What Are Factors In An Experiment
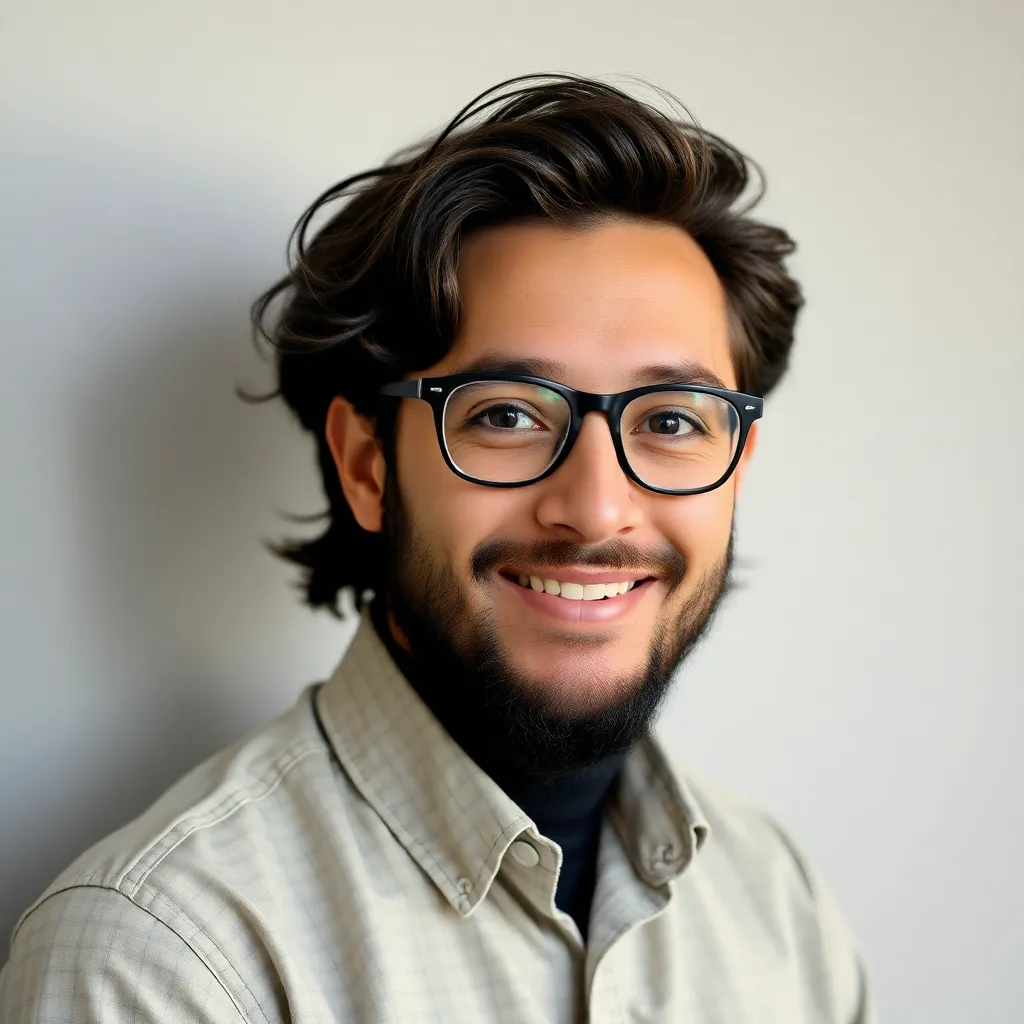
Muz Play
Mar 28, 2025 · 6 min read
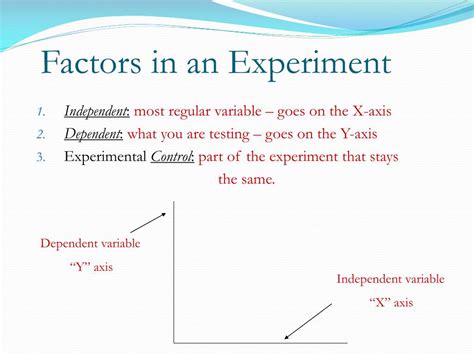
Table of Contents
What Are the Factors in an Experiment? A Comprehensive Guide
Understanding the factors involved in an experiment is crucial for conducting sound research and drawing valid conclusions. This comprehensive guide delves into the various types of factors, their roles, and how to effectively manage them to ensure the reliability and integrity of your experimental findings. We'll explore the nuances of independent, dependent, control, confounding, and mediating variables, providing clear examples and practical strategies for researchers at all levels.
