Confidence Interval Calculator With Two Samples
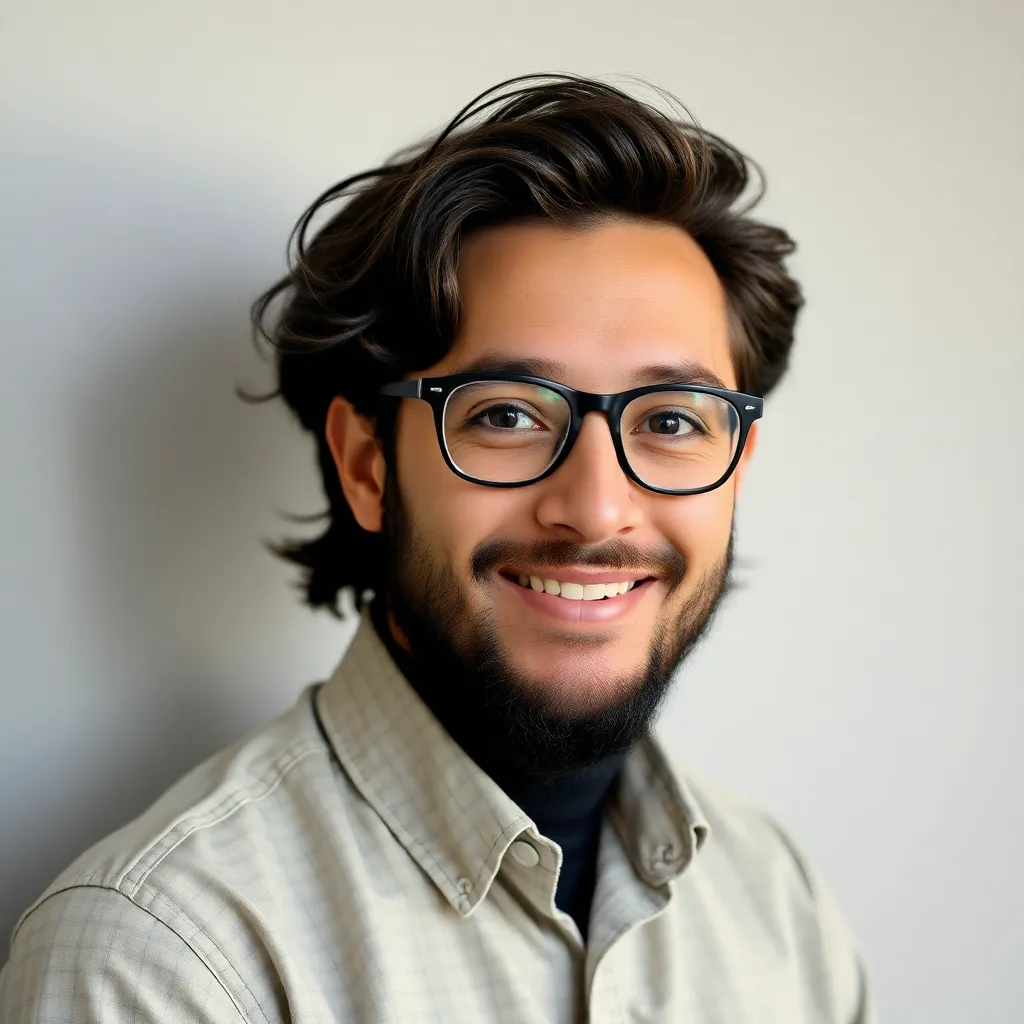
Muz Play
Apr 02, 2025 · 7 min read
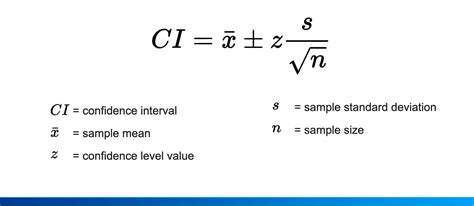
Table of Contents
Confidence Interval Calculator with Two Samples: A Comprehensive Guide
Understanding the difference between populations is crucial in many fields, from medical research comparing treatment efficacy to market analysis assessing customer preferences between products. A powerful statistical tool for making such comparisons is the confidence interval calculator for two samples. This article provides a comprehensive guide to understanding, using, and interpreting these calculations. We'll explore the underlying statistical principles, different scenarios (independent vs. dependent samples), considerations for sample size, and potential pitfalls to avoid.
