Can Sample Variance Be Smaller Than Population Variance
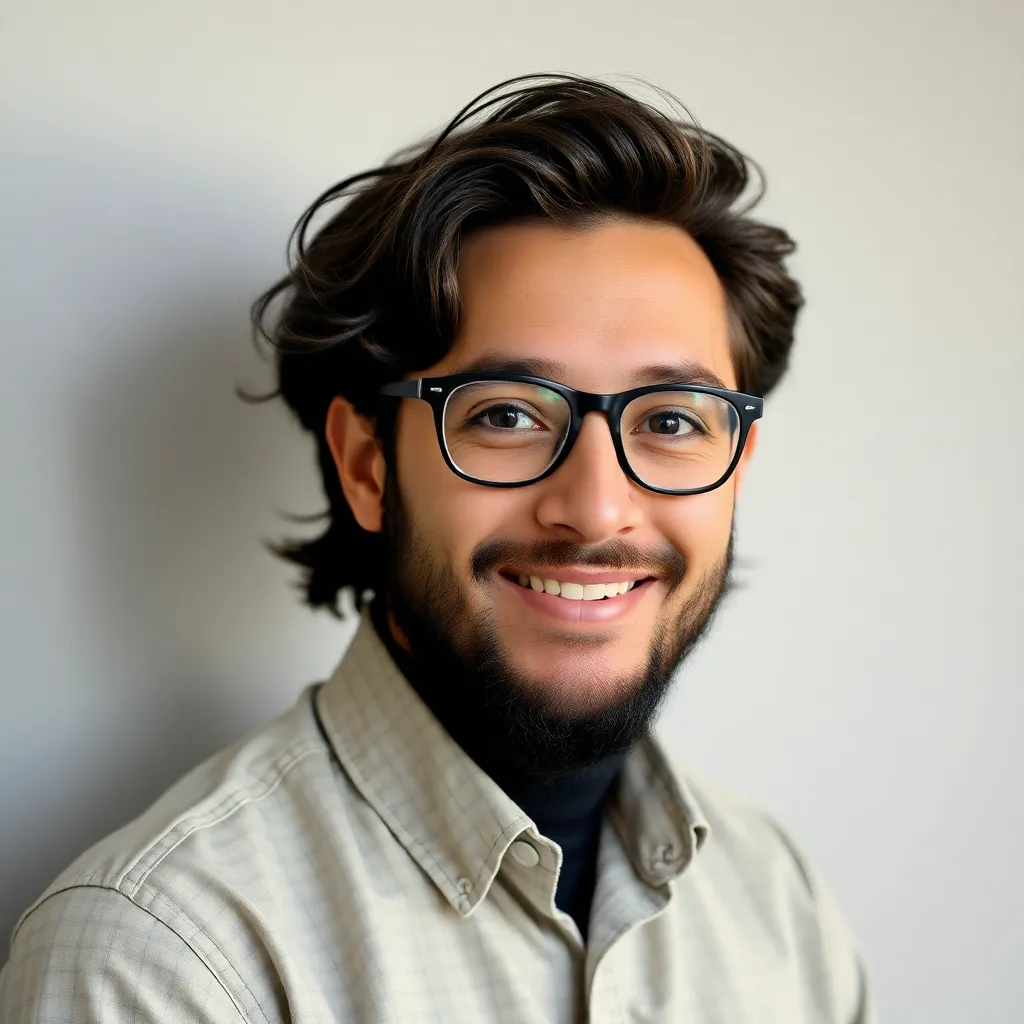
Muz Play
Mar 31, 2025 · 6 min read
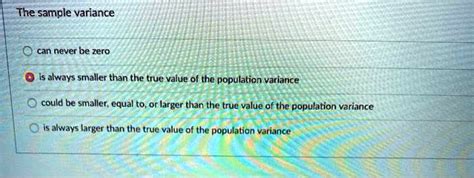
Table of Contents
Can Sample Variance Be Smaller Than Population Variance? A Deep Dive into Statistical Inference
Understanding the relationship between sample variance and population variance is crucial in statistics. While intuition might suggest that the sample variance should always be smaller than the population variance, this isn't always the case. This article delves into the intricacies of this relationship, exploring the mathematical underpinnings and providing practical examples to illuminate the nuances involved. We'll unpack the concepts of variance, sampling, and the implications of these differences for statistical inference.
Understanding Variance: A Foundation
Before diving into the central question, let's solidify our understanding of variance itself. Variance is a measure of the dispersion or spread of a dataset around its mean. A higher variance indicates greater variability, while a lower variance suggests data points are clustered closely around the mean. The population variance (σ²) is calculated using all data points in the entire population, while the sample variance (s²) is calculated using a subset of the population.
Population Variance (σ²)
The population variance is calculated as:
σ² = Σ(xi - μ)² / N
Where:
- xi represents each individual data point in the population.
- μ represents the population mean.
- N represents the total number of data points in the population.
Sample Variance (s²)
The sample variance, however, uses a slightly different formula:
s² = Σ(xi - x̄)² / (n - 1)
Where:
- xi represents each individual data point in the sample.
