Based On The Frequency Distribution Above Is 22.5 A
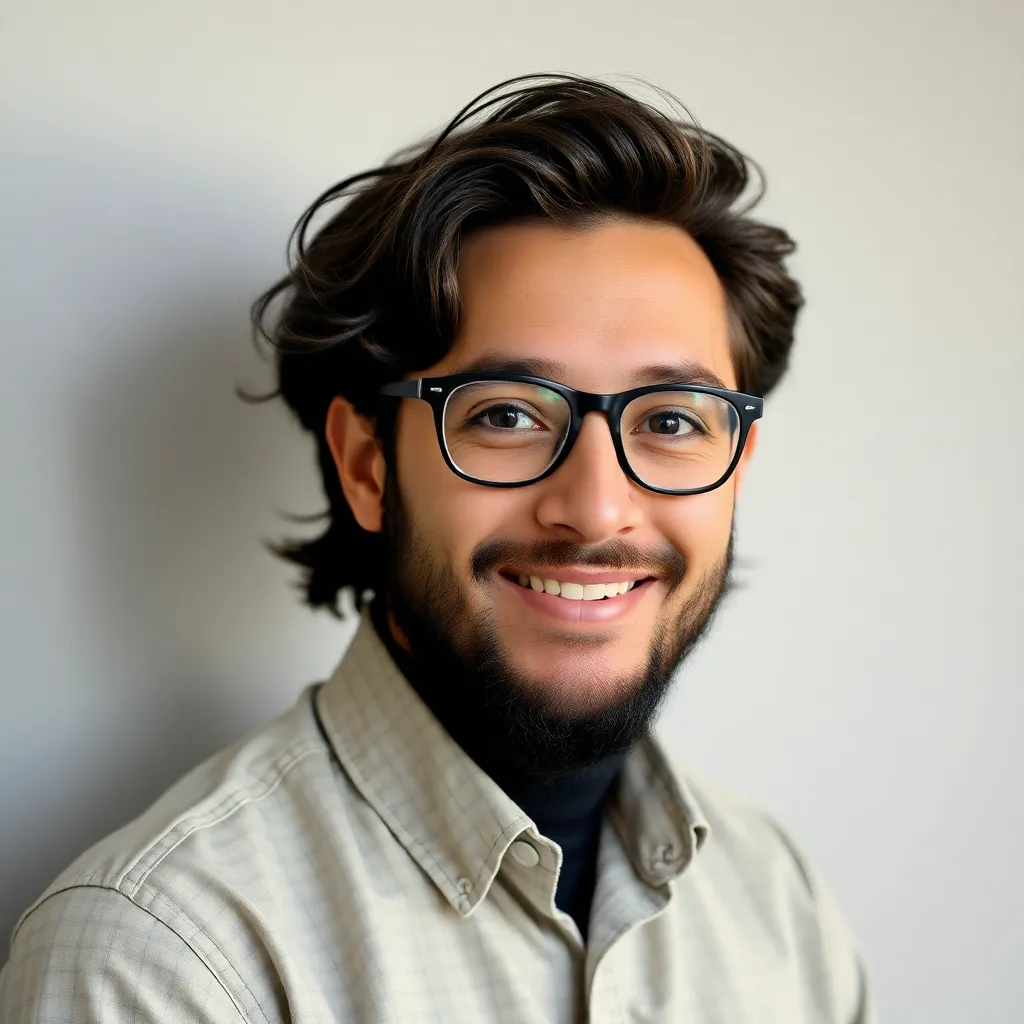
Muz Play
Apr 04, 2025 · 6 min read
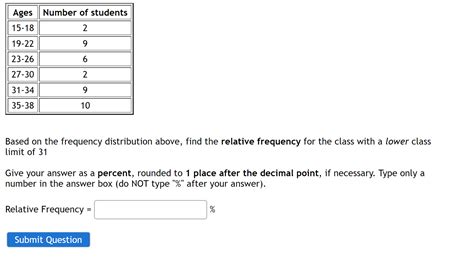
Table of Contents
Is 22.5 an Outlier? Determining Significance Based on Frequency Distribution
Understanding whether a data point, like 22.5, is an outlier requires more than just looking at the number itself. It depends heavily on the context of the data—specifically, its frequency distribution. A value might be considered an outlier in one dataset but perfectly normal in another. This article will delve into the methods for determining if 22.5 is an outlier, given a frequency distribution, examining various statistical techniques and offering practical guidance. We'll cover the importance of context, explore different outlier detection methods, and discuss the interpretation of results.
Understanding Frequency Distributions and Outliers
Before we determine if 22.5 is an outlier, let's establish a firm understanding of the key concepts.
-
Frequency Distribution: A frequency distribution shows how often different values appear in a dataset. It can be represented in various ways, including frequency tables, histograms, and cumulative frequency curves. The shape of the distribution (e.g., normal, skewed, bimodal) significantly influences outlier detection.
-
Outlier: An outlier is a data point that significantly deviates from other observations in a dataset. They can arise from measurement errors, data entry mistakes, or simply represent genuinely unusual occurrences within the population being studied. Identifying outliers is crucial as they can disproportionately affect statistical analyses and conclusions. Ignoring them might lead to inaccurate results and misleading interpretations.
-
Why Identifying Outliers Matters: Outliers can skew descriptive statistics (mean, standard deviation), inflate variability, and bias statistical models. Understanding their presence and potential causes is essential for accurate data analysis and robust decision-making.
Methods for Detecting Outliers based on Frequency Distribution
Several methods can be used to identify outliers, depending on the nature of the data and the specific research question. Here are some of the most common techniques:
1. Visual Inspection of Frequency Distributions:
-
Histograms: Creating a histogram of the data provides a visual representation of the frequency distribution. Outliers will often appear as isolated points far from the main cluster of data. This method is intuitive and helps quickly identify potential outliers.
