Row Vs Column Percentages Independent Variable
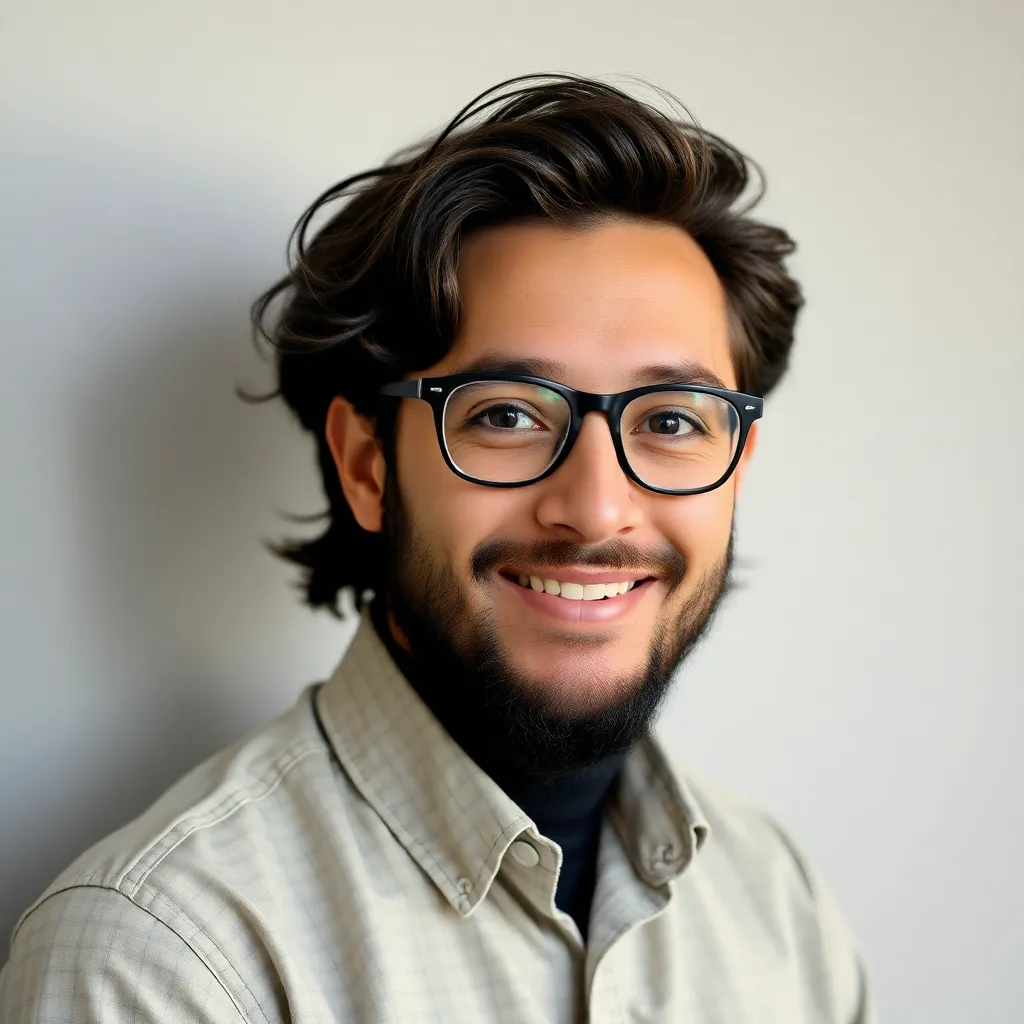
Muz Play
Apr 10, 2025 · 7 min read

Table of Contents
Row vs. Column Percentages: Understanding Independent Variables in Data Analysis
Understanding the difference between row and column percentages is crucial for accurately interpreting data, especially when dealing with independent variables. This distinction is fundamental in various analytical methods, including cross-tabulation, chi-square tests, and the visualization of relationships between categorical variables. This comprehensive guide will delve into the nuances of row versus column percentages, clarifying their applications and implications for interpreting the influence of independent variables.
What are Row and Column Percentages?
Before diving into the complexities, let's establish a clear understanding of the basics. Imagine a contingency table, a two-way table showing the frequency distribution of two categorical variables. This table forms the foundation for calculating both row and column percentages.
-
Row Percentages: These percentages are calculated for each row of the contingency table. They represent the proportion of each category of the dependent variable within each category of the independent variable. The denominator is the row total. In essence, row percentages answer the question: "What percentage of the dependent variable falls into each category, given a specific category of the independent variable?"
-
Column Percentages: Conversely, column percentages are calculated for each column of the contingency table. They represent the proportion of each category of the independent variable within each category of the dependent variable. The denominator is the column total. Column percentages answer: "What percentage of the independent variable falls into each category, given a specific category of the dependent variable?"
Identifying the Independent Variable: The Key to Correct Interpretation
The critical step in determining whether to use row or column percentages lies in correctly identifying the independent variable. The independent variable is the variable that is believed to influence or cause a change in the dependent variable. It is the variable that is manipulated (in experimental settings) or observed (in observational studies). The dependent variable, on the other hand, is the variable that is measured or observed to see if it changes in response to the independent variable.
Example: Let's consider a study examining the relationship between smoking (independent variable) and lung cancer (dependent variable). The researchers hypothesize that smoking influences the likelihood of developing lung cancer.
