Mean And Standard Deviation Of Sampling Distribution Calculator
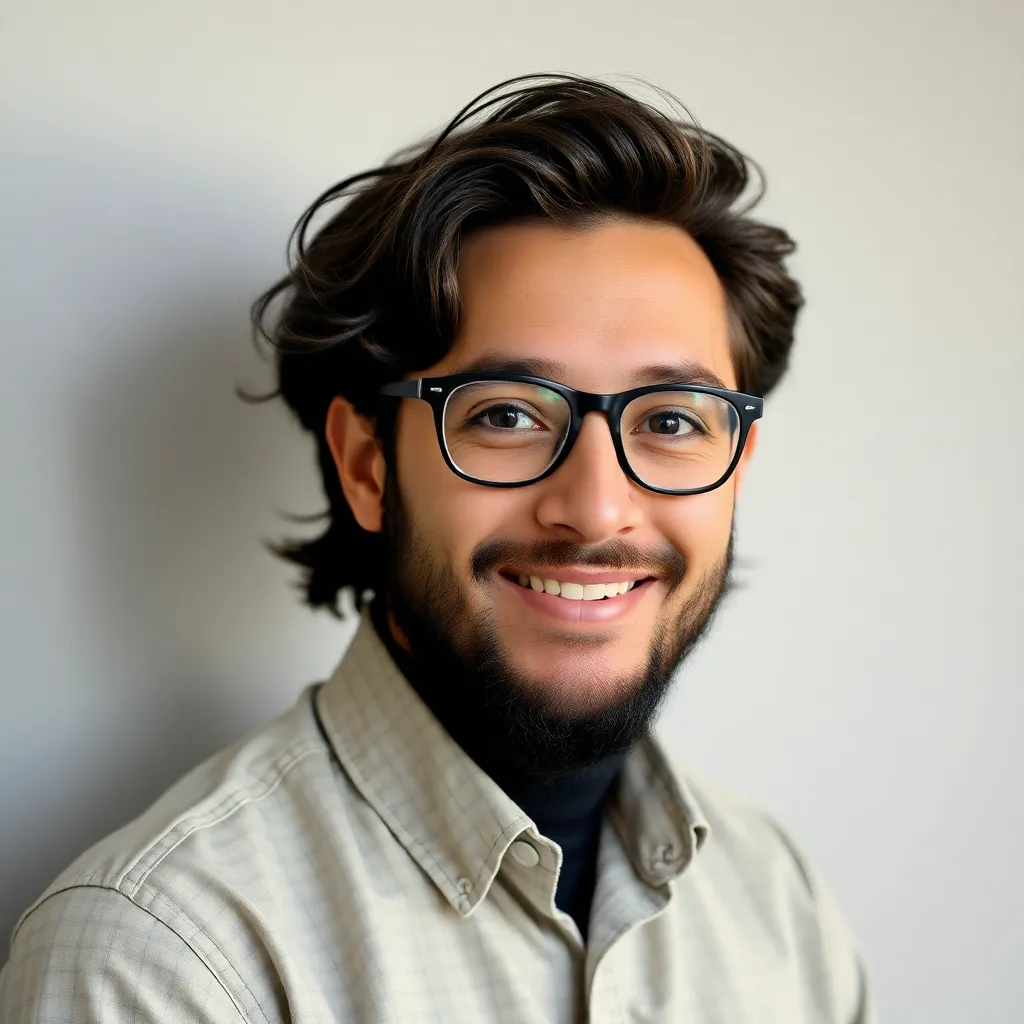
Muz Play
Apr 02, 2025 · 6 min read
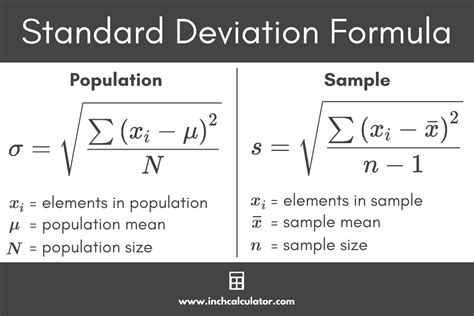
Table of Contents
Mean and Standard Deviation of Sampling Distribution Calculator: A Comprehensive Guide
Understanding the mean and standard deviation of a sampling distribution is crucial for statistical inference. This comprehensive guide delves deep into these concepts, explaining their significance, how to calculate them, and the role of a sampling distribution calculator. We'll explore various scenarios and provide practical examples to solidify your understanding.
What is a Sampling Distribution?
Before diving into the calculations, let's establish a clear understanding of sampling distributions. A sampling distribution is the probability distribution of a statistic (like the sample mean or sample proportion) obtained from a large number of samples drawn from a population. Instead of focusing on individual data points, we're interested in the behavior of the statistic across many samples. This allows us to make inferences about the population based on the characteristics of the sample.
Think of it like this: you have a large jar of marbles, some red, some blue. You don't know the exact proportion of red marbles. You take several small samples of marbles, count the red marbles in each sample, and calculate the proportion of red marbles in each sample. The distribution of these proportions across all your samples forms the sampling distribution of the sample proportion.
The Importance of Mean and Standard Deviation of Sampling Distribution
The mean and standard deviation of a sampling distribution are critical because they describe the center and spread of this distribution, respectively. These values provide valuable insights:
-
Mean of the Sampling Distribution (μ<sub>x̄</sub>): This represents the average of all possible sample means. Importantly, for the mean, it's equal to the population mean (μ). This means that if you take many samples and average their means, you'll get a value close to the true population mean. This is a fundamental principle underlying many statistical tests.