- x̄ represents the sample mean.
- n represents the number of data points in the sample.
The crucial difference lies in the denominator. The population variance uses N, the total population size, while the sample variance uses (n - 1), known as Bessel's correction. This correction is essential because sample variance is used to estimate the population variance. Using (n-1) provides an unbiased estimator of the population variance.
Why Bessel's Correction? The Unbiased Estimator
The seemingly small difference in the denominator significantly impacts the estimation. If we used 'n' instead of 'n-1' in the sample variance calculation, the resulting estimate would consistently underestimate the true population variance. This is because the sample mean (x̄) is calculated from the sample itself, and it tends to be closer to the data points within the sample than to the true population mean (μ). This leads to a smaller sum of squared deviations, resulting in an underestimation of the population variance.
Bessel's correction adjusts for this inherent bias, providing an unbiased estimate of the population variance. It accounts for the fact that we're using a sample, which naturally contains less information than the entire population. Therefore, we need to inflate the variance slightly to compensate for this loss of information.
Can Sample Variance Be Smaller Than Population Variance? Yes, It Can!
While Bessel's correction aims for an unbiased estimator, it doesn't guarantee that the sample variance will always be larger than the population variance. It's entirely possible, and even statistically probable in certain scenarios, for the sample variance to be smaller.
Here's why:
-
Random Sampling: The act of sampling itself introduces randomness. A particular sample might, by sheer chance, contain data points that are less dispersed than the overall population. This is especially likely with small sample sizes where the sample mean might be exceptionally close to the true population mean, and the deviations are smaller.
-
Sample Selection Bias: If the sampling method isn't truly random and introduces bias, the sample variance can deviate significantly from the population variance, potentially being smaller. For instance, if you selectively sample data points closer to the population mean, your sample variance will naturally be lower.
-
Statistical Fluctuation: Even with perfect random sampling, statistical fluctuations can lead to instances where the sample variance is smaller than the population variance. This is particularly true for smaller sample sizes.
Illustrative Examples
Let's consider two examples to clarify this concept:
Example 1: A "Lucky" Sample
Imagine a population with a variance of 100. We take a small sample (n=5) and, purely by chance, the five data points are remarkably close to the population mean. The sample variance might be, for instance, 20. In this case, the sample variance (20) is considerably smaller than the population variance (100). This scenario highlights the role of randomness in sampling.
Example 2: Biased Sampling
Suppose we're interested in the variance of heights among adults. If we deliberately sample only individuals of average height, our sample will exhibit a much lower variance than the population variance, which includes individuals of all height ranges. This clearly demonstrates how biased sampling can lead to a sample variance that underestimates the population variance.
Implications for Statistical Inference
The possibility of a sample variance being smaller than the population variance has significant implications for statistical inference, especially when drawing conclusions about the population based on sample data. This necessitates a cautious approach to interpreting sample statistics.
Hypothesis Testing
In hypothesis testing, where we use sample data to make inferences about the population, the difference between sample and population variances is crucial. Many statistical tests, like the t-test, rely on the assumption of equal variances or utilize adjustments if variances are significantly different. A smaller sample variance than expected could lead to incorrect conclusions if these assumptions aren't carefully considered.
Confidence Intervals
When constructing confidence intervals for the population mean, the sample variance plays a vital role in determining the width of the interval. A smaller sample variance would result in a narrower confidence interval, suggesting a more precise estimate of the population mean. However, this increased precision might be misleading if the sample variance underestimates the true population variance due to chance or bias.
Sample Size Matters
It's important to acknowledge that the likelihood of a sample variance being smaller than the population variance decreases with increasing sample size. As the sample size grows larger, the sample statistics become increasingly representative of the population parameters. Therefore, with large samples, the chance of observing a significantly smaller sample variance becomes less probable.
Conclusion: A Nuance Worth Understanding
The relationship between sample variance and population variance is not a simple, one-directional inequality. While Bessel's correction aims to produce an unbiased estimate, the inherent randomness of sampling and the possibility of biased sampling methods mean that the sample variance can indeed be smaller than the population variance. Recognizing this nuance is critical for accurate statistical inference and avoiding misinterpretations of data. Researchers must be aware of the potential for sampling variability and take appropriate steps to minimize bias and ensure adequate sample sizes to improve the reliability of their conclusions. Careful consideration of sampling methods and appropriate statistical techniques remain paramount in achieving accurate and robust findings.
Latest Posts
Latest Posts
-
How To Find Moles Of Naoh Used In Titration
Apr 02, 2025
-
Different Conformations Of The Same Compound
Apr 02, 2025
-
Induced Fit Model Vs Lock And Key
Apr 02, 2025
-
Colligative Properties Freezing Point Depression Lab
Apr 02, 2025
-
Matrix As A Product Of Elementary Matrices
Apr 02, 2025
Related Post
Thank you for visiting our website which covers about Can Sample Variance Be Smaller Than Population Variance . We hope the information provided has been useful to you. Feel free to contact us if you have any questions or need further assistance. See you next time and don't miss to bookmark.