In this scenario:
- Independent Variable: Smoking (Yes/No)
- Dependent Variable: Lung Cancer (Yes/No)
A contingency table would show the frequency of individuals in each combination: smokers with lung cancer, smokers without lung cancer, non-smokers with lung cancer, and non-smokers without lung cancer. To understand the influence of smoking (the independent variable) on lung cancer (the dependent variable), we would use column percentages. This is because we want to see what percentage of smokers developed lung cancer compared to what percentage of non-smokers developed lung cancer. The denominator (the column total) represents the total number of individuals in each smoking category (smokers and non-smokers).
When to Use Row Percentages vs. Column Percentages
The choice between row and column percentages depends entirely on the research question and the variables involved. Here's a breakdown:
-
Use Column Percentages when:
- You want to analyze the effect of the independent variable on the dependent variable.
- You want to compare the distribution of the dependent variable across different categories of the independent variable.
- Your research question focuses on the conditional probabilities of the dependent variable, given the independent variable. (e.g., What is the probability of developing lung cancer given that a person is a smoker?)
-
Use Row Percentages when:
- You want to analyze the effect of the dependent variable on the independent variable. (This is less common in most analyses).
- You are interested in comparing the distribution of the independent variable across categories of the dependent variable.
- Your research question focuses on the conditional probabilities of the independent variable, given the dependent variable. (e.g., What is the probability of being a smoker given that a person has lung cancer?). Note that while statistically valid, this interpretation focuses on a conditional probability that may be less relevant to causal inference in many contexts.
In summary: If the goal is to assess the effect of the independent variable, use column percentages. Otherwise, if the goal is to assess the effect of the dependent variable (less common and typically involving a different theoretical approach), then use row percentages.
Practical Applications and Examples
Let's illustrate with more concrete examples:
Example 1: Effectiveness of a New Drug
Suppose a pharmaceutical company is testing a new drug to treat hypertension. They conduct a clinical trial and record the following data:
Drug Effective | Drug Ineffective | Total | |
---|---|---|---|
Treatment Group | 150 | 50 | 200 |
Control Group | 75 | 125 | 200 |
Total | 225 | 175 | 400 |
Independent Variable: Treatment Group (Treatment/Control) Dependent Variable: Drug Effectiveness (Effective/Ineffective)
To analyze the effectiveness of the drug, we use column percentages:
- Treatment Group: (150/200) * 100% = 75% effective
- Control Group: (75/200) * 100% = 37.5% effective
This shows that the drug is significantly more effective in the treatment group than in the control group.
Example 2: Voting Preferences and Age
Consider a survey examining voting preferences and age:
Voted for Candidate A | Voted for Candidate B | Total | |
---|---|---|---|
18-35 | 100 | 150 | 250 |
36-55 | 120 | 80 | 200 |
55+ | 80 | 120 | 200 |
Total | 300 | 350 | 650 |
Independent Variable: Age Group Dependent Variable: Voting Preference
Analyzing the influence of age on voting preference, we use row percentages:
- 18-35: Candidate A: (100/250) * 100% = 40%; Candidate B: (150/250) * 100% = 60%
- 36-55: Candidate A: (120/200) * 100% = 60%; Candidate B: (80/200) * 100% = 40%
- 55+: Candidate A: (80/200) * 100% = 40%; Candidate B: (120/200) * 100% = 60%
This shows the proportion of each age group that voted for each candidate.
Potential Pitfalls and Misinterpretations
Incorrectly interpreting row and column percentages can lead to flawed conclusions. Here are some common mistakes:
-
Confusing Independent and Dependent Variables: Failure to correctly identify the independent and dependent variables is a major source of error. This directly impacts the choice between row and column percentages and consequently the interpretation of the results.
-
Ignoring Marginal Totals: Focusing solely on the percentages within the table without considering the marginal totals (row and column sums) can lead to a skewed understanding of the overall distribution of the variables.
-
Over-interpreting Association as Causation: Even with correctly calculated percentages, it's crucial to remember that correlation doesn't equal causation. A statistically significant association between two variables doesn't automatically imply that one variable causes the other. Other factors may be influencing the relationship.
Advanced Considerations: Statistical Tests and Visualization
The choice of row versus column percentages often informs the selection of appropriate statistical tests. For instance, chi-square tests of independence typically utilize the observed frequencies to assess the association between categorical variables, and the interpretation of results is closely linked to the understanding of row and column percentages.
Visualizing data using appropriate charts and graphs (like bar charts or clustered bar charts) can greatly enhance understanding. The choice of chart type should align with whether row or column percentages are being emphasized. Clear labeling and informative titles are essential to avoid misinterpretations.
Conclusion
The distinction between row and column percentages is paramount for accurate data analysis. Correct identification of the independent and dependent variables is crucial for selecting the appropriate type of percentage and for drawing meaningful conclusions from your analysis. By understanding the nuances of these percentages and the potential pitfalls of misinterpretation, you can ensure that your data analysis is robust, insightful, and contributes to a clearer understanding of the relationship between variables. Remember to always contextualize your findings and avoid drawing causal conclusions without supporting evidence. Thorough data analysis, combined with clear visualization, forms the cornerstone of effective communication of research findings.
Latest Posts
Latest Posts
-
The Types Of Bonds Found In Nucleic Acids Are
Apr 18, 2025
-
Find A Formula For The Vector Field
Apr 18, 2025
-
Why Are Regions Called Promoters Essential To Rna Transcription
Apr 18, 2025
-
A Molecule With Partially Charged Areas
Apr 18, 2025
-
Label The Structures Of The Internal View Of The Skull
Apr 18, 2025
Related Post
Thank you for visiting our website which covers about Row Vs Column Percentages Independent Variable . We hope the information provided has been useful to you. Feel free to contact us if you have any questions or need further assistance. See you next time and don't miss to bookmark.