-
Standard Deviation of the Sampling Distribution (σ<sub>x̄</sub>): Also known as the standard error, this measures the variability or spread of the sample means. A smaller standard error indicates that the sample means tend to cluster closely around the population mean, suggesting more precision in our estimations. Conversely, a larger standard error indicates greater variability among the sample means, implying less precision.
Calculating the Mean and Standard Deviation of a Sampling Distribution
The formulas for calculating these values are straightforward:
1. Mean of the Sampling Distribution (μ<sub>x̄</sub>):
μ<sub>x̄</sub> = μ
Where:
- μ<sub>x̄</sub> = Mean of the sampling distribution
- μ = Population mean
2. Standard Deviation of the Sampling Distribution (σ<sub>x̄</sub>):
σ<sub>x̄</sub> = σ / √n
Where:
- σ<sub>x̄</sub> = Standard deviation of the sampling distribution (standard error)
- σ = Population standard deviation
- n = Sample size
Key Observation: The standard error (σ<sub>x̄</sub>) is inversely proportional to the square root of the sample size (n). This means that as the sample size increases, the standard error decreases. Larger samples lead to more precise estimates of the population mean.
The Central Limit Theorem: A Cornerstone of Sampling Distributions
The Central Limit Theorem (CLT) is a cornerstone in statistics. It states that the sampling distribution of the sample mean approaches a normal distribution as the sample size gets larger, regardless of the shape of the population distribution. This is extremely powerful because it allows us to use normal distribution properties to make inferences, even if we don't know the population distribution. This is true even if the original population is not normally distributed, provided the sample size is sufficiently large (generally considered to be n ≥ 30).
Using a Sampling Distribution Calculator
While the formulas are straightforward, manually calculating these values for large datasets can be tedious and prone to errors. This is where a sampling distribution calculator becomes invaluable. These calculators streamline the process, allowing you to input your data (sample mean, sample standard deviation, and sample size) and instantly receive the mean and standard deviation of the sampling distribution.
Features of a typical sampling distribution calculator might include:
- Input fields for sample statistics: You'll enter the sample mean, sample standard deviation, and sample size.
- Selection of the statistic: You might be able to calculate the sampling distribution for the mean, proportion, or other statistics.
- Output of the mean and standard deviation: The calculator will provide the calculated mean (μ<sub>x̄</sub>) and standard deviation (σ<sub>x̄</sub>) of the sampling distribution.
- Optional visualization: Some calculators might display the sampling distribution graphically, providing a visual representation of the data.
Practical Examples
Let's illustrate the concepts with examples:
Example 1: Exam Scores
Suppose the average score on a standardized exam is 75 (μ = 75) with a standard deviation of 10 (σ = 10). We take a sample of 100 students (n = 100).
- Mean of the sampling distribution (μ<sub>x̄</sub>): μ<sub>x̄</sub> = μ = 75
- Standard deviation of the sampling distribution (σ<sub>x̄</sub>): σ<sub>x̄</sub> = σ / √n = 10 / √100 = 1
The mean of the sampling distribution of the sample mean is 75, and the standard error is 1. This indicates that the sample means will cluster closely around 75.
Example 2: Website Visits
Let's say the average number of daily website visits is 500 (μ = 500) with a standard deviation of 50 (σ = 50). We collect data from 25 days (n = 25).
- Mean of the sampling distribution (μ<sub>x̄</sub>): μ<sub>x̄</sub> = μ = 500
- Standard deviation of the sampling distribution (σ<sub>x̄</sub>): σ<sub>x̄</sub> = σ / √n = 50 / √25 = 10
Here, the mean of the sampling distribution remains 500, but the standard error is 10. This shows that the sample means will exhibit more variability compared to the previous example due to the smaller sample size.
Applications of Sampling Distributions
Understanding sampling distributions is fundamental to many statistical applications:
- Confidence Intervals: Constructing confidence intervals for population parameters relies heavily on the concept of sampling distributions. The standard error is crucial in determining the width of the confidence interval.
- Hypothesis Testing: Many statistical hypothesis tests involve comparing sample statistics to a hypothesized population parameter. The sampling distribution helps determine the probability of observing the sample statistic if the null hypothesis were true.
- A/B Testing: In A/B testing (comparing two versions of a website or advertisement), sampling distributions are used to assess whether the observed differences between the groups are statistically significant or due to chance.
Conclusion
The mean and standard deviation of a sampling distribution are critical concepts in statistical inference. They allow us to make inferences about population parameters based on sample data. While the calculations are straightforward, a sampling distribution calculator can significantly simplify the process, especially for larger datasets. Understanding these concepts, coupled with the use of appropriate tools, empowers you to conduct more accurate and reliable statistical analyses. Remember the importance of the Central Limit Theorem in ensuring the applicability of these calculations across diverse data scenarios. Mastering these concepts will significantly enhance your understanding and application of statistical methods in various fields.
Latest Posts
Latest Posts
-
Bases Produce Which Ions In Aqueous Solution
Apr 03, 2025
-
An Atom That Has Lost Or Gained An Electron
Apr 03, 2025
-
What Is The Formula Of A Hydronium Ion
Apr 03, 2025
-
What Is Closed And Open System
Apr 03, 2025
-
What Does It Mean When We Say Water Is Adhesive
Apr 03, 2025
Related Post
Thank you for visiting our website which covers about Mean And Standard Deviation Of Sampling Distribution Calculator . We hope the information provided has been useful to you. Feel free to contact us if you have any questions or need further assistance. See you next time and don't miss to bookmark.