What is a Confidence Interval?
Before diving into two-sample confidence intervals, let's clarify the fundamental concept of a confidence interval. A confidence interval provides a range of values within which a population parameter (e.g., mean, proportion) is likely to lie with a certain level of confidence. This confidence level is typically expressed as a percentage (e.g., 95%, 99%). A 95% confidence interval, for example, means that if we were to repeat the sampling process many times, 95% of the calculated intervals would contain the true population parameter.
Two-Sample Confidence Intervals: Independent Samples
The most common scenario involves comparing the means of two independent samples. This means that the observations in one sample are not related to the observations in the other sample. For instance, comparing the average test scores of students in two different schools or the average heights of men and women.
Assumptions for Independent Samples:
- Independence: Observations within each sample and between the two samples are independent.
- Normality (or large sample size): The populations from which the samples are drawn are normally distributed, or the sample sizes are sufficiently large (generally, n ≥ 30) for the Central Limit Theorem to apply. The Central Limit Theorem states that the sampling distribution of the mean approaches a normal distribution as the sample size increases, regardless of the population distribution.
- Equal Variances (Optional): While not strictly necessary with large sample sizes, the assumption of equal variances between the two populations simplifies the calculation and leads to a more powerful test. Statistical tests (like Levene's test) can be used to assess the equality of variances.
Formula for the Confidence Interval:
The formula for calculating the confidence interval for the difference between two independent sample means (µ₁ - µ₂) is:
(x̄₁ - x̄₂) ± t * √[(s₁²/n₁) + (s₂²/n₂)]
Where:
- x̄₁ and x̄₂: are the sample means of group 1 and group 2, respectively.
- s₁ and s₂: are the sample standard deviations of group 1 and group 2, respectively.
- n₁ and n₂: are the sample sizes of group 1 and group 2, respectively.
- t: is the critical t-value from the t-distribution with degrees of freedom (df) calculated using a specific formula (detailed below) and corresponding to the chosen confidence level. If the assumption of equal variances is made, a pooled variance is calculated instead.
Degrees of Freedom (df): For independent samples with unequal variances, the calculation of degrees of freedom is more complex and often involves the Welch-Satterthwaite equation. Many statistical software packages automatically handle this calculation. If we assume equal variances (homoscedasticity), the degrees of freedom is simply (n₁ + n₂ - 2).
Example Calculation:
Let's say we have two samples:
- Sample 1: n₁ = 40, x̄₁ = 75, s₁ = 10
- Sample 2: n₂ = 50, x̄₂ = 70, s₂ = 8
We want to calculate a 95% confidence interval for the difference in means. Assuming unequal variances, a statistical software package would provide the appropriate t-value and degrees of freedom. Suppose the t-value obtained is 1.98 (this is a simplified example, and the actual value depends on the degrees of freedom and confidence level).
The confidence interval would be:
(75 - 70) ± 1.98 * √[(10²/40) + (8²/50)] ≈ 5 ± 3.65
Therefore, the 95% confidence interval for the difference in means is approximately (1.35, 8.65). This means we are 95% confident that the true difference in population means lies between 1.35 and 8.65.
Two-Sample Confidence Intervals: Dependent Samples (Paired Samples)
Dependent samples, also known as paired samples, occur when the observations in one sample are related to the observations in the other sample. Common examples include before-and-after measurements on the same individuals (e.g., weight loss before and after a diet program) or comparing matched pairs (e.g., comparing the test scores of twins).