-
Box Plots: Box plots effectively summarize the distribution of data, displaying the median, quartiles, and potential outliers. Data points falling outside the "whiskers" (typically 1.5 times the interquartile range from the box edges) are often flagged as outliers. This method is particularly useful for comparing distributions across different groups.
2. Z-Score Method:
The Z-score measures how many standard deviations a data point is from the mean. Data points with Z-scores above a certain threshold (often 2 or 3) are considered outliers.
-
Formula: Z = (x - μ) / σ, where x is the data point, μ is the mean, and σ is the standard deviation.
-
Limitations: The Z-score method assumes a normal distribution. If the data is significantly non-normal, this method may not be reliable.
3. Interquartile Range (IQR) Method:
The IQR is the difference between the third quartile (Q3) and the first quartile (Q1) of the data. Outliers are often defined as data points falling below Q1 - 1.5 * IQR or above Q3 + 1.5 * IQR.
-
Advantages: The IQR method is less sensitive to extreme values than the Z-score method and works well with skewed distributions.
-
Limitations: The choice of the multiplier (1.5) is somewhat arbitrary and can be adjusted depending on the specific context.
4. Modified Z-Score:
The modified Z-score is a robust alternative to the standard Z-score that is less sensitive to outliers. It uses the median absolute deviation (MAD) instead of the standard deviation.
-
Formula: Modified Z-score = 0.6745 * (x - median) / MAD
-
Advantages: More resistant to outliers than the standard Z-score.
5. Data Transformation:
Sometimes, data transformations (e.g., logarithmic, square root) can help normalize the distribution, making outlier detection more straightforward. This approach is particularly useful when dealing with skewed data.
Applying the Methods to Determine if 22.5 is an Outlier
To definitively answer whether 22.5 is an outlier, we need the actual frequency distribution. Let's consider a hypothetical example:
Hypothetical Frequency Distribution:
Assume we have a dataset with the following frequency distribution:
Value | Frequency |
---|---|
10-14 | 2 |
15-19 | 8 |
20-24 | 15 |
25-29 | 10 |
30-34 | 5 |
35-39 | 2 |
22.5 | 1 |
Analysis:
-
Visual Inspection: A histogram of this data would show a somewhat symmetrical distribution centered around the 20-24 range. The value 22.5 falls within this range, suggesting it's not dramatically different from other values.
-
Z-score: To calculate the Z-score, we'd need the mean and standard deviation of the entire dataset. Without the raw data, we can't calculate these precisely. However, a visual inspection strongly suggests that 22.5 would not have an exceptionally high Z-score.
-
IQR Method: The IQR would be calculated from the quartiles of the dataset. Given the apparent central tendency around the 20-24 range, 22.5 would likely fall within the 1.5 * IQR boundaries, thus not classified as an outlier.
-
Modified Z-Score: Similar to the standard Z-score, calculating the modified Z-score would require the complete dataset. However, given the visual observation, it's unlikely that 22.5 would have a modified Z-score indicating it's an outlier.
Conclusion (Based on Hypothetical Data):
In this hypothetical scenario, based on the provided frequency distribution, 22.5 is unlikely to be considered a significant outlier. Its value falls within a range containing a large proportion of the data, suggesting it is consistent with the overall distribution.
The Importance of Context and Further Considerations
The determination of whether 22.5 is an outlier is fundamentally dependent on the context of the entire dataset and its distribution. The frequency distribution provided is merely one piece of the puzzle. Several other factors need to be considered:
-
The Nature of the Data: The units of measurement and the scale of the data are critical. A value of 22.5 might be an outlier in a dataset measuring temperatures in Celsius, but not in a dataset measuring lengths in millimeters.
-
The Research Question: The specific research question will influence how outliers are handled. In some cases, outliers may be legitimately significant and warrant further investigation, while in others, they might be safely removed or adjusted.
-
Subject Matter Expertise: Experts in the field will often have valuable insight into whether particular data points are likely to be genuine or due to errors.
-
Data Cleaning: Before outlier analysis, it is essential to thoroughly clean the data to eliminate any obvious errors (data entry mistakes, corrupted data, etc.). These errors can lead to spurious identification of outliers.
-
Robust Statistical Methods: Using robust statistical methods that are less sensitive to outliers (e.g., median instead of mean) can mitigate the influence of outliers on the analysis.
Conclusion
Determining whether 22.5 is an outlier is not a simple yes or no answer. It depends entirely on the specific context of the frequency distribution and the data itself. By carefully examining the frequency distribution using appropriate methods (visual inspection, Z-scores, IQR, modified Z-scores) and considering the context of the data, we can make an informed decision about the significance of 22.5. Always remember that thorough data cleaning, awareness of potential biases, and informed interpretation of results are critical components of effective data analysis. Using this comprehensive approach to outlier detection will help you to draw more accurate and reliable conclusions from your data.
Latest Posts
Latest Posts
-
Acetic Acid Sodium Acetate Buffer Equation
Apr 05, 2025
-
What Is The Standard Electrode Potential
Apr 05, 2025
-
Negative Binomial Distribution Vs Binomial Distribution
Apr 05, 2025
-
Express 1 2cosa As A Product
Apr 05, 2025
-
Proteins Are Polymers Of Amino Acid Monomers
Apr 05, 2025
Related Post
Thank you for visiting our website which covers about Based On The Frequency Distribution Above Is 22.5 A . We hope the information provided has been useful to you. Feel free to contact us if you have any questions or need further assistance. See you next time and don't miss to bookmark.