Defining Experimental Factors: The Building Blocks of Research
In the realm of experimental design, a factor is any variable that can influence the outcome of an experiment. These variables are carefully manipulated or observed to determine their impact on the phenomenon under investigation. Identifying and understanding these factors is the cornerstone of a well-designed experiment. Failing to account for all relevant factors can lead to inaccurate conclusions and flawed interpretations.
Independent Variables: The Manipulated Variables
The independent variable (IV) is the variable that is manipulated or changed by the researcher. It's the presumed cause in a cause-and-effect relationship. The researcher systematically alters the independent variable to observe its effects on the dependent variable. For instance, in an experiment testing the effect of fertilizer on plant growth, the type and amount of fertilizer would be the independent variable.
-
Example: An experiment studying the impact of different teaching methods (lecture, group work, online learning) on student test scores. The teaching method is the independent variable.
-
Characteristics: Independent variables are typically categorical (e.g., different types of fertilizer) or continuous (e.g., varying dosages of a medication). They should be clearly defined and measurable to ensure consistent manipulation across experimental conditions.
Dependent Variables: The Measured Outcomes
The dependent variable (DV) is the variable that is measured or observed. It's the presumed effect in a cause-and-effect relationship. The researcher measures the dependent variable to determine the impact of the independent variable. In the fertilizer example, plant height or yield would be the dependent variable.
-
Example: In the teaching method experiment, the student test scores are the dependent variable. The researcher measures how these scores change as a result of the different teaching methods.
-
Characteristics: Dependent variables should be reliably measured using valid and reliable instruments. The selection of the appropriate dependent variable is crucial for accurately assessing the effects of the independent variable.
Control Variables: Maintaining Consistency
Control variables are variables that are held constant throughout the experiment to minimize their influence on the dependent variable. Controlling these variables ensures that any observed changes in the dependent variable are due to the manipulation of the independent variable and not to extraneous factors.
-
Example: In the fertilizer experiment, control variables might include the amount of sunlight, water, and soil type, all of which are kept consistent across different fertilizer groups to ensure a fair comparison.
-
Importance: Proper control of extraneous variables is paramount for establishing internal validity – the confidence that the observed effects are truly due to the independent variable and not other factors.
Confounding Variables: The Unwanted Guests
Confounding variables are extraneous variables that are not controlled and do influence the dependent variable. They create ambiguity in interpreting the results, making it difficult to determine whether the observed changes are due to the independent variable or the confounding variable.
-
Example: In the fertilizer experiment, if some plants receive more sunlight than others, sunlight becomes a confounding variable. It becomes impossible to isolate the effect of fertilizer alone on plant growth.
-
Mitigation: Careful experimental design and statistical techniques (e.g., random assignment, blocking, statistical control) can help to minimize the impact of confounding variables.
Mediating Variables: The Intervening Mechanisms
Mediating variables (also known as intermediary variables) explain the process or mechanism through which the independent variable affects the dependent variable. They represent the underlying causal pathway linking the IV and DV.
-
Example: Suppose an experiment shows that a new drug (IV) reduces blood pressure (DV). A mediating variable might be the drug's effect on reducing inflammation in blood vessels, which, in turn, lowers blood pressure.
-
Importance: Understanding mediating variables provides a deeper understanding of the relationship between the independent and dependent variables, going beyond simple correlation to explore the underlying causal processes.