Assumptions for Dependent Samples:
- Paired Data: Observations are paired, creating a dependent relationship between the two samples.
- Normality (or large sample size): The differences between paired observations are normally distributed, or the sample size is sufficiently large.
Formula for the Confidence Interval:
The formula for calculating the confidence interval for the mean difference between paired samples (µd) is:
d̄ ± t * (sd / √n)
Where:
- d̄: is the mean of the differences between paired observations.
- sd: is the standard deviation of the differences between paired observations.
- n: is the number of pairs.
- t: is the critical t-value from the t-distribution with n-1 degrees of freedom and the chosen confidence level.
Example Calculation:
Let's say we have 10 pairs of before-and-after weight measurements. The mean difference (d̄) is 5 pounds, and the standard deviation of the differences (sd) is 2 pounds. For a 95% confidence interval with 9 degrees of freedom, the t-value is approximately 2.26.
The confidence interval would be:
5 ± 2.26 * (2 / √10) ≈ 5 ± 1.43
Therefore, the 95% confidence interval for the mean weight loss is approximately (3.57, 6.43) pounds.
Choosing the Right Test: Independent vs. Dependent Samples
The choice between using a confidence interval for independent or dependent samples hinges on the nature of the data. If the samples are independent, meaning there's no relationship between observations in one sample and the other, you use the independent samples approach. If the samples are paired (e.g., before-and-after measurements on the same subjects), then you must use the dependent samples approach. Choosing the incorrect method leads to inaccurate and misleading results.
Sample Size Considerations
The sample size significantly impacts the width of the confidence interval. Larger sample sizes generally lead to narrower confidence intervals, providing more precise estimates of the population parameter difference. However, obtaining larger samples can be costly and time-consuming. Power analysis can help determine the appropriate sample size needed to achieve a desired level of precision.
Interpreting Confidence Intervals
A confidence interval doesn't tell you the probability that the true population parameter lies within the calculated range. Instead, it tells you the probability that the method used to construct the interval will produce an interval containing the true parameter if repeated many times. A wider interval indicates greater uncertainty, while a narrower interval suggests higher precision. If the confidence interval for the difference between two means includes zero, it suggests that there is not a statistically significant difference between the population means.
Potential Pitfalls and Limitations
- Violation of assumptions: If the assumptions of normality or equal variances (for independent samples) are severely violated, the calculated confidence interval may be unreliable. Transformations of the data or non-parametric methods can sometimes be used to address violations.
- Sampling bias: If the samples are not representative of the populations of interest, the confidence interval will not accurately reflect the true difference between the population parameters.
- Misinterpretation of confidence levels: It's crucial to understand that the confidence level refers to the long-run frequency of intervals containing the true parameter, not the probability that a particular interval contains the true parameter.
Conclusion
Confidence interval calculators for two samples are invaluable tools for comparing population parameters. Understanding the underlying statistical principles, appropriate assumptions, and interpretation is crucial for drawing valid and meaningful conclusions from the data. Remember to choose the correct method (independent or dependent samples) based on the nature of your data and carefully consider the impact of sample size and potential limitations. Using statistical software packages can significantly simplify the calculation and interpretation of two-sample confidence intervals, ensuring accuracy and efficiency in your analysis.
Latest Posts
Latest Posts
-
What Happened To Islam After The Death Of Muhammad
Apr 03, 2025
-
Which Of These Diagrams Is A Convex Mirror
Apr 03, 2025
-
Which State Of Matter Has A Definite Shape
Apr 03, 2025
-
Which Of The Following Is A Single Replacement Reaction
Apr 03, 2025
-
Point Estimate Of The Population Standard Deviation
Apr 03, 2025
Related Post
Thank you for visiting our website which covers about Confidence Interval Calculator With Two Samples . We hope the information provided has been useful to you. Feel free to contact us if you have any questions or need further assistance. See you next time and don't miss to bookmark.