, consider the presence of outliers, and choose analytical methods that align with the characteristics of your dataset to ensure robust and reliable analysis.
Latest Posts
Latest Posts
-
Match The Name Of The Eukaryotic Organism With Its Description
Apr 22, 2025
-
The Fiber Cell Membrane Is Termed The
Apr 22, 2025
-
How Many Electrons In Third Shell
Apr 22, 2025
-
Do Nonmetals Gain Or Lose Electrons
Apr 22, 2025
-
Where Do Chemosynthetic Get Their Energy
Apr 22, 2025
Related Post
Thank you for visiting our website which covers about If The Median Is Greater Than The Mean . We hope the information provided has been useful to you. Feel free to contact us if you have any questions or need further assistance. See you next time and don't miss to bookmark.