Advanced Considerations in Factor Analysis
The complexities of experimental research often necessitate a more nuanced approach to factor analysis. Let's examine some key refinements:
Categorical vs. Continuous Variables: Choosing the Right Approach
Independent variables can be either categorical (e.g., type of therapy) or continuous (e.g., dosage of medication). The choice influences the statistical analysis applied. Categorical IVs often use ANOVA or chi-square tests, while continuous IVs frequently involve regression analysis.
Interaction Effects: When Variables Collaborate
Interaction effects occur when the effect of one independent variable on the dependent variable depends on the level of another independent variable. These interactions indicate that the variables don't act independently but rather in a combined manner.
-
Example: Imagine an experiment examining the impact of both fertilizer and watering frequency on plant growth. An interaction effect might occur if the positive effect of fertilizer is stronger under frequent watering than under infrequent watering.
-
Importance: Identifying interaction effects is critical for a complete understanding of the experimental results and avoids oversimplified interpretations.
Multiple Independent Variables: Factorial Designs
Experiments often involve multiple independent variables. Factorial designs systematically manipulate multiple IVs and assess their individual and combined effects on the DV. This allows researchers to investigate interaction effects and gain a more comprehensive understanding of the phenomenon under study.
- Example: A study investigating the impact of both sleep deprivation (IV1) and caffeine consumption (IV2) on cognitive performance (DV). A 2x2 factorial design would examine all four combinations: adequate sleep/no caffeine, adequate sleep/caffeine, sleep deprivation/no caffeine, and sleep deprivation/caffeine.
Moderating Variables: Influencing the Relationship
Moderating variables influence the strength or direction of the relationship between the independent and dependent variables. They don't explain the mechanism (like mediators), but rather alter the overall relationship.
-
Example: In the fertilizer experiment, soil type might act as a moderator. The effect of fertilizer on plant growth might be stronger in fertile soil than in poor soil.
-
Distinguishing Moderators and Mediators: Moderators change the relationship between IV and DV, while mediators explain the relationship. This distinction is crucial for proper interpretation.
Practical Strategies for Effective Factor Management
Effective management of experimental factors is crucial for obtaining reliable and meaningful results. Here are some practical strategies:
-
Careful Planning: Start with a clear research question and hypothesis. Identify all potential factors and determine which should be manipulated (IVs), measured (DVs), and controlled.
-
Random Assignment: Randomly assign participants or subjects to different experimental conditions to minimize bias and ensure that groups are comparable.
-
Blinding: When appropriate, use blinding techniques (single-blind or double-blind) to prevent bias from influencing the results.
-
Replication: Replicate the experiment to verify the findings and increase the generalizability of the results.
-
Statistical Analysis: Use appropriate statistical techniques to analyze the data and draw valid inferences.
-
Thorough Documentation: Maintain meticulous records of the experimental procedures, data collection, and analysis.
Conclusion: Mastering the Factors for Success
Understanding the various types of factors in an experiment – independent, dependent, control, confounding, mediating, and moderating variables – is essential for conducting rigorous research and generating reliable results. By carefully planning and controlling these factors, researchers can minimize bias, enhance internal validity, and draw accurate conclusions that advance our understanding of the world around us. Mastering these principles transforms experimentation from a haphazard process into a powerful tool for scientific discovery. Remember, meticulous attention to detail and a systematic approach are paramount for success in the experimental realm.
Latest Posts
Latest Posts
-
List The Types Of Persuasive Speeches
Mar 31, 2025
-
The Energy Needed To Start A Chemical Reaction Is Called
Mar 31, 2025
-
Person In Environment In Social Work
Mar 31, 2025
-
What Is The Funnel Used For
Mar 31, 2025
-
Chains Of Carbon Atoms Bonded To Hydrogen Atoms
Mar 31, 2025
Related Post
Thank you for visiting our website which covers about What Are Factors In An Experiment . We hope the information provided has been useful to you. Feel free to contact us if you have any questions or need further assistance. See you next time and don't miss to bookmark.