Criteria Of Good Measurement In Research
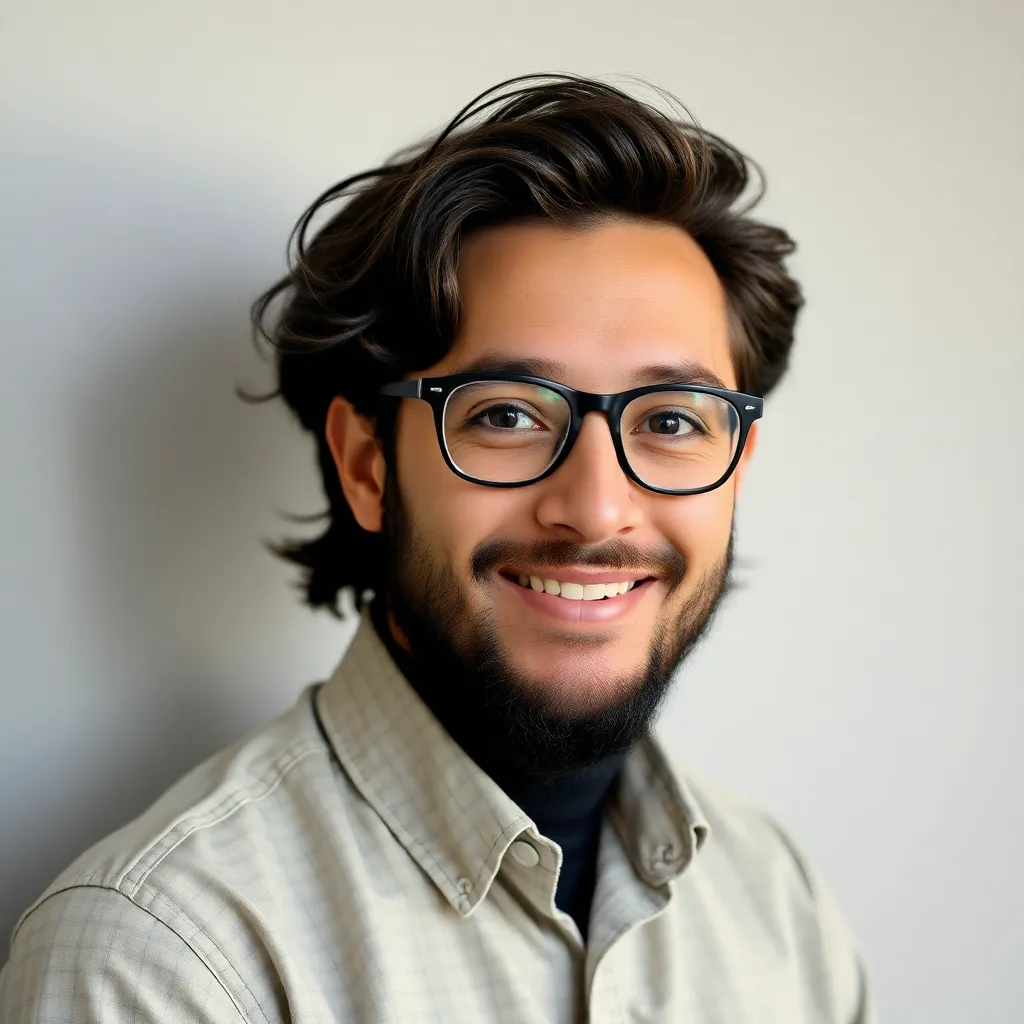
Muz Play
Apr 01, 2025 · 7 min read
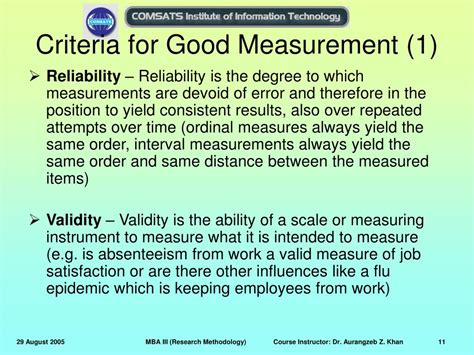
Table of Contents
Criteria of Good Measurement in Research
Measurement, the cornerstone of quantitative research, is the process of assigning numerals to objects, events, or properties according to a set of rules. The quality of your research hinges heavily on the quality of your measurement. Poor measurement leads to unreliable and invalid conclusions, undermining the entire research endeavor. Therefore, understanding the criteria of good measurement is paramount for any researcher. This article delves into these crucial criteria, exploring their significance and providing practical examples.
Defining Good Measurement: Validity and Reliability
Two fundamental concepts underpin the quality of measurement: validity and reliability. They are not mutually exclusive; rather, they are complementary aspects that together determine the trustworthiness of your findings.
Reliability: Consistency and Stability
Reliability refers to the consistency and stability of your measurement. A reliable measure yields similar results when repeated under the same conditions. Think of it like a well-calibrated scale: if you weigh the same object multiple times, you should get approximately the same weight each time. If the scale gives wildly different readings, it’s unreliable.
Several types of reliability exist:
-
Test-retest reliability: This assesses the consistency of a measure over time. The same instrument is administered to the same group on two different occasions, and the scores are correlated. A high correlation indicates good test-retest reliability.
-
Internal consistency reliability: This assesses the consistency of items within a measure. It evaluates whether different items on a questionnaire, for example, are measuring the same underlying construct. Cronbach's alpha is a commonly used statistic to measure internal consistency.
-
Inter-rater reliability: This assesses the consistency of observations made by different raters. If multiple observers are coding data, inter-rater reliability ensures they are applying the same criteria and reaching similar conclusions. Cohen's kappa is a frequently used statistic for assessing inter-rater reliability.
Example: A researcher uses a questionnaire to measure job satisfaction. High reliability would mean that individuals who score high on the questionnaire at one point in time would also score high at a later point in time (test-retest reliability) and that the different items within the questionnaire are all measuring aspects of job satisfaction (internal consistency reliability).
Validity: Accuracy and Meaningfulness
Validity refers to the accuracy and meaningfulness of your measurement. A valid measure actually measures what it intends to measure. It's about ensuring your instrument is truly capturing the concept you are interested in, not something else.
Various types of validity exist, including:
-
Content validity: This assesses whether the measure comprehensively covers all aspects of the construct being measured. It involves expert judgment to determine if the items adequately represent the domain of interest.
-
Criterion validity: This assesses the relationship between the measure and an external criterion. It can be further divided into:
- Concurrent validity: The measure is compared to a criterion measured at the same time.
- Predictive validity: The measure is used to predict a future criterion.
-
Construct validity: This assesses whether the measure accurately reflects the underlying theoretical construct. It's the most complex type of validity and often involves multiple lines of evidence, including convergent and discriminant validity. Convergent validity demonstrates that the measure correlates with other measures of the same construct, while discriminant validity shows that it does not correlate with measures of different constructs.
Example: A researcher is measuring intelligence. High content validity would mean the test encompasses a broad range of cognitive abilities typically associated with intelligence. High criterion validity would mean that scores on the test correlate strongly with academic performance (predictive validity) or with scores on other established intelligence tests (concurrent validity). High construct validity would mean that the test accurately measures the underlying theoretical concept of intelligence as defined in the literature.
Beyond Reliability and Validity: Other Important Criteria
While reliability and validity are the cornerstones, other criteria contribute to good measurement:
Objectivity: Minimizing Bias
Objectivity means that the measurement process is independent of the researcher's personal biases or subjective interpretations. The goal is to ensure that the same results would be obtained regardless of who conducts the measurement. This often involves using standardized procedures, clear operational definitions, and blinding techniques when appropriate.
Example: In a study measuring blood pressure, using a standardized blood pressure cuff and following a pre-defined procedure ensures objectivity. The researcher's personal opinions about the participants do not influence the blood pressure readings.
Sensitivity: Detecting Differences
A sensitive measure is capable of detecting subtle differences in the construct being measured. A measure with low sensitivity may fail to differentiate between individuals or groups that actually differ in the construct of interest.
Example: A scale that only measures weight in kilograms lacks sensitivity compared to one that measures weight in grams. The latter can detect smaller differences in weight.
Specificity: Avoiding Overlap
Specificity refers to the ability of a measure to accurately identify only the construct it is intended to measure, minimizing overlap with other related constructs. A measure with low specificity might be measuring multiple things at once, making it difficult to isolate the effect of the intended construct.
Example: A depression scale that also measures anxiety lacks specificity. It is hard to determine whether high scores reflect depression, anxiety, or both.
Practicality: Feasibility and Resources
Practicality considers the feasibility and resources required for the measurement process. A good measure should be relatively easy and inexpensive to administer, score, and interpret. Factors to consider include the time involved, the cost of materials, the availability of trained personnel, and the accessibility of the measurement instrument.
Example: A lengthy and complex questionnaire might be impractical for a large-scale survey, whereas a shorter, simpler questionnaire would be more practical.
Generalizability: Applicability to Wider Populations
Generalizability refers to the extent to which the findings from a study can be generalized to other populations, settings, or times. A highly generalizable measure is one that performs consistently across diverse contexts. This often requires careful consideration of sampling methods and the representativeness of the sample.
Example: A study using a particular intelligence test on a sample of university students might not be generalizable to a population of elderly individuals. The test might need to be adapted for that different population.
Ensuring Good Measurement: Practical Steps
Several practical steps can help researchers ensure good measurement in their studies:
-
Clearly Define the Construct: Before choosing or developing a measure, carefully define the theoretical concept you intend to measure. This provides a framework for evaluating the validity and reliability of your chosen instrument.
-
Review Existing Measures: Explore existing measures that have already been validated and shown to be reliable. Using established measures saves time and resources and increases the likelihood of obtaining valid and reliable data.
-
Pilot Test the Measure: Before conducting the main study, pilot test your measure on a small sample to identify any problems with the instrument or administration procedures. This allows for revisions and refinements before the main data collection begins.
-
Use Multiple Measures: Whenever possible, use multiple measures to assess the same construct. This helps to triangulate your findings and increase confidence in the validity and reliability of your results.
-
Analyze Data Thoroughly: Perform appropriate statistical analyses to assess the reliability and validity of your measures. Report these analyses transparently in your research.
Conclusion: The Imperative of Good Measurement
Good measurement is the bedrock of credible research. By understanding and applying the criteria of good measurement – reliability, validity, objectivity, sensitivity, specificity, practicality, and generalizability – researchers can significantly enhance the quality of their data and the trustworthiness of their findings. The investment in meticulous measurement pays substantial dividends in the form of robust, meaningful, and impactful research that advances knowledge and informs decision-making. Remember that the pursuit of good measurement is an ongoing process, requiring careful consideration at every stage of the research design and implementation.
Latest Posts
Latest Posts
-
Liquids Have A Definite Shape And Volume
Apr 02, 2025
-
Difference Between Molar Mass And Atomic Mass
Apr 02, 2025
-
Write In Standard Form The Equation Of Each Line
Apr 02, 2025
-
What Does Fad Stand For In Biology
Apr 02, 2025
-
The Outermost Layer Of The Heart Is Called The
Apr 02, 2025
Related Post
Thank you for visiting our website which covers about Criteria Of Good Measurement In Research . We hope the information provided has been useful to you. Feel free to contact us if you have any questions or need further assistance. See you next time and don't miss to